Table of Contents
Introduction
As reported by Market.us, The Global Large Language Model Market is projected to experience significant growth, with the market size expected to reach USD 82.1 billion by 2033, up from USD 4.5 billion in 2023. This represents a robust compound annual growth rate (CAGR) of 33.7% during the forecast period from 2024 to 2033. North America dominates the market, accounting for a 32.7% share in 2023, valued at USD 1.47 billion. This leadership position can be attributed to the region’s advanced technological infrastructure, high investment in AI research, and the presence of key market players.
Large Language Models (LLMs) are advanced AI systems designed to understand, generate, and manipulate human language. They are trained on vast datasets and use deep learning techniques to process text in a way that mimics human comprehension and response. These models have become integral in various applications such as chatbots, translation services, content generation, and more, due to their ability to handle complex language tasks with high efficiency.
The market for Large Language Models is growing rapidly, driven by the increasing demand for enhanced natural language processing tools across different industries. Businesses are leveraging LLMs to improve customer service, automate content creation, and refine search engine results. The education sector uses LLMs to assist with tutoring and personalized learning experiences, while the healthcare industry employs these models to interpret clinical notes and assist in diagnostic processes.
Demand for Large Language Models is on the rise due to their capability to streamline operations and offer insights through data analysis. Industries such as finance, healthcare, and customer service are particularly keen on integrating LLMs to automate routine tasks and provide analytical support. This growing demand is spurred by the need for efficiency and the desire to leverage big data for strategic advantages.
Large Language Models have gained popularity because of their versatility and effectiveness in various applications. Their ability to understand context and nuance in language has made them a valuable asset in fields ranging from AI research and software development to marketing and legal services. As AI becomes more embedded in everyday technology, the popularity of LLMs continues to soar, reflecting a broader adoption of AI tools in both business and consumer applications.
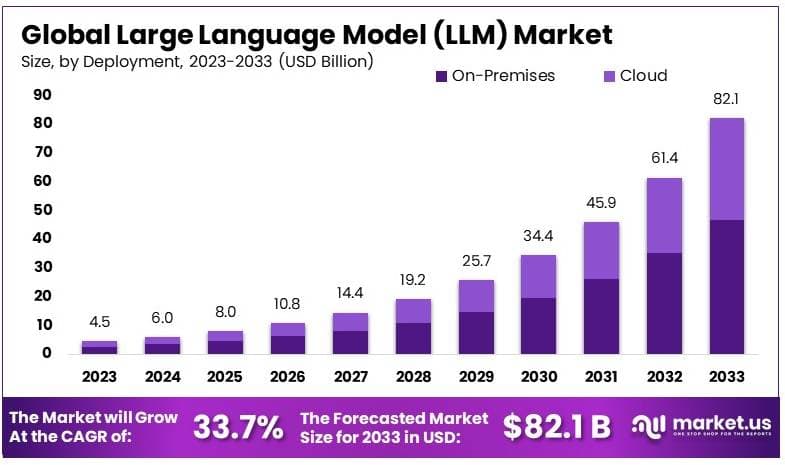
The market for Large Language Models is expanding, not only in size but also in scope. New advancements in AI and machine learning continually enhance the capabilities of LLMs, making them more accessible and applicable to a wider range of industries. Moreover, the geographic expansion is notable, with significant growth in regions such as Asia-Pacific and Europe, driven by increasing technological adoption and investment in AI infrastructure. This expansion is supported by collaborative efforts between academia, industry, and government to foster innovation and implement ethical AI practices.
“The Large Language Model (LLM) market is rapidly evolving, driven by increasing demand for advanced AI solutions that enhance understanding and interaction in various digital platforms. As businesses and individuals rely more on technology for communication, LLMs are becoming essential tools for providing accurate, real-time responses and insights. This market’s growth is a testament to the transformative impact of AI on global communication strategies.” says Yogesh Shinde, ICT – Manager at Market.us.
Drivers and Opportunities
The growth of the Large Language Model (LLM) market is propelled by several key drivers. One of the primary drivers is the abundant availability of internet data, which provides a robust base for training these models with diverse and expansive datasets. This improves the performance and versatility of LLMs, making them more effective across various applications. Additionally, advancements in hardware technologies like GPUs and TPUs have significantly reduced the time required for training and deploying these models, enhancing their feasibility for real-time applications.
