Table of Contents
- Introduction
- Editor’s Choice
- History and Evolution of Artificial Intelligence Chipset Statistics
- Artificial Intelligence Chipset Market Statistics
- AI Chip Revenue Statistics
- AI Chipset Specifications
- Trends in the Number of Chipmakers and Photolithography Companies
- Cost Involved in Chip Designing
- Leading U.S. and Chinese AI chips
- Studies Performed to Analyze AI Chip Efficiency and Speed
- Sales Price of Chips
- Key Investment Statistics
- Innovations and Developments in AI Chipsets
- Regulations for AI Chipsets
- Recent Developments
- Conclusion
- FAQs
Introduction
Artificial Intelligence Chipset Statistics: AI chipsets are specialized hardware designed to accelerate artificial intelligence tasks, particularly in machine learning and deep learning.
They include GPUs, TPUs, FPGAs, ASICs, and NPUs, each optimized for parallel processing, enabling faster and more efficient data handling.
These chipsets are crucial for applications such as autonomous vehicles, smartphones, and cloud computing, where speed and energy efficiency are key.
By processing large volumes of data through neural networks, AI chipsets enhance performance and scalability, making them essential for real-time AI operations and complex model training across industries.
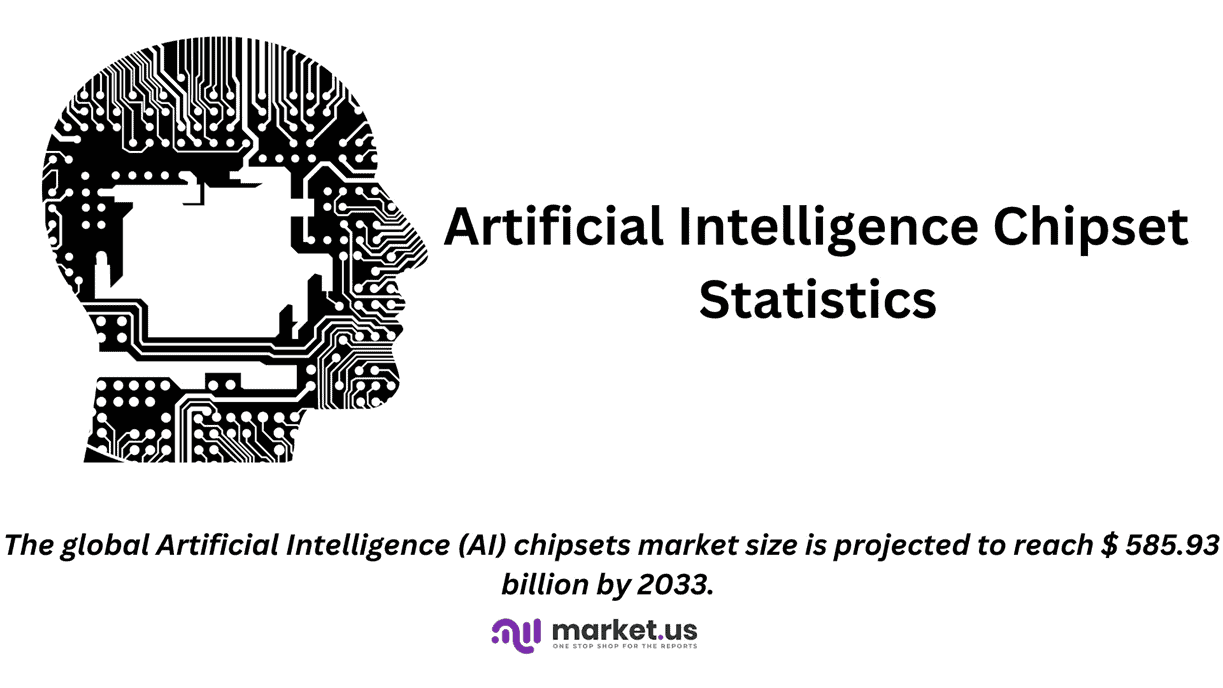
Editor’s Choice
- Significant milestones in AI hardware include the creation of chips like Google’s TPUs (Tensor Processing Units), which revolutionized machine learning applications by accelerating the training and deployment of AI models.
