Table of Contents
- Introduction
- Editor’s Choice
- AI Training Dataset Market Overview
- Global AI Usage for IT and Telecommunications
- Technical Specifications of AI Training Dataset Statistics
- Scale of Datasets Utilized in AI Training Across Various Applications Statistics
- Data Pre-Processing and Cleaning Among Organizations
- Data Sources Used by the Public Sector for Training AI Models
- Popular AI Training Dataset Programs Statistics
- Level of Integration of Organizations’ Analytics Tools with Data Sources and AI Platforms Used
- Sophistication of Organizations’ Analytics Tools in Handling Complex AI Dataset Training Statistics
- Adaptability and Scalability of Organizations’ Analytics Tools in Catering to Evolving Needs of AI Projects
- Technical Advancements in AI Training Dataset Statistics
- Recent Developments
- Conclusion
- FAQs
Introduction
AI Training Dataset Statistics: AI training datasets are essential for developing machine learning models. Containing data that helps the model learn to recognize patterns and make predictions.
These datasets can be categorized into supervised learning, where data includes input-output pairs; and unsupervised learning.
Where only inputs are provided; and reinforcement learning, which involves sequences of actions and rewards.
Key steps in data preparation include cleaning, normalization, and splitting into training, validation, and test sets.
Data can come from real-world sources, be synthetically generated, or be annotated. Challenges include managing biases and ensuring data quality.
Best practices involve using diverse data, data augmentation, and addressing ethical concerns to create effective and fair AI models.
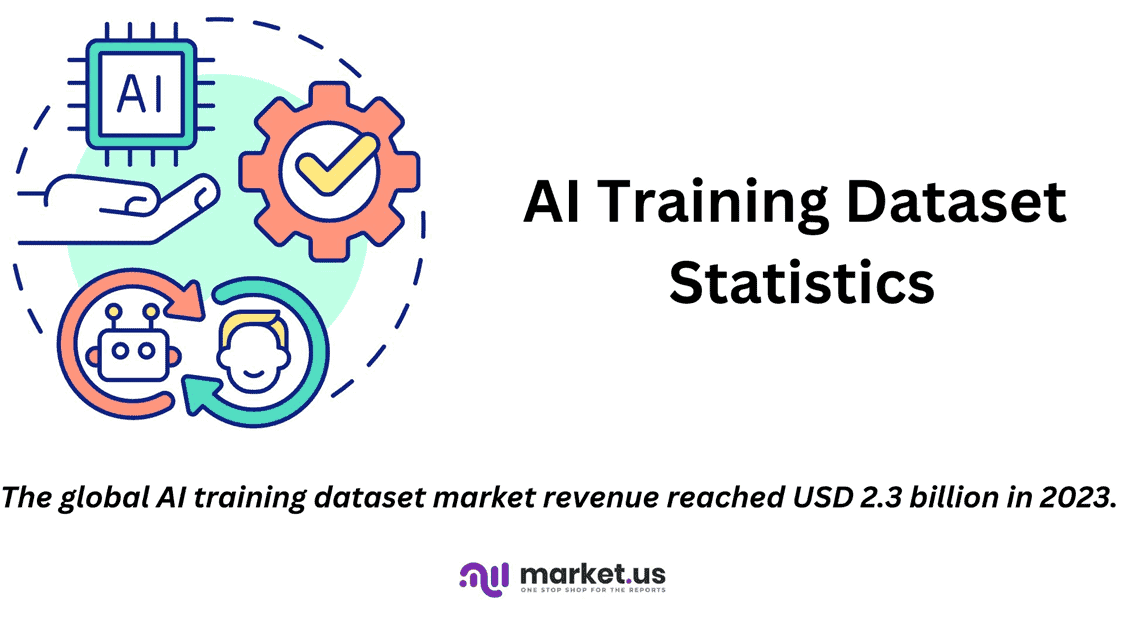
Editor’s Choice
- The global AI training dataset market revenue reached USD 2.3 billion in 2023.
- By 2032, the market is expected to culminate in a total revenue of USD 11.7 billion, with text datasets contributing USD 4.42 billion. Image and video datasets are USD 3.79 billion, and audio datasets are USD 3.49 billion.
- Various end-use industries significantly influence the global AI training dataset market, each contributing a distinct share to the overall market. The IT and telecommunications sector holds the largest share at 31%. Reflecting its critical role in driving technological advancements and data-centric innovations.
- As of 2020, the IT and telecommunications industry worldwide employed artificial intelligence (AI) across various use cases, each reflecting a significant share of respondents. Cybersecurity emerged as the leading application. With 52% of respondents indicating its usage to enhance security measures against cyber threats.
- For facial recognition technologies, training data encompasses over 450,000 facial images.
- As of October 2022, the public sector in South Korea utilized a variety of data sources for training artificial intelligence (AI) models. The predominant source was in-house data, excluding customer data, which 56.3% of respondents used.
- Google’s Open Images dataset offers over 9 million annotated images suitable for various computer vision tasks.
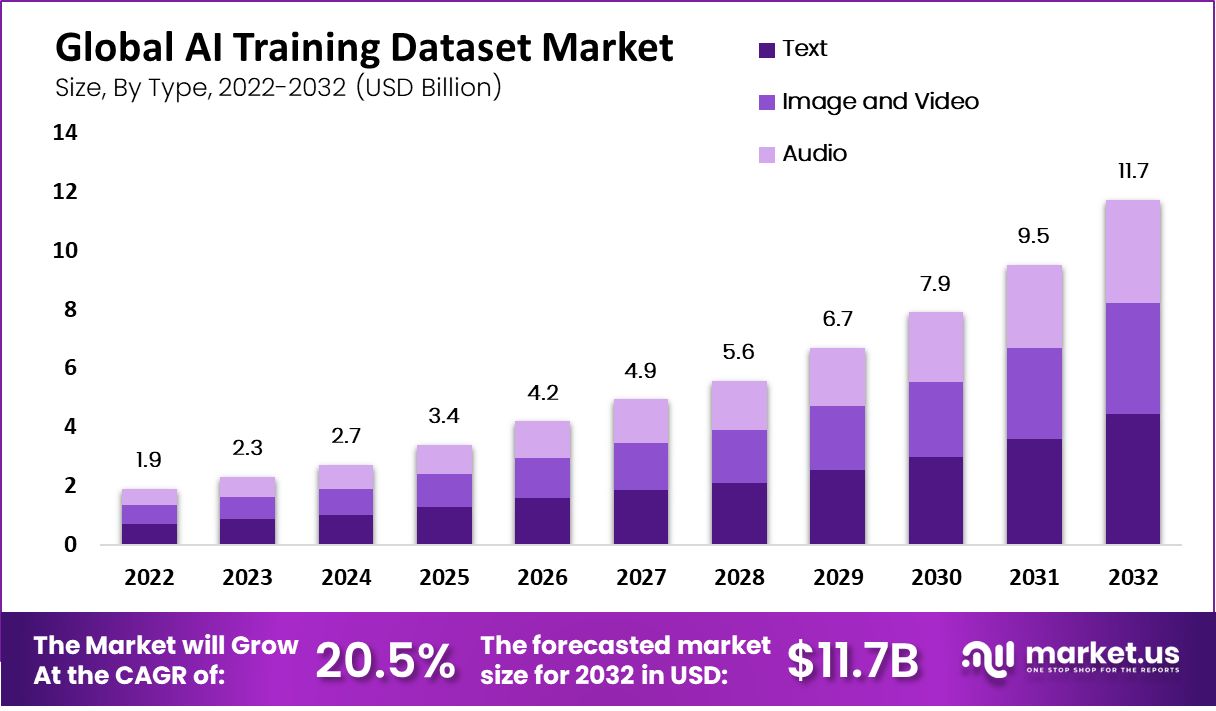
AI Training Dataset Market Overview
Global AI Training Dataset Market Size Statistics
- The global AI training dataset market has demonstrated significant growth over the years at a CAGR of 20.5%. With revenue increasing from USD 1.9 billion in 2022 to USD 2.3 billion in 2023.