Government policies and investments in AI research and development are also notable drivers. Many governments are increasingly focusing on AI to maintain a competitive edge globally, creating a conducive environment for the growth of LLM technologies. These policies, alongside regulatory frameworks concerning data privacy and ethics, help shape the deployment of LLMs, especially in sensitive sectors such as finance and healthcare.
The LLM market presents vast opportunities due to its wide applicability across different sectors. In the financial sector, LLMs are leveraged for tasks like risk assessment, fraud detection, and providing personalized financial advice, which are crucial for maintaining security and compliance in real-time financial operations. Another significant opportunity lies in the use of LLMs in customer service applications, where they enhance user engagement through chatbots and virtual assistants that offer personalized and instant responses.
The retail and e-commerce sector also stands out, with LLMs being used to personalize shopping experiences and improve customer service efficiency, thus driving sales and customer satisfaction. Moreover, the integration of LLMs into cloud computing platforms offers scalability and flexibility, which are vital for businesses looking to adapt quickly to changing market demands without substantial upfront investments.
Key Takeaways
- The Large Language Model (LLM) Market demonstrated significant growth dynamics in 2023, with a valuation of USD 4.5 Billion. It is projected to experience a robust Compound Annual Growth Rate (CAGR) of 33.7%, reaching an estimated USD 82.1 Billion by 2033. This growth trajectory can be attributed to the increasing integration of AI technologies across various sectors.
- In 2023, the On-Premises deployment segment led the market, securing a 57.7% share, primarily driven by escalating data privacy concerns among enterprises that prefer to manage sensitive data internally.
- The application of LLMs in Chatbots and Virtual Assistants was particularly notable, accounting for 27.1% of the market share. This reflects a growing trend towards leveraging AI-powered solutions to enhance customer interaction and service delivery.
- The Retail and E-Commerce industry emerged as a leading vertical, representing 27.5% of the market. This prominence underscores the sector’s adoption of AI to refine customer experience and streamline operations.
- Regionally, North America maintained a leading position, holding a 32.7% market share, a reflection of its advanced AI research and development infrastructure, which continues to drive innovation and adoption of cutting-edge technologies in the region.
Large Language Model (LLM) Statistics
- Natural Language Understanding: LLMs have shown a 15% increase in efficiency in natural language understanding tasks, indicating enhanced capabilities over previous iterations.
- Market Adoption: By 2025, it is estimated that 750 million applications will utilize LLMs, projecting extensive integration into digital infrastructures. These applications are expected to automate 50% of digital work, signifying a major shift towards AI-driven operational models.
- Market Share: In 2023, the world’s top five LLM developers collectively secured approximately 88.22% of the market revenue, highlighting a concentrated market dominance.
- Translation Accuracy: LLMs have reduced translation errors by 25% compared to earlier models, enhancing communication across different languages and reducing barriers in global operations.
- Code Generation and Problem Solving: Advanced LLMs like GPT-3, with 175 billion parameters, demonstrate profound capabilities in complex tasks such as code generation and logic problem-solving, expanding their utility in technical fields.
- Real-world Application Accuracy: In specific sectors like insurance, LLM applications show an accuracy of 22% when handling real business data, suggesting areas for improvement in model training and application.
- Personalization: 91% of clients regard personalized recommendations as crucial, underscoring the importance of tailored content generation capabilities of LLMs.
- Educational Applications: LLMs have been effectively used to create customized tests and quizzes, with students improving their test scores by 62% through personalized revision tools provided by AI.
- Organizational Usage: According to a report by Iopex, 67% of organizations utilize generative AI products powered by LLMs for language-related tasks, demonstrating widespread adoption across industries.
- Experimental and Commercial Deployment: A survey by Datanami in August 2023 revealed that 58% of companies are experimenting with LLMs, whereas only 23% have plans for commercial deployment or have already implemented such models.
- Content Generation: The use of LLMs in content generation has led to a 20% improvement in the quality of generated text, showcasing their growing sophistication in creating human-like content.
