Table of Contents
- Introduction
- Editor’s Choice
- Global Neuromorphic Computing Market Overview
- Capacity of Some Recent Neuromorphic Systems
- Neural Networks Digital Hardware Implementations
- Recent Neuromorphic Device Implementations
- Materials Systems for Neuromorphic Applications
- Development of Neuromorphic Chips
- Recent Developments in Neuromorphic Computing
Introduction
According to Neuromorphic Computing Statistics, Neuromorphic computing is inspired by the structure and operations of the human brain, aiming to develop computational systems that can efficiently tackle complex tasks while consuming minimal power.
It entails replicating the brain’s neural networks through technologies like spiking neural networks (SNNs) and memristors, which imitate synaptic connections and signaling processes.
With applications spanning edge computing, pattern recognition, and robotics, neuromorphic computing offers advantages such as energy efficiency and flexibility.
Despite encountering hurdles like scalability and hardware limitations, it shows significant potential for creating intelligent systems inspired by the brain’s extraordinary capabilities.
Editor’s Choice
- The global neuromorphic computing market accounted for a revenue of 5.1 billion USD in 2023.
- By 2032, the market had expanded significantly, reaching a total revenue of 29.2 billion USD, with hardware accounting for 19.0 billion USD, software for 6.25 billion USD, and services for 3.97 billion USD.
- Consumer electronics dominate the landscape with a substantial market share of 48%, reflecting the widespread integration of neuromorphic technologies in devices and gadgets to enhance user experience and functionality.
- DYNAPs offers 256 neurons per core and 16K synapses per core, while BrainScaleS provides 512 neurons per core and 128K synapses per core, with four chips per board.
- Among slice architectures, devices like Microdevices MD-1220a, NeuraLogix NLX-420a, and Philips Lneuro-1 offer feedforward and machine-learning capabilities with varying precision, neurons, synapses, and processing speeds.
- In 2016, researchers at MIT introduced a novel chip tailored for neural network implementation. With efficiency levels tenfold greater than a mobile GPU, the chip has the potential to empower mobile devices to execute AI algorithms locally, eliminating the need to transmit data to the cloud for processing.
- In December 2022, Intel introduced its AI neuromorphic technology, which offers processing speeds 1,000 times faster than conventional CPUs and GPUs, with notably lower energy consumption.