- This upward trend is projected to continue, reaching USD 2.7 billion in 2024 and further accelerating to USD 3.4 billion by 2025.
- The market is expected to experience substantial expansion. With revenues climbing to USD 4.2 billion in 2026, USD 4.9 billion in 2027, and USD 5.6 billion in 2028.
- By 2029, the market is anticipated to grow to USD 6.7 billion. Followed by a significant rise to USD 7.9 billion in 2030.
- The upward trajectory is projected to persist, with revenues reaching USD 9.5 billion in 2031 and culminating in an impressive USD 11.7 billion by 2032.
- This consistent growth highlights the increasing demand and investment in AI training datasets across various industries.
(Source: market.us)
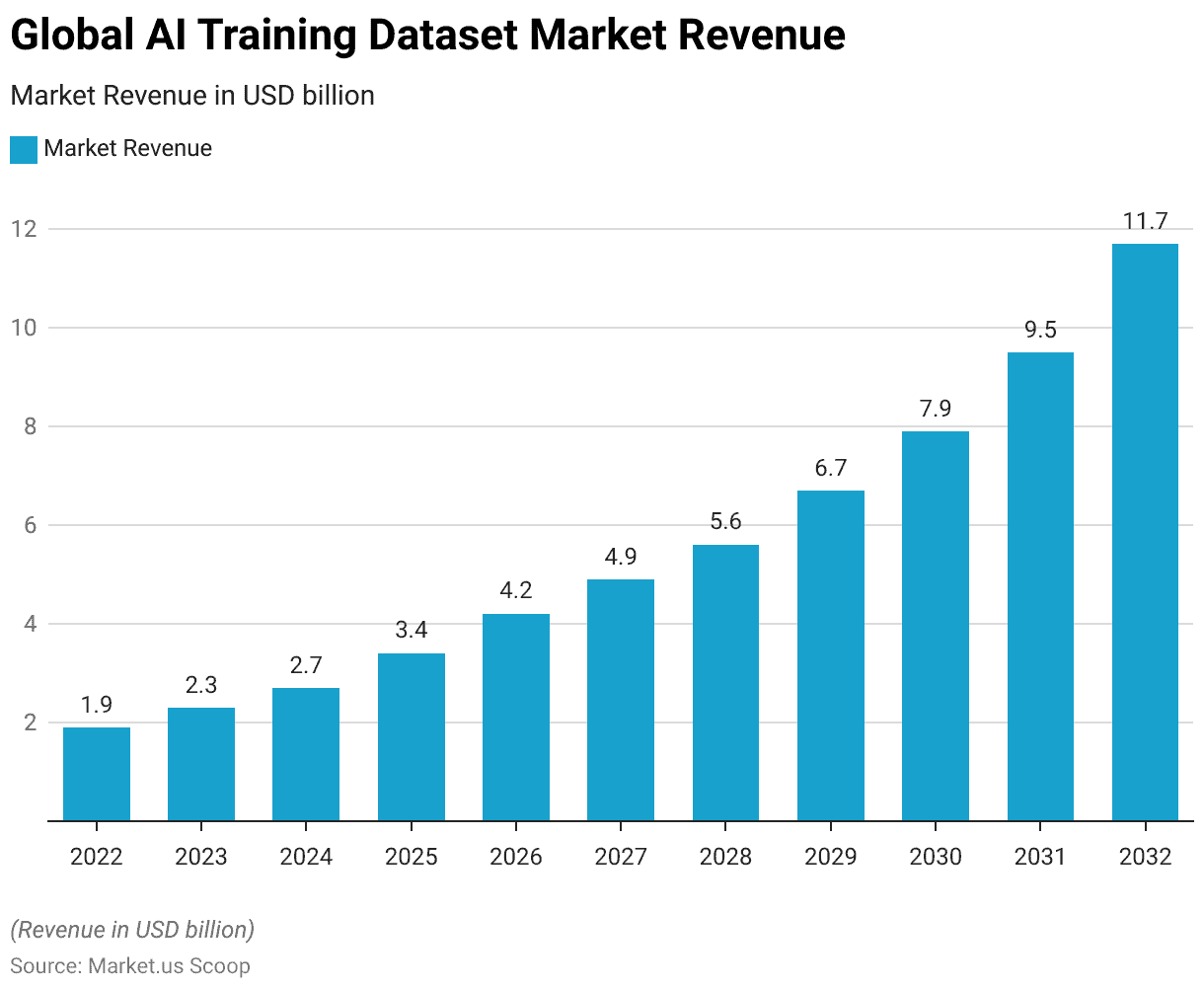
Global AI Training Dataset Market Size – By Type Statistics
2022-2027
- The global AI training dataset market, segmented by type, has exhibited robust growth from 2022 to 2027.
- In 2022, the total market revenue was USD 1.9 billion, with text datasets contributing USD 0.72 billion. Image and video datasets account for USD 0.62 billion, and audio datasets generate USD 0.57 billion.
- By 2023, the total revenue increased to USD 2.3 billion, with text, image, video, and audio datasets earning USD 0.87 billion, USD 0.75 billion, and USD 0.69 billion, respectively.
- This growth trend continued, reaching USD 2.7 billion in 2024, with text datasets at USD 1.02 billion. Image and video at USD 0.87 billion, and audio at USD 0.80 billion.
- The market’s expansion persisted into 2025, achieving USD 3.4 billion in total revenue. Where text datasets contributed USD 1.29 billion, image and video USD 1.10 billion, and audio USD 1.01 billion.
- By 2026, the total revenue rose to USD 4.2 billion, with text, image, video, and audio datasets generating USD 1.59 billion, USD 1.36 billion, and USD 1.25 billion, respectively.
- In 2027, the market reached USD 4.9 billion, with contributions of USD 1.85 billion from text datasets. USD 1.59 billion from image and video datasets, and USD 1.46 billion from audio datasets.
2028-2032
- The growth continued through 2028, with the total market size reaching USD 5.6 billion, and text datasets contributing USD 2.12 billion. Image and video datasets are USD 1.81 billion, and audio datasets are USD 1.67 billion.
- By 2029, the market size grew to USD 6.7 billion, with text datasets at USD 2.53 billion, image and video at USD 2.17 billion, and audio at USD 2.00 billion.
- In 2030, the total market revenue was USD 7.9 billion, with text, image, video, and audio datasets contributing USD 2.99 billion, USD 2.56 billion, and USD 2.35 billion, respectively.
- The upward trajectory continued into 2031, reaching USD 9.5 billion in total revenue, with text datasets at USD 3.59 billion, image and video datasets at USD 3.08 billion, and audio datasets at USD 2.83 billion.
- By 2032, the market culminated in a total revenue of USD 11.7 billion, with text datasets contributing USD 4.42 billion, image and video datasets USD 3.79 billion, and audio datasets USD 3.49 billion.