- Customer Support Efficiency: LLMs have improved customer support efficiency by 10%, leveraging AI chatbots to streamline service experiences.
- AI Development: LLMs have reduced the training time for new AI models by 30%, accelerating the development and deployment of AI technologies.
- Sentiment Analysis: In sentiment analysis tasks, LLMs have increased accuracy by 12%, enhancing the ability of AI to interpret and react to human emotions effectively.
- AI Research: The contribution of LLMs to AI research is notable, with a 40% increase in annual AI research papers, reflecting their integral role in advancing AI knowledge.
- Medical Diagnostics: In healthcare, LLMs have helped reduce diagnostic errors by 35%, supporting professionals in achieving more accurate diagnoses.
- Advertising Impact: LLM application in advertising has yielded a 22% increase in ad engagement, highlighting their effectiveness in engaging consumers.
- Financial Predictions: LLMs have improved the accuracy of financial predictions by 28%, indicating their potential to enhance decision-making in the financial sector.
- Startup Ecosystem: The influence of LLMs has led to a 50% increase in startups focusing on AI applications, emphasizing the expanding investment in AI-driven solutions.
Top 21 leading Large Language Models
LLM Name | Developer | Release Date | Access | Parameters |
GPT-4o | OpenAI | May 13, 2024 | API | Unknown |
Claude 3.5 | Anthropic | June 20, 2024 | API | Unknown |
Grok-1 | xAI | November 4, 2023 | Open-Source | 314 billion |
Mistral 7B | Mistral AI | September 27, 2023 | Open-Source | 7.3 billion |
PaLM 2 | May 10, 2023 | Open-Source | 340 billion | |
Falcon 180B | Technology Innovation Institute | September 6, 2023 | Open-Source | 180 billion |
Stable LM 2 | Stability AI | January 19, 2024 | Open-Source | 1.6 billion, 12 billion |
Gemini 1.5 | Google DeepMind | February 2nd, 2024 | API | Unknown |
Llama 3.1 | Meta AI | June 23, 2024 | Open-Source | 405 billion |
Mixtral 8x22B | Mistral AI | April 10, 2024 | Open-Source | 141 billion |
Inflection-2.5 | Inflection AI | March 10, 2024 | Proprietary | Unknown |
Jamba | AI21 Labs | March 29, 2024 | Open-Source | 52 billion |
Command R | Cohere | March 11, 2024 | Both | 35 billion |
Gemma | Google DeepMind | February 21, 2024 | Open-Source | 2 billion, 7 billion |
Phi-3 | Microsoft | April 23, 2024 | Both | 3.8 billion |
XGen-7B | Salesforce | July 3, 2023 | Open-Source | 7 billion |
DBRX | Databricks’ Mosaic ML | March 27, 2024 | Open-Source | 132 billion |
Pythia | EleutherAI | February 13, 2023 | Open-Source | 70 million to 12 billion |
Sora | OpenAI | February 15, 2024 (announced) | API | Unknown |
Alpaca 7B | Stanford CRFM | March 13, 2023 | Open-Source | 7 billion |
Nemotron-4 | Nvidia | June 14, 2024 | Open-Source | 340 billion |
Emerging Trends
- No-Code Development Platforms: The increasing integration of LLMs with no-code platforms is enabling businesses and individuals to leverage AI for tasks like speech transcription and content summarization without extensive programming knowledge.
- Customizable Small Language Models (SLMs): The shift towards smaller, more efficient models that can operate on edge devices and be tailored for specific tasks is gaining traction. These models offer advantages in performance and reduced training times compared to their larger counterparts.
- Ethical AI and Transparency: There is a growing focus on developing LLMs with an emphasis on ethical guidelines and transparency in their operations and training data, making them more trustworthy and accessible for research and application across various fields.
- Enhanced Interactivity and Personalization: LLMs are being used to create more dynamic and personalized digital experiences, from customer service bots to personalized learning and content creation.
- Expansion in Non-English Languages: Increasing efforts are being directed towards training LLMs in multiple languages, broadening their usability and inclusiveness globally.