- The global Artificial Intelligence (AI) chipsets market size is projected to reach $ 585.93 billion by 2033.
- In 2021, the global AI chipsets market was led by Advanced Micro Devices Inc. (AMD), holding a 13% market share.
- In 2021, North America dominated the global AI chipsets market with a 37.4% share.
- Stanford (2017) showed that Nvidia Tesla K80 GPUs outperformed Intel Broadwell vCPUs by 2x to 12x in training ResNet and 5x to 3x in inference.
- From 2013 to 2023, private investments in artificial intelligence (AI) worldwide were led by the United States, which received a total of USD 335.24 billion.
- The United States has implemented stringent export controls, particularly affecting trade with China, emphasizing restrictions on the sale and distribution of high-end AI chips used in advanced computing applications.
History and Evolution of Artificial Intelligence Chipset Statistics
- The history and evolution of AI chipsets can be traced back to several pivotal developments in artificial intelligence and computing technology, illustrating a journey from theoretical concepts to sophisticated, specialized hardware.
- The roots of AI technology start with early philosophical and logical ideas, such as those of Aristotle and, later, the computational advances of Blaise Pascal and Gottfried Wilhelm Leibniz.
- The term “artificial intelligence” itself was coined in 1956 by John McCarthy at the Dartmouth Conference, setting the stage for the formal field of AI.
- The development of AI chipsets began to gain real momentum with the emergence of specialized hardware designed to optimize AI tasks.
- This includes GPUs (Graphics Processing Units) primarily used for developing and refining AI algorithms through training, and ASICs (Application-Specific Integrated Circuits) and FPGAs (Field-Programmable Gate Arrays) used for inference, the application of these algorithms to real-world data.
- The superiority of these AI chips lies in their ability to perform tasks significantly faster and more efficiently than general-purpose CPUs, making them integral to pushing the boundaries of what AI can achieve in practical applications.
- Significant milestones in AI hardware include the creation of chips like Google’s TPUs (Tensor Processing Units), which revolutionized machine learning applications by accelerating the training and deployment of AI models.
- The development of AI chipsets has been characterized by rapid advancements in efficiency, reducing both the cost and environmental impact of AI applications.
- Today, the AI chipset industry continues to evolve, driven by the need for more powerful, efficient, and cost-effective solutions in a range of applications, from natural language processing to autonomous driving.
- This evolution reflects a broader trend in AI development, from foundational algorithms to transformative applications across various sectors.
(Sources: Coursera, CSET, IEEE Xplore, The Tech Historian)
Artificial Intelligence Chipset Market Statistics
Global Artificial Intelligence Chipset Market Size Statistics
- The global Artificial Intelligence (AI) chipsets market has experienced significant growth over the past few years at a CAGR of 36.8%, with its size expanding from USD 13.64 billion in 2021 to an estimated USD 18.66 billion in 2022.
- This upward trajectory continued in 2023, reaching USD 25.53 billion.
- Projections indicate that the market will maintain its robust growth, reaching USD 34.92 billion in 2024 and further accelerating to USD 47.77 billion by 2025.
- By 2026, the market is expected to surpass USD 65.35 billion, and by 2027, it will reach USD 89.40 billion.
- Over the next decade, the market is set to experience exponential growth, reaching USD 122.30 billion in 2028, USD 167.30 billion in 2029, and USD 228.87 billion in 2030.
- The market will continue its upward trend, reaching an estimated USD 313.09 billion in 2031 and USD 428.31 billion in 2032, with a potential value of USD 585.93 billion by 2033.
- This growth reflects the increasing demand for AI-enabled technologies and the expanding applications of AI across various industries.