- This consistent growth underscores the increasing demand for diverse AI training datasets across various applications and industries.
(Source: market.us)
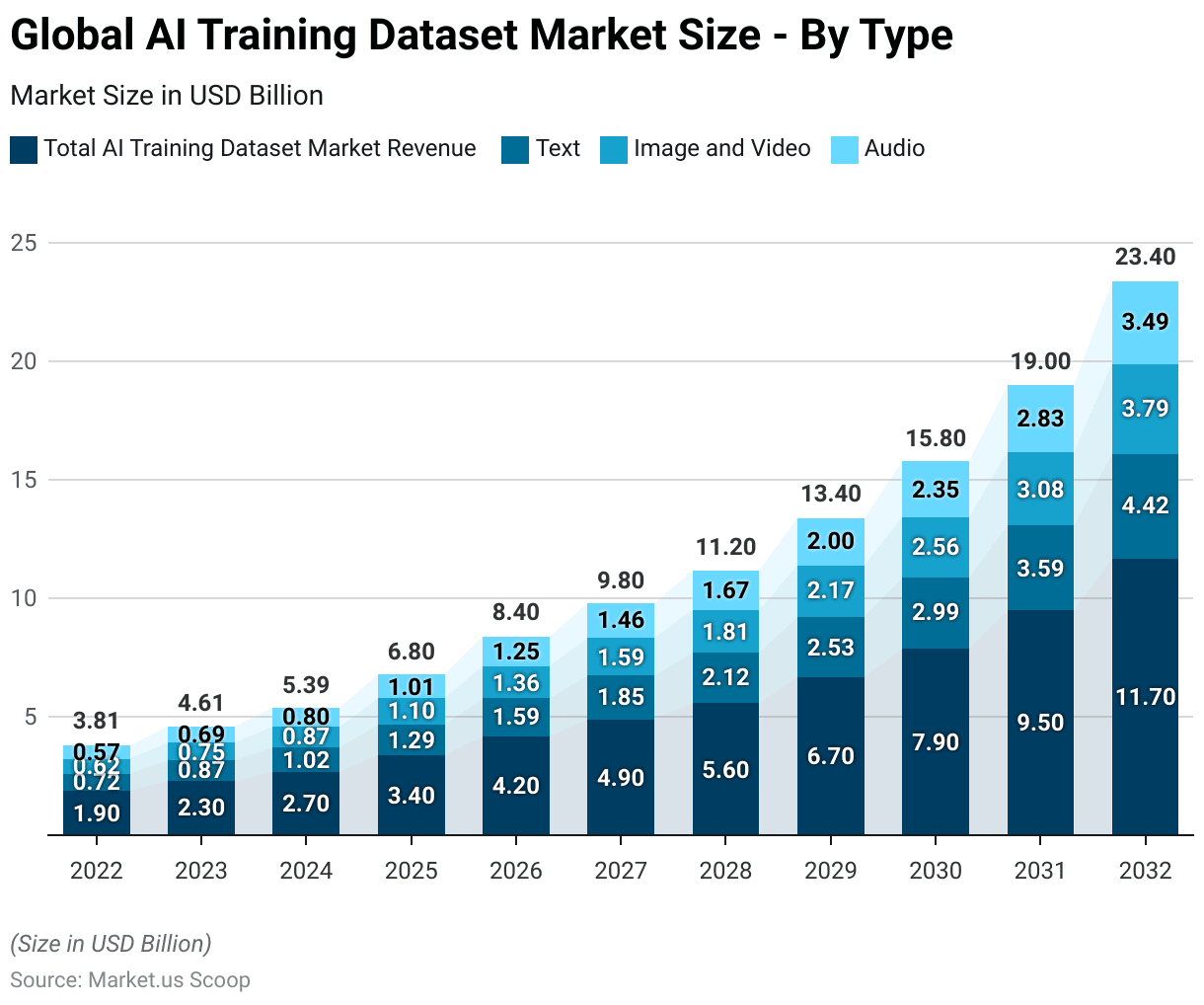
Global AI Training Dataset Market Share – By End-Use Industry Statistics
- Various end-use industries significantly influence the global AI training dataset market, each contributing a distinct share to the overall market.
- The IT and telecommunications sector holds the largest share at 31%, reflecting its critical role in driving technological advancements and data-centric innovations.
- The automotive industry follows with a 22% share, underscoring the growing adoption of AI for autonomous driving and smart vehicle technologies.
- The healthcare sector, representing 18% of the market, demonstrates the increasing integration of AI in medical diagnostics, patient care, and healthcare management.
- The retail and e-commerce industry accounts for 7%, highlighting the use of AI for personalized shopping experiences and supply chain optimization.
- The government sector, with a 12% share, indicates the implementation of AI for public administration, security, and infrastructure development.
- The banking, financial services, and insurance (BFSI) sector contributes 6%, showcasing AI’s role in fraud detection, customer service, and financial analytics.
- Other end-use industries, collectively holding 4%, further illustrate the diverse applications and growing penetration of AI training datasets across various sectors.
(Source: market.us)
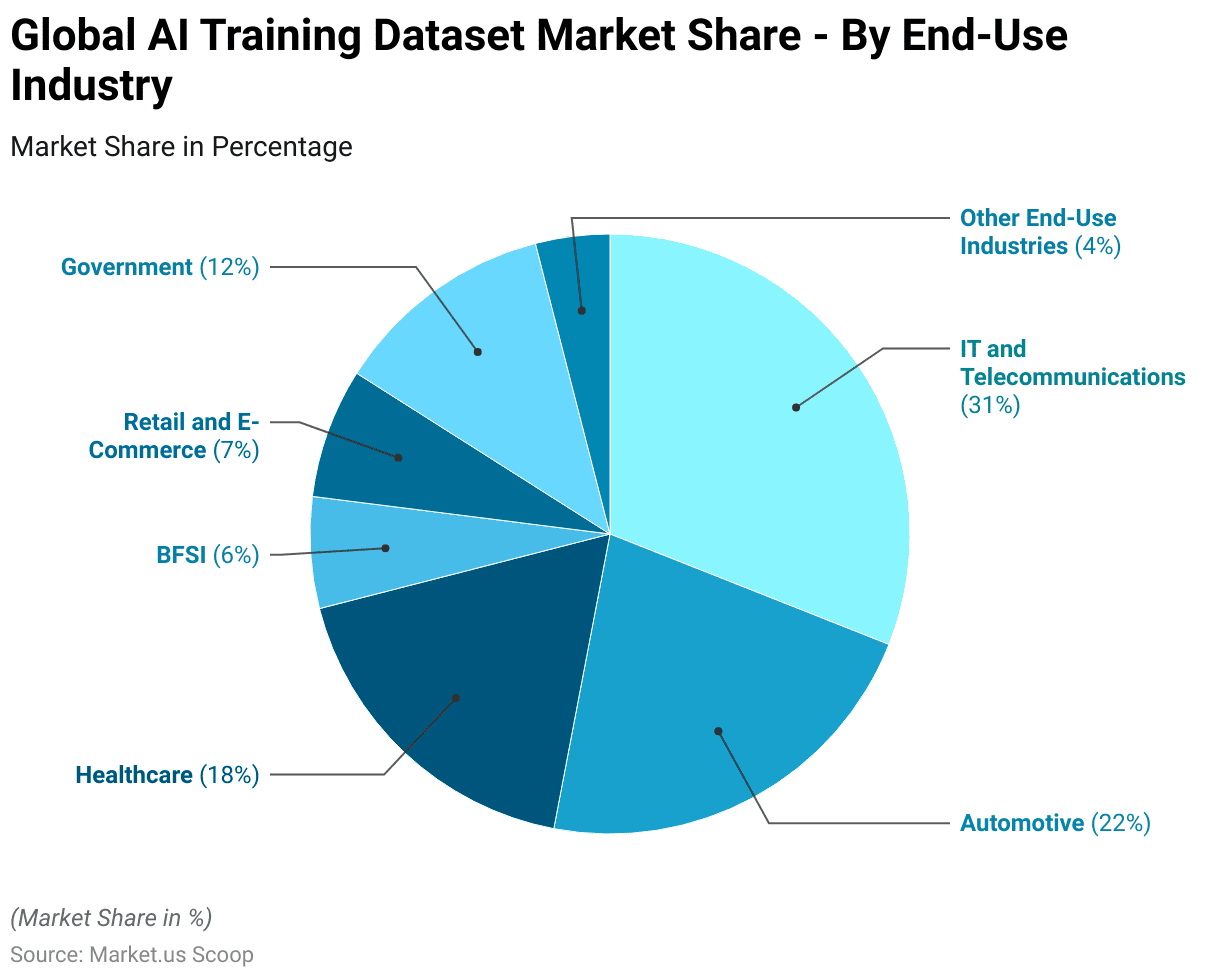
Global AI Usage for IT and Telecommunications
- As of 2020, the IT and telecommunications industry worldwide employed artificial intelligence (AI) across various use cases, each reflecting a significant share of respondents.