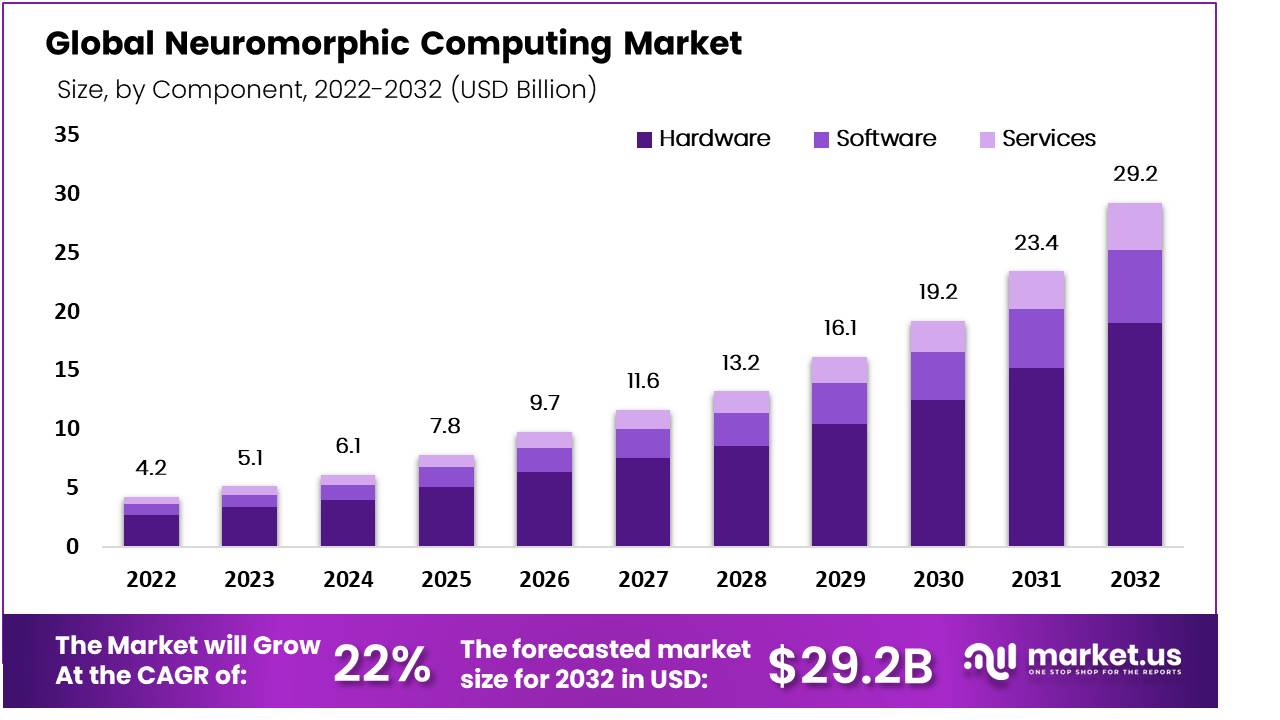
Global Neuromorphic Computing Market Overview
Global Neuromorphic Computing Market Size
- The global neuromorphic computing market has consistently grown over the past decade at a CAGR of 22%, with revenues steadily increasing from 4.2 billion USD in 2022 to an estimated 29.2 billion USD by 2032.
- Notably, the sector experienced notable year-on-year gains, with revenues climbing to 5.1 billion USD in 2023, followed by subsequent increases to 6.1 billion USD in 2024, 7.8 billion USD in 2025, and 9.7 billion USD in 2026.
- The upward trend continued in subsequent years, with revenues surging to 13.2 billion USD in 2028, 16.1 billion USD in 2029, and 19.2 billion USD in 2030.
- As the decade unfolded, the market witnessed robust expansion, culminating in revenues exceeding 23.4 billion USD in 2031 and a substantial 29.2 billion USD by 2032.
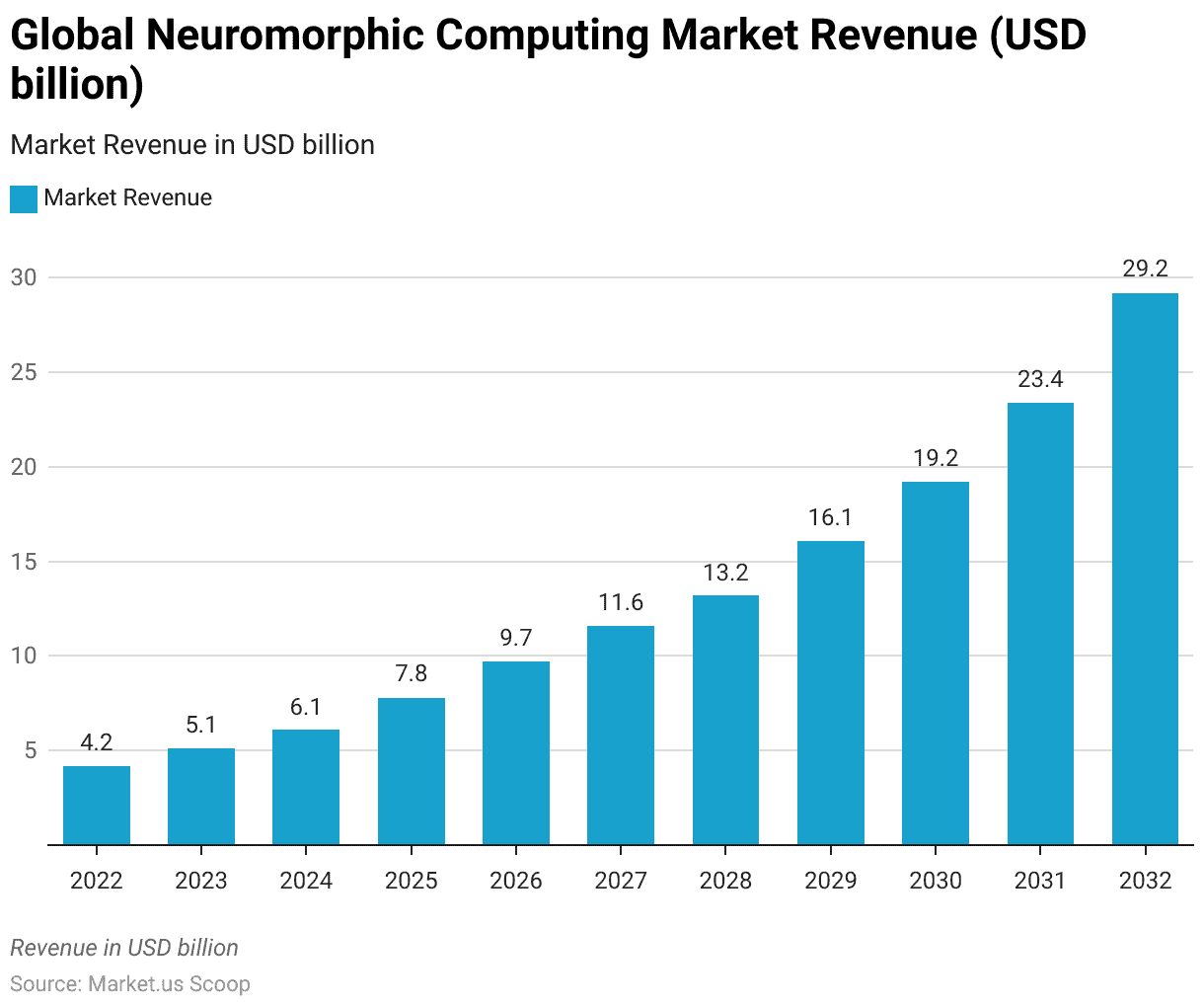
Neuromorphic Computing Market Size – By Component
- The global neuromorphic computing market exhibits a multifaceted landscape delineated by its various components – hardware, software, and services.
- From 2022 to 2032, the market has grown substantially across all segments for a decade. In 2022, the total market revenue stood at 4.2 billion USD, with hardware contributing 2.7 billion USD, software 0.90 billion USD, and services 0.57 billion USD.