Top Use Cases
- Content Generation: LLMs are extensively used for generating text, from drafting emails to creating long-form content, thereby enhancing productivity in content-driven industries.
- Customer Support Automation: By automating responses and handling inquiries, LLMs improve efficiency and customer satisfaction in support services. They are capable of understanding context and sentiment, which enhances interaction quality.
- Language Translation and Localization: LLMs provide real-time translation and localization services that are crucial for global business operations, ensuring content is culturally and contextually appropriate across different languages.
- Educational Applications: In the field of education, LLMs offer personalized learning experiences, generate practice questions, and adapt content according to individual learner needs, thereby democratizing access to education.
- Cybersecurity: LLMs are used to analyze large volumes of data for potential security threats, improving the speed and accuracy of threat detection and response in cybersecurity operations.
Major Challenges
- Data and Model Size: As LLMs become larger, the datasets required to train them grow exponentially, demanding significant computational power and energy. This scale presents logistical and environmental challenges, especially as the models’ energy consumption can be massive during training phases.
- Domain Adaptation: Generic LLMs often struggle when applied to specialized domains such as legal or medical fields due to their lack of specific domain knowledge. Tailoring these models to specific industries requires additional layers or fine-tuning, which can be complex and resource-intensive.
- Ethical and Bias Considerations: LLMs can perpetuate or even amplify biases present in their training data. Ensuring that these models are fair and unbiased is a critical challenge, necessitating ongoing efforts in model training and data curation to address potential ethical issues.
- Maintenance and Updates: Keeping LLMs up-to-date with the latest information and maintaining their performance over time requires continual retraining and updates. This process can be resource-intensive and difficult to manage, especially with the rapid pace of information change
- Accessibility and Scalability: Deploying LLMs effectively at scale poses significant challenges. Despite their capabilities, ensuring that these models are accessible and performant under heavy loads, similar to the usage levels seen by major tech platforms, requires substantial infrastructure and optimization.
Top Opportunities
- Enhanced Natural Language Understanding: LLMs offer superior capabilities in understanding and generating human-like text, which can revolutionize areas such as chatbots, personal assistants, and customer service automation.
- Educational Applications: LLMs have the potential to personalize learning and offer scalable solutions for educational challenges, such as automatic question generation, tutoring systems, and even grading.
- Content Creation: In fields like journalism, advertising, and entertainment, LLMs can assist in generating creative content, from writing articles to composing music or scripting video content, thereby augmenting human creativity with AI efficiency.
- Business Intelligence and Analytics: LLMs can analyze large volumes of text data to extract insights, make predictions, and support decision-making processes in business environments, offering a significant advantage in market analysis and strategic planning.
- Healthcare and Research: LLMs can aid in medical research by analyzing scientific papers, generating medical documentation, and providing support for diagnostic processes, potentially improving healthcare outcomes through more informed decision-making.
Conclusion
The Large Language Model market is poised for significant growth and diversification as industries across the globe recognize the transformative potential of these AI technologies. As LLMs become more sophisticated and integrated into various sectors, they not only enhance operational efficiencies but also drive innovation.
The continuous development and ethical implementation of these models will be crucial in ensuring they contribute positively to technological advancement and societal benefit. The future of LLMs looks promising, with vast opportunities for expansion and improvement in capabilities that could redefine how businesses and consumers interact with AI.
Discuss your needs with our analyst
Please share your requirements with more details so our analyst can check if they can solve your problem(s)
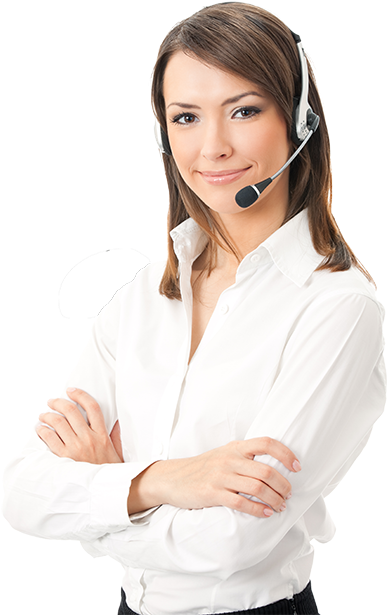