- Cybersecurity emerged as the leading application, with 52% of respondents indicating its usage to enhance security measures against cyber threats.
- Customer care was the second most prevalent use case, utilized by 48% of respondents to improve customer service and support through AI-driven solutions.
- Both fraud detection and quality control were reported by 38% of respondents. Showcasing AI’s role in identifying fraudulent activities and ensuring product and service quality.
- Personalization of products and services was cited by 31% of respondents. Highlighting AI’s capability to tailor offerings to individual customer preferences.
- Additionally, 30% of respondents reported using AI for monitoring and diagnostics. Demonstrating its importance in maintaining and optimizing IT and telecommunication systems.
- This diverse range of AI applications underscores the technology’s integral role in enhancing efficiency, security, and customer satisfaction within the industry.
(Source: Statista)
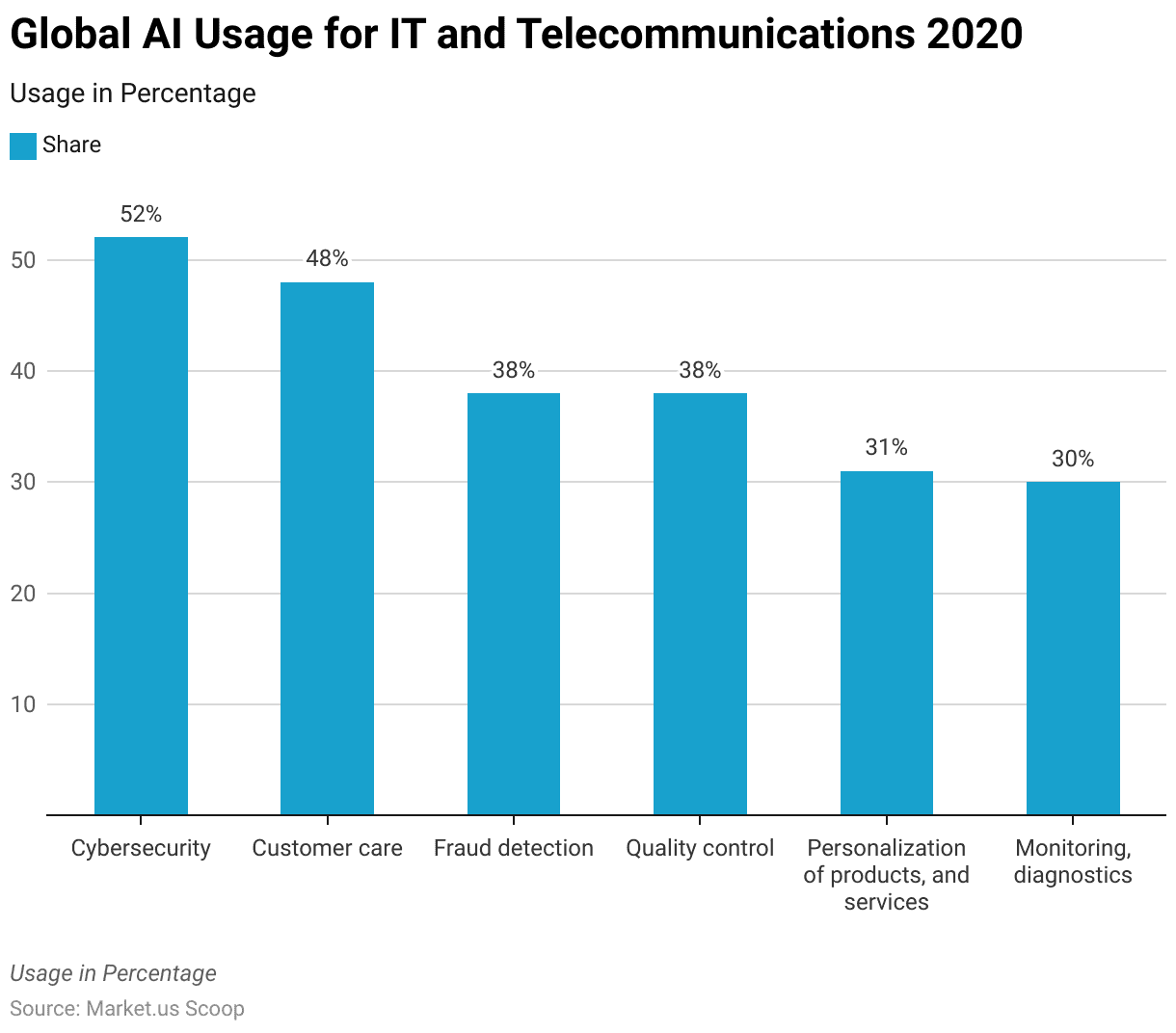
Technical Specifications of AI Training Dataset Statistics
- The technical specifications of AI training datasets encompass several key aspects crucial for developing high-performing AI models.
- The data storage requirements for AI datasets are significant. Often involving terabytes or petabytes of structured and unstructured data such as text, images, audio, and video.
- Processing capacity must be robust, leveraging high-performance computing (HPC) systems and GPU clusters to handle extensive computations and parallel processing necessary for training complex models.
- Network parameters, including bandwidth and latency, play a vital role, especially when datasets are distributed across multiple locations, requiring efficient data transfer protocols.
- Data volume varies widely; for instance, facial recognition systems may require over 450,000 images, while chatbot training could involve millions of text samples.
- Testing scales often necessitate large and diverse datasets to ensure model robustness and generalization across different scenarios.
- Quality control measures, such as data annotation and bias elimination. They are critical for maintaining dataset integrity and ensuring accurate model training.
(Sources: SHAIP, Appen)
Scale of Datasets Utilized in AI Training Across Various Applications Statistics
- The datasets employed for training artificial intelligence systems vary widely in scale depending on the application.
- For facial recognition technologies, training data encompasses over 450,000 facial images.
- Image annotation efforts involve more than 185,000 images, with close to 650,000 objects annotated within these images.