- By 2032, the market had expanded significantly, reaching a total revenue of 29.2 billion USD, with hardware accounting for 19.0 billion USD, software for 6.25 billion USD, and services for 3.97 billion USD.
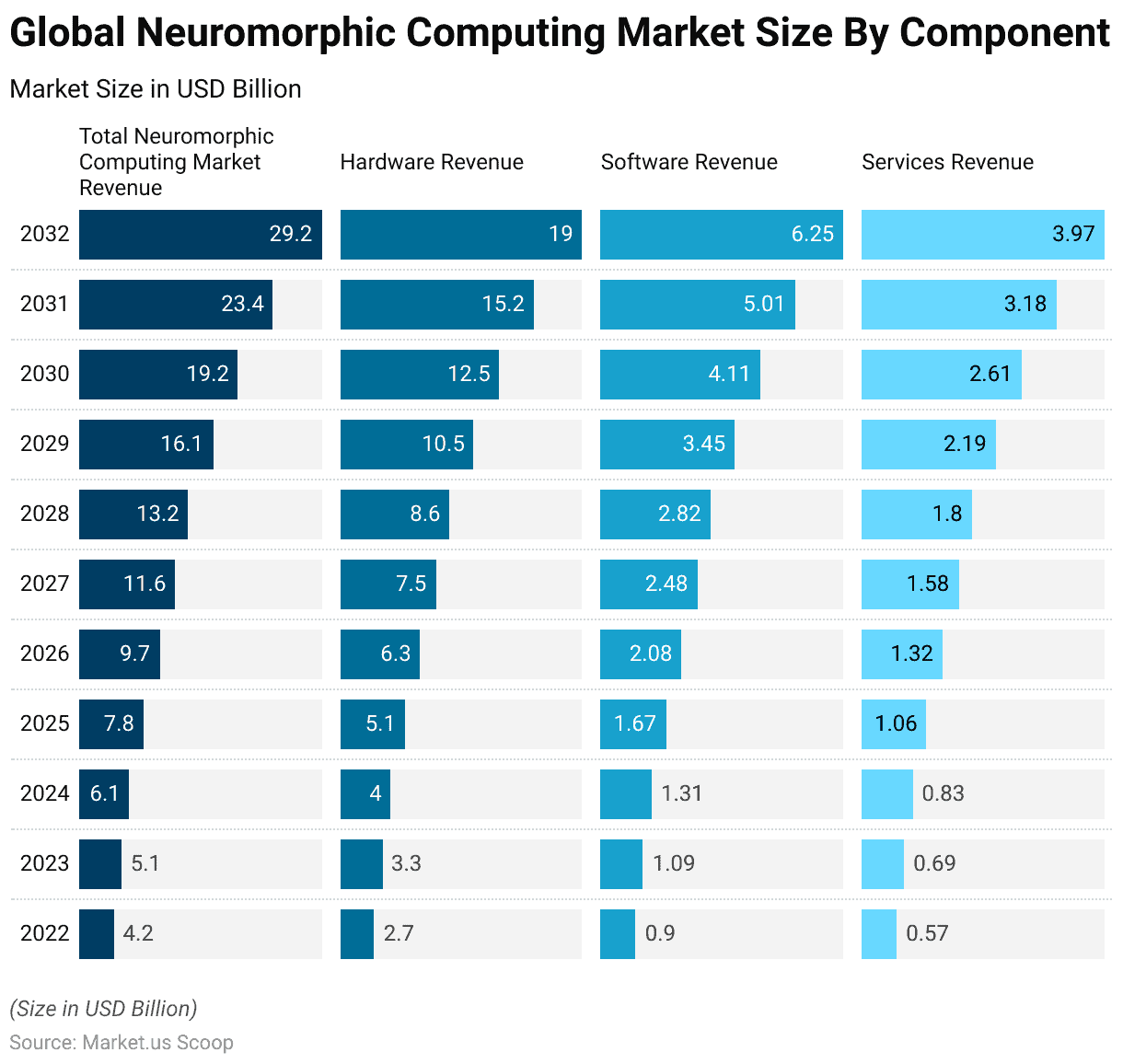
Global Neuromorphic Computing Market Share – By End-Use
- The global market for neuromorphic computing is divided among various end-use sectors, with each industry claiming a distinct market share.
- Consumer electronics dominate the landscape with a substantial market share of 48%, reflecting the widespread integration of neuromorphic technologies in devices and gadgets to enhance user experience and functionality.
- Following closely behind is the automotive industry, commanding a significant share of 27%, as manufacturers increasingly incorporate neuromorphic computing for autonomous driving, vehicle safety, and infotainment systems.
- Aerospace & defense sectors hold 11% of the market share, leveraging neuromorphic computing for applications such as advanced surveillance, autonomous drones, and military simulations.
- Healthcare follows with an 8% share, where neuromorphic computing finds utility in medical imaging, diagnostics, and personalized treatment solutions.