- Sentiment analysis on platforms like Facebook utilizes a dataset comprising over 9,000 comments and 62,000 posts.
- Chatbot systems are trained on a substantial dataset of approximately 200,000 questions and over 2 million corresponding answers.
- Additionally, translation applications leverage a dataset consisting of over 300,000 audio or speech recordings from non-native speakers.
(Source: SHAIP)
Data Pre-Processing and Cleaning Among Organizations
- The state of data pre-processing and cleaning among organizations reveals varying levels of readiness for artificial intelligence (AI) applications.
- As of the latest survey, 19% of respondents indicated that their data is consistently pre-processed. Their data strategy ensures it is always AI-ready.
- A larger segment, 40%, reported that most of their data is mostly pre-processed and primed for AI use.
- However, 33% of respondents acknowledged that their data is only occasionally pre-processed. Meaning that while some datasets are AI-ready, many still require additional work.
- Lastly, 8% of respondents admitted that their data is rarely pre-processed. Necessitating significant time and effort to clean and organize it for AI applications.
- This distribution highlights the varying degrees of data readiness and the ongoing challenges organizations face in achieving optimal data pre-processing for AI.
(Source: Cisco AI Readiness Index)
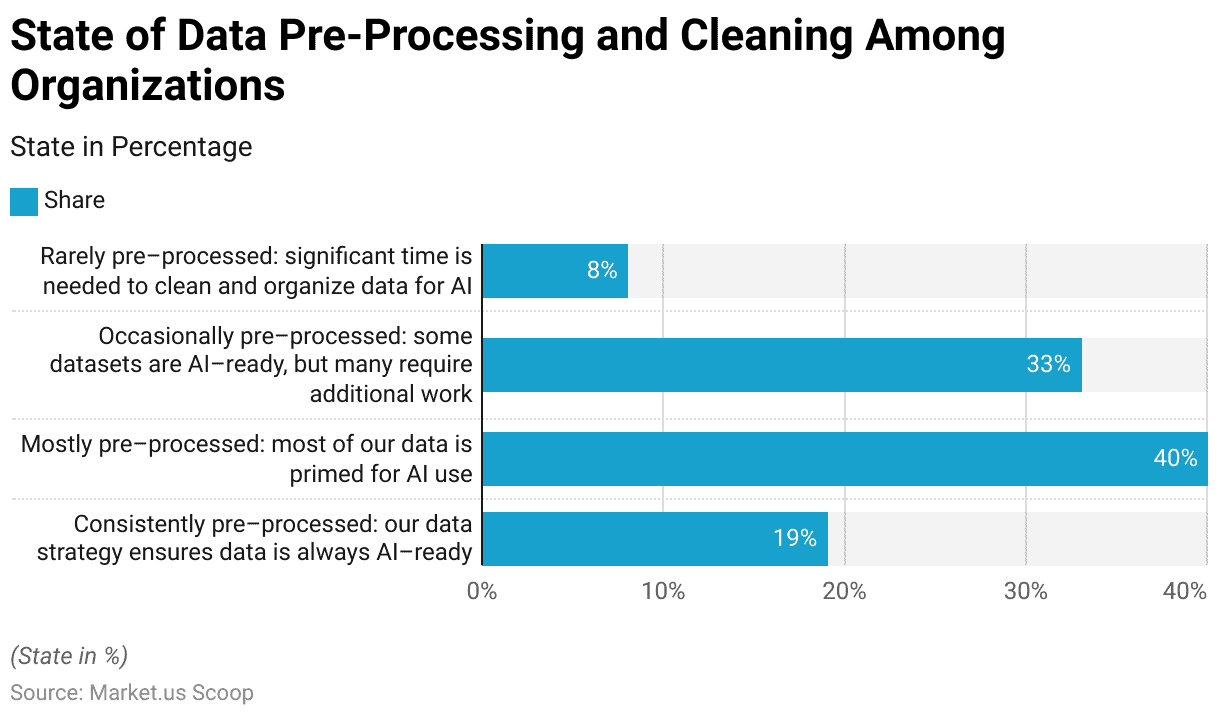
Data Sources Used by the Public Sector for Training AI Models
- As of October 2022, the public sector in South Korea utilized a variety of data sources for training artificial intelligence (AI) models.
- The predominant source was in-house data, excluding customer data, which was used by 56.3% of respondents.
- Public data was the next most common source, utilized by 36.3% of respondents.
- Customer data was employed by 24.1% of the respondents, indicating its significant role in AI model training.
- Additionally, private-public data sourced from the internet and social media accounted for 10.2% of usage.
- Private data was purchased by 7.8% of respondents.
- Other data sources were reported by 3.1% of respondents. Reflecting a smaller but notable diversity in data sourcing for AI training within the public sector.
(Source: Statista)
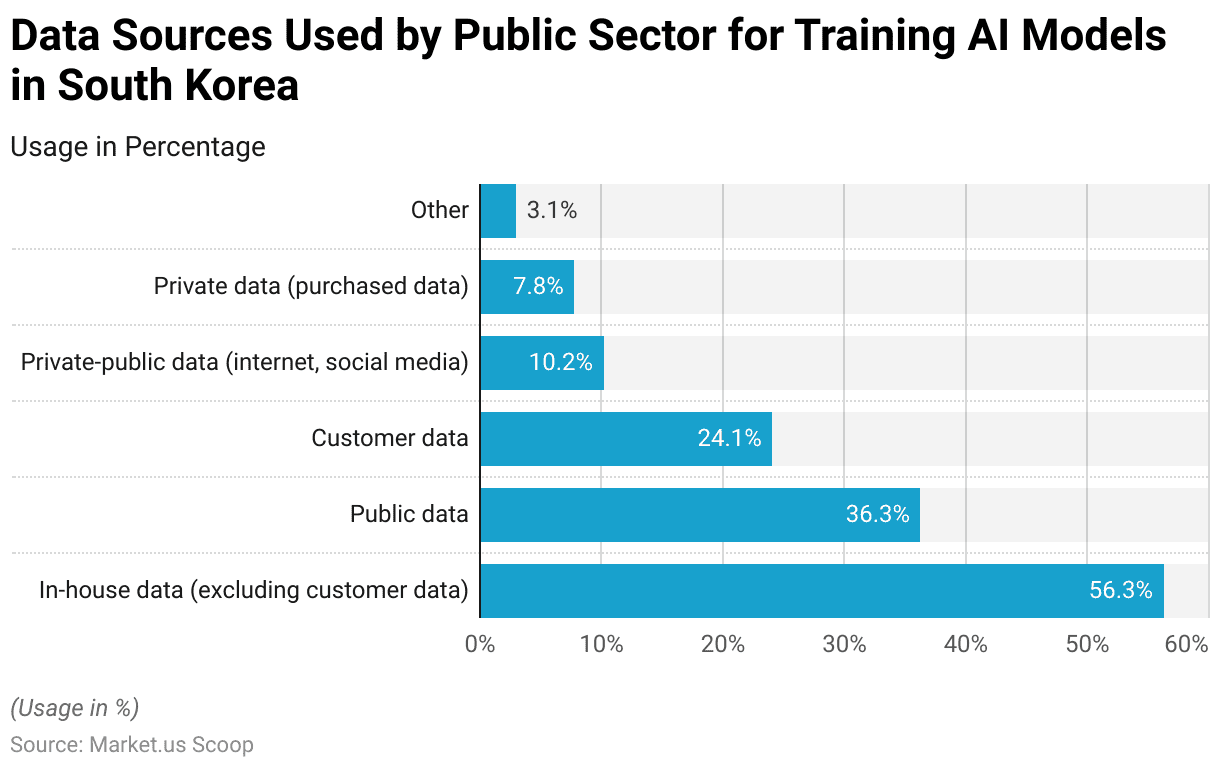
Popular AI Training Dataset Programs Statistics
- AI training dataset programs are crucial for developing robust machine learning models.
- Popular programs include Appen, which provides high-quality, diverse datasets across text, audio, image, and video modalities. Leveraging a global workforce of over 1 million to ensure detailed annotation and data quality.
- Google’s Open Images dataset offers over 9 million annotated images suitable for various computer vision tasks.
- Another notable mention is ImageNet, which contains over 14 million images across numerous categories and is widely used for image classification and object detection tasks.
- Companies like OpenAI collaborate with various partners to curate domain-specific datasets, such as their partnerships for enhancing language models with data from specific languages or industries.
- The use of these comprehensive datasets can range from financial data provided by Quandl to large-scale video datasets like Kinetics-700. It is integral in advancing AI capabilities across different sectors.
(Sources: SHAIP, V7 Labs, Appen, Open AI)
Level of Integration of Organizations’ Analytics Tools with Data Sources and AI Platforms Used
- The integration levels of organizations’ analytics tools with data sources and AI platforms vary significantly, reflecting diverse stages of technological maturity.
- As of the latest data, 26% of respondents reported that their analytics tools are fully integrated with their data sources and AI platforms, indicating a seamless and efficient data processing environment.
- A larger proportion, 43%, described their integration level as moderately integrated. Suggesting that while there is substantial connectivity, some areas may still require optimization.
- Additionally, 27% of respondents indicated that their tools are somewhat integrated. Pointing to partial connectivity that allows for basic functionality but lacks comprehensive integration.
- Finally, 4% of respondents reported no integration at all. Highlighting a critical gap that can impede effective data utilization and AI implementation.
- This spectrum of integration levels underscores the ongoing efforts and challenges faced by organizations in achieving full integration of their analytics tools with data and AI platforms.
(Source: Cisco AI Readiness Index)
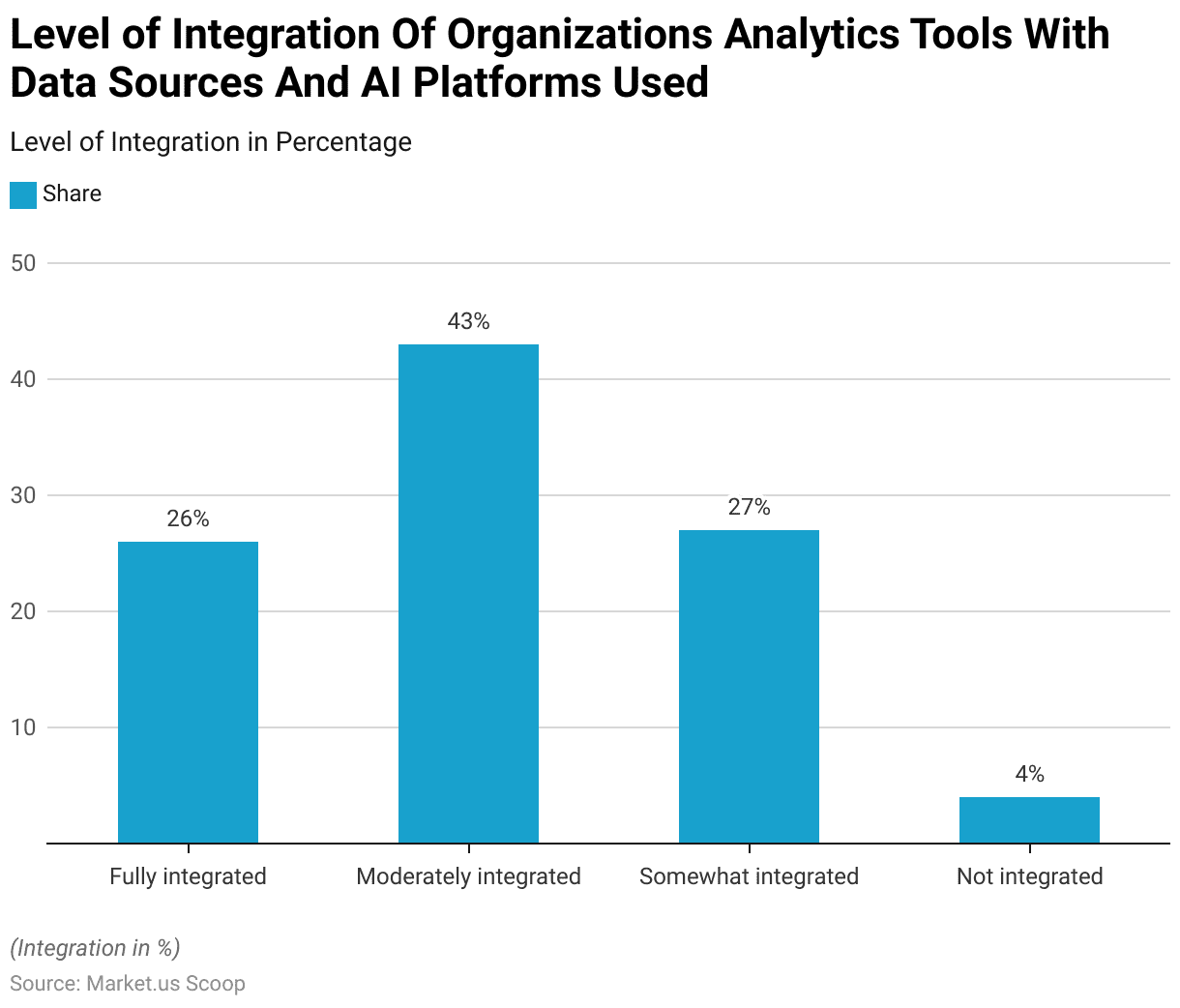
Sophistication of Organizations’ Analytics Tools in Handling Complex AI Dataset Training Statistics
- The sophistication of organizations’ analytics tools in handling complex AI-related datasets varies considerably.
- According to recent data, 24% of respondents rated their analytics tools as excellent in managing these intricate datasets, reflecting a high level of capability and efficiency.
- A significant portion, 43%, considered their tools to be good, indicating robust functionality and performance in most scenarios.
- Meanwhile, 26% of respondents described their tools as fair. Suggesting adequate performance but with room for improvement in handling complex data.
- Lastly, 7% of respondents rated their tools as basic, highlighting minimal capabilities that may hinder effective AI-related data processing.
- This range of responses illustrates the differing levels of advancement and effectiveness in organizations’ analytics tools for managing sophisticated AI datasets.