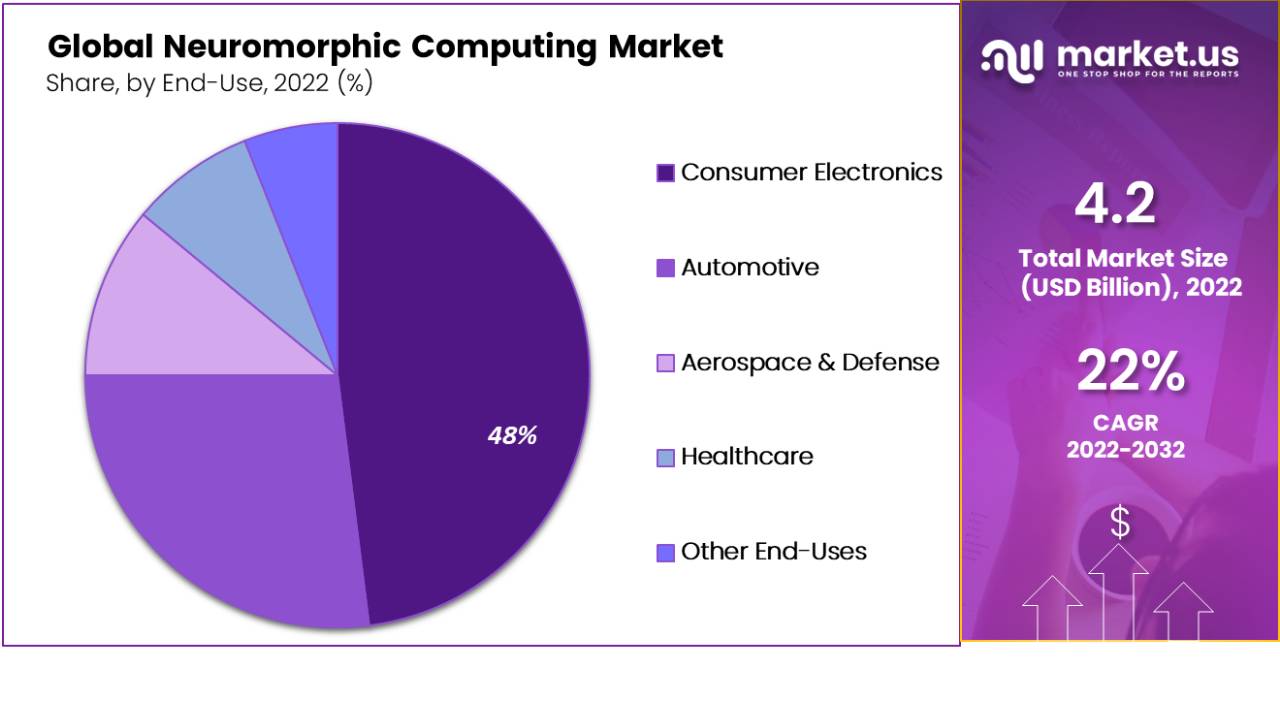
Capacity of Some Recent Neuromorphic Systems
- Recent neuromorphic systems such as ODIN, µBrain, DYNAPs, BrainScaleS, SpiNNaker, Neurogrid, Loihi, and TrueNorth exhibit varying capacities in terms of neurons, synapses, cores per chip, and chips per board.
- For instance, ODIN and µBrain feature 256 neurons per core and 64K synapses per core, with a single chip per board configuration.
- DYNAPs offers 256 neurons per core and 16K synapses per core, while BrainScaleS provides 512 neurons per core and 128K synapses per core, with four chips per board.
- In contrast, SpiNNaker has 36K neurons per core and 2.8M synapses per core, distributed across 352 chips per board.
- Neurogrid boasts 65K neurons per core and 8M synapses per core, spread across 56 chips per board.
Neural Networks Digital Hardware Implementations
- Various neural networks’ digital hardware implementations exhibit diverse architectures, learning capabilities, precision levels, and processing speeds.
- Among slice architectures, devices like Microdevices MD-1220a, NeuraLogix NLX-420a, and Philips Lneuro-1 offer feedforward and machine-learning capabilities with varying precision, neurons, synapses, and processing speeds.
- Similarly, SIMD architectures like Inova N64000a and Hecht-Nielson HNC 100-NAPb employ parallel processing elements for efficient computation.
- Systolic arrays, represented by Siemens MA-16, leverage matrix operations for neural network tasks. Radial basis function (RBF) architectures, such as Nestors/Intel NI1000c and IBM ZISC036, specialize in pattern recognition with different precision levels and processing speeds.
Recent Neuromorphic Device Implementations
- Several recent neuromorphic device implementations, including Darwin6, DANNA1, TrueNorth2, Neurogrid3, BrainScaleS4, and SpiNNaker5, offer various programmable structures and component complexities tailored to different application needs.