(Source: Cisco AI Readiness Index)
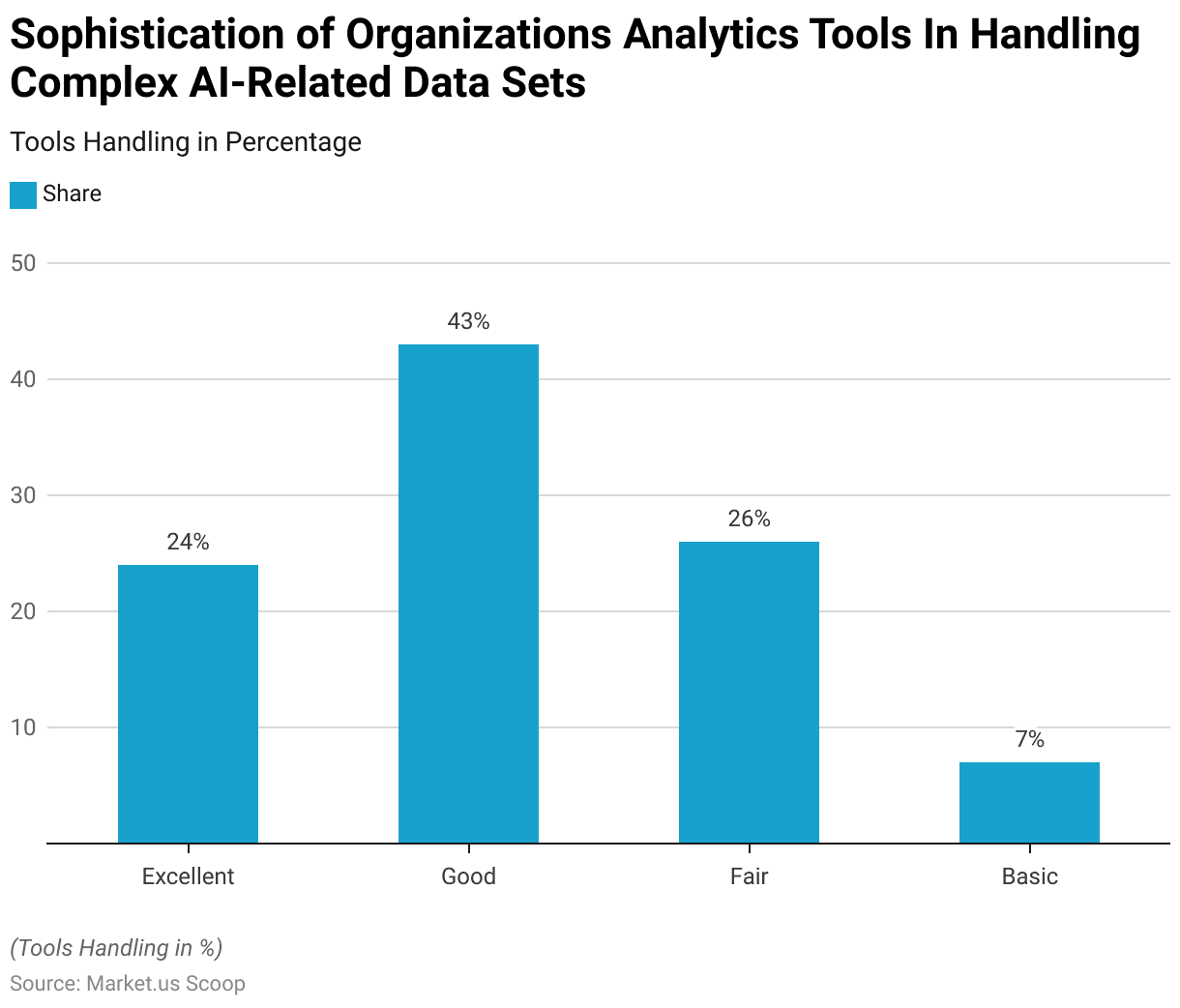
Adaptability and Scalability of Organizations’ Analytics Tools in Catering to Evolving Needs of AI Projects
- The adaptability and scalability of organizations’ analytics tools in meeting the evolving needs of AI projects show considerable variation.
- As of the latest survey, 30% of respondents reported that their analytics tools are highly adaptable. Indicating a strong capability to adjust and scale according to the dynamic requirements of AI initiatives.
- A larger segment, 42%, described their tools as moderately adaptable. Suggesting a good level of flexibility and scalability, though not without limitations.
- Additionally, 25% of respondents indicated that their tools are somewhat adaptable. Implying partial capability to adjust to new AI demands but with notable constraints.
- Finally, 3% of respondents reported that their tools are not adaptable. Highlighting a significant challenge in keeping pace with the evolving needs of AI projects.
- This distribution underscores the varying degrees of readiness among organizations to effectively adapt and scale their analytics tools for advancing AI applications.
(Source: Cisco AI Readiness Index)
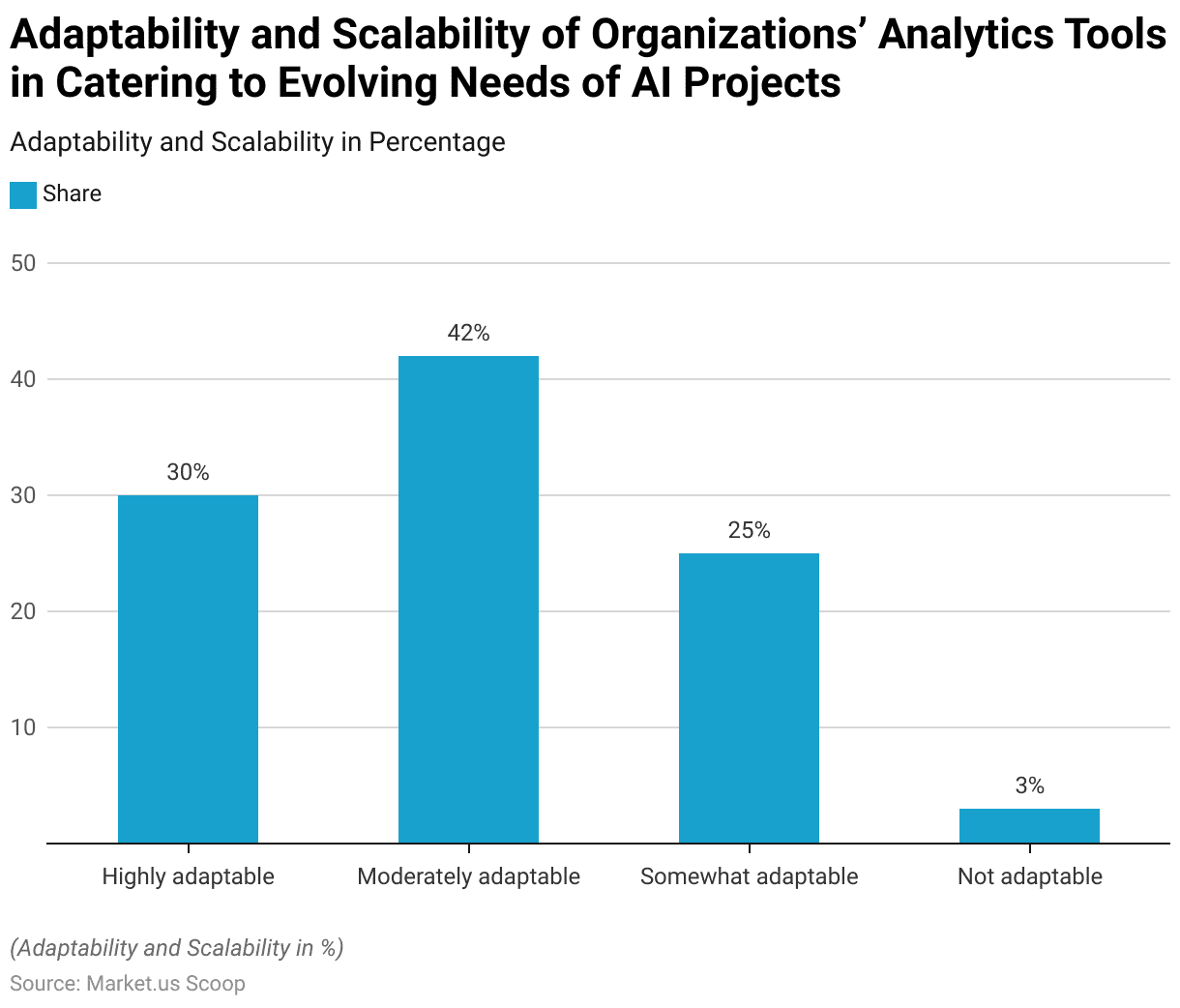
Technical Advancements in AI Training Dataset Statistics
- Recent advancements in AI training datasets have significantly enhanced the efficiency and accuracy of AI models across various applications.
- One notable development is the use of synthetic data generation, spearheaded by companies like NVIDIA and MIT.
- NVIDIA’s Nemotron-4 340B model generates synthetic data that mimics real-world characteristics, optimizing data quality and improving the performance of custom AI models.
- This approach is highly effective, particularly in scenarios where access to large, diverse, labeled datasets is limited.
- Additionally, MIT’s StableRep+ model, which combines synthetic imagery with language supervision, has achieved superior accuracy and efficiency compared to traditional models, demonstrating that synthetic data can rival or even surpass real data in training efficacy.
- Key players like Google, Amazon, and Microsoft are continuously launching new datasets to cater to the growing demand across sectors such as IT, healthcare, and automotive.
(Sources: Artificial Intelligence Index- Stanford Institute for Human-Centered Artificial Intelligence, NVIDIA, Tech Xplore, SciTech Daily)
Recent Developments
Acquisitions and Mergers:
- Scale AI acquires Helia.ai: In mid-2023, Scale AI, a company specializing in AI data labeling, acquired Helia.ai for $70 million. This acquisition aims to enhance Scale AI’s capabilities in generating high-quality training datasets. Particularly for autonomous driving and computer vision applications.
- Appen acquires Quadrant: Appen, a global leader in data for the AI lifecycle, acquired Quadrant, a location data provider, for $90 million in early 2024. This merger is expected to strengthen Appen’s data collection and annotation services by integrating Quadrant’s location intelligence solutions.
New Product Launches:
- Google’s Data Augmentation Platform: In late 2023, Google launched a data augmentation platform designed to automatically enhance training datasets by generating synthetic data. This platform aims to improve the robustness of AI models by increasing the diversity and quantity of training data.
- Amazon SageMaker Ground Truth Plus: Amazon Web Services (AWS) introduced SageMaker Ground Truth Plus in early 2024, offering enhanced data labeling services with built-in quality controls and automated workflows to ensure high-quality training datasets for machine learning models.
Funding:
- Labelbox raises $150 million: In 2023, Labelbox, a leading data labeling platform, secured $150 million in a Series C funding round to expand its platform capabilities and accelerate product development, focusing on improving dataset quality and annotation efficiency.
- Sama secures $100 million: Sama, a company providing data annotation and AI training data, raised $100 million in early 2024 to scale its operations and enhance its AI-driven annotation tools, aiming to deliver high-quality datasets faster.
Technological Advancements:
- AI-Powered Data Labeling: Advances in AI and machine learning are being used to automate the data labeling process, improving the accuracy and speed of creating training datasets. These technologies enable semi-supervised and unsupervised learning approaches to handle large-scale data.
- Synthetic Data Generation: The development of synthetic data generation techniques is allowing companies to create large volumes of high-quality training data without the need for real-world data collection, addressing privacy concerns and data scarcity.
Market Dynamics:
- Growth in AI Training Dataset Market: The global market for AI training datasets is projected to grow at a CAGR of 22.3% from 2023 to 2028, driven by the increasing adoption of AI across various industries, the need for large and diverse datasets, and advancements in data labeling technologies.
- Increased Demand for Domain-Specific Data: There is a rising demand for domain-specific training datasets tailored to particular industries such as healthcare, finance, and automotive, where specialized data is crucial for developing accurate AI models.
Regulatory and Strategic Developments:
- GDPR and Data Privacy Regulations: Companies providing AI training datasets are enhancing their data handling practices to comply with data privacy regulations such as GDPR and CCPA, ensuring that the data used for training AI models is anonymized and ethically sourced.
- US National AI Initiative: The US government’s National AI Initiative, implemented in early 2024, emphasizes the importance of developing high-quality training datasets to support AI research and innovation, providing funding and resources for data collection and annotation.
Research and Development:
- Bias Mitigation in Training Data: R&D efforts are focusing on identifying and mitigating biases in training datasets to ensure fair and unbiased AI models. Techniques such as data balancing, bias detection algorithms, and diverse data sourcing are being developed to address this challenge.
- Interactive Data Annotation Tools: Researchers are developing interactive data annotation tools that leverage human-in-the-loop approaches, combining human expertise with AI to improve the accuracy and efficiency of data labeling processes.
Conclusion
AI Training Dataset Statistics – The AI training dataset market is experiencing rapid growth. With revenues projected to soar from USD 1.9 billion in 2022 to USD 11.7 billion by 2032.
Key industries like IT, telecommunications, automotive, healthcare, and government are driving this expansion through applications in cybersecurity, customer care, fraud detection, and personalized services.
Despite significant progress, challenges remain in data pre-processing, tool integration, and adaptability, with varying levels of readiness among organizations.
Continued advancements in data management and more sophisticated analytics tools will be crucial for fully leveraging AI’s potential.
FAQs
An AI training dataset is a data collection used to train artificial intelligence (AI) models. This data can include text, images, video, and audio and is essential for teaching AI algorithms to recognize patterns, make predictions, and improve over time.
AI training datasets are crucial because they provide the foundational information that AI models need to learn and function accurately. High-quality, well-preprocessed datasets enable AI systems to perform tasks such as image recognition, natural language processing, and predictive analytics effectively.
AI training datasets can comprise various types of data, including text, images, video, and audio. Each type of data is used to train models for specific tasks, such as text for natural language processing. Images for visual recognition, and audio for speech recognition.
Data pre-processing involves cleaning and organizing raw data to make it suitable for AI training. This includes removing errors, handling missing values, normalizing data formats, and sometimes augmenting datasets to improve model accuracy.
AI training datasets are used across many industries, including IT and telecommunications, automotive, healthcare, banking, financial services and insurance (BFSI), retail and e-commerce, and government. Each industry utilizes AI for various applications, such as cybersecurity, customer service, fraud detection, and personalized services.
Discuss your needs with our analyst
Please share your requirements with more details so our analyst can check if they can solve your problem(s)
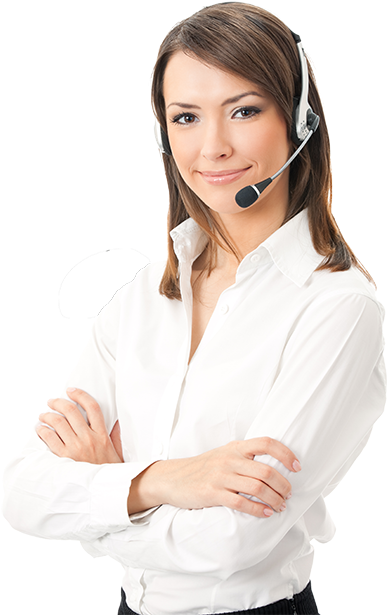