- Darwin6 features a programmable structure with high component complexity, accommodating neurons and synapses exceeding five in number.
- It incorporates on-chip learning capabilities and utilizes novel materials while being fabricated with existing CMOS processes.
- DANNA1, on the other hand, maintains a more straightforward structure with neurons and synapses limited to two, and it supports on-chip learning. It can be implemented using FPGA or ASIC technologies.
- TrueNorth2 and Neurogrid3 both employ fixed structures for synapses (on/off), with TrueNorth having a higher neuron-to-synapse ratio than Neurogrid.
Materials Systems for Neuromorphic Applications
- Materials systems for neuromorphic applications encompass diverse mechanisms and characteristics tailored to address specific requirements.
- Oxides like HfOX/TiOX/HfOX/TiOX demonstrate fast write speeds of 10s of nanoseconds, with excellent data retention exceeding 15 minutes at temperatures ranging from 20 to 85 degrees Celsius.
- VO2 offers rapid write speeds of 1 second and good data retention at 70 degrees Celsius, albeit with concerns regarding memory duration and limited potentiation.
- Nb2O5/Pt exhibits moderate write speeds in the order of 100s of nanoseconds and exceptional data retention exceeding 500 years at room temperature, making it suitable for long-term storage applications.
- WOX provides relatively slower write speeds in the range of 100s to microseconds but offers reasonable data retention of over 3 hours at room temperature.
- Other materials systems like Nb-doped-a-STO, Pt/TiO2/Pt, and phase change materials such as GeSbTe and carbon nanotubes present varying write speed, data retention, and temperature range characteristics.
Development of Neuromorphic Chips
- In 2016, researchers at MIT introduced a novel chip tailored for neural network implementation. With efficiency levels tenfold greater than a mobile GPU, the chip has the potential to empower mobile devices to execute AI algorithms locally, eliminating the need to transmit data to the cloud for processing.
- BM Research has been steadily advancing the development of the TrueNorth chip.
- Qualcomm has been working on the Zeroth NPU, which can recognize gestures, expressions, and faces and intelligently sense surroundings.
- Numenta, led by Jeff Hawkins since its establishment in 2005, has been making progress in emulating cortical columns found in the brain’s neocortex. They have released products based on the NuPIC architecture for analyzing streaming data.
Recent Developments in Neuromorphic Computing
- In December 2022, Intel introduced its AI neuromorphic technology, which offers processing speeds 1,000 times faster than conventional CPUs and GPUs, with notably lower energy consumption.
- Its exceptional energy efficiency suits smart homes, industrial equipment, and cybersecurity applications.
- In June 2022, researchers at China’s Tsinghua University Center for Brain-Inspired Computing Research developed a neuromorphic chip for AI applications with lower power consumption than a standard NVIDIA chip.
- The Tianjicat chip used just over half the power of an equivalent NVIDIA-based robot. Additionally, the neuromorphic chip-based robot exhibited significantly reduced latency, 79 times less than the NVIDIA-based system, enabling quicker decision-making.
- In November 2022, Oppo revealed its partnership with Qualcomm Technologies to enhance ray-tracing graphics on mobile devices.
Discuss your needs with our analyst
Please share your requirements with more details so our analyst can check if they can solve your problem(s)
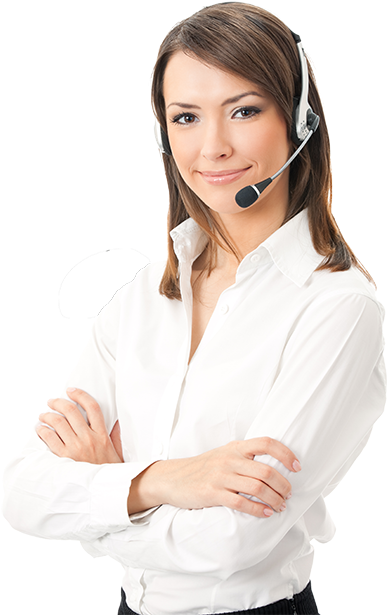