(Source: market.us)
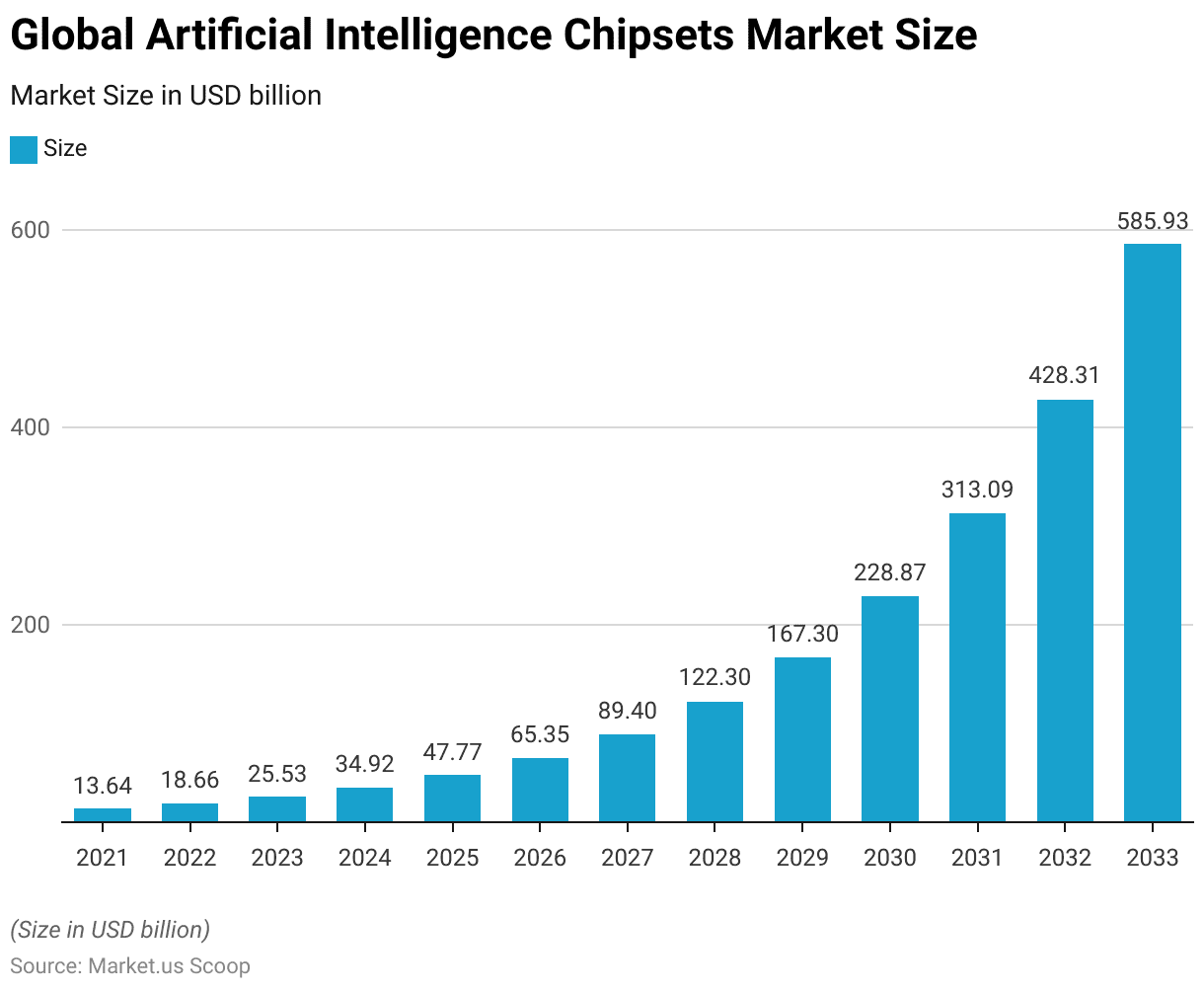
Competitive Landscape of Global Artificial Intelligence Chipset Market Statistics
- In 2021, the global Artificial Intelligence (AI) chipsets market was dominated by several key players, each holding a significant market share.
- Advanced Micro Devices Inc. (AMD) led with a 13% market share, closely followed by Google Inc. and Intel Corporation, each with a 12% share.
- Baidu Inc. and Graphcore each captured 11% of the market, while Qualcomm Technologies, Inc. held 9%.
- Apple Inc. accounted for 8%, and Mythic secured 6%.
- Huawei Technologies Co., Ltd. and Xilinx Inc. rounded out the competitive landscape with 6% and 5% market shares, respectively.
- Other key players shared the remaining 7% of the market in the industry.
- This distribution reflects the competitive nature of the AI chipsets market, with several major technology companies vying for market dominance.
(Source: market.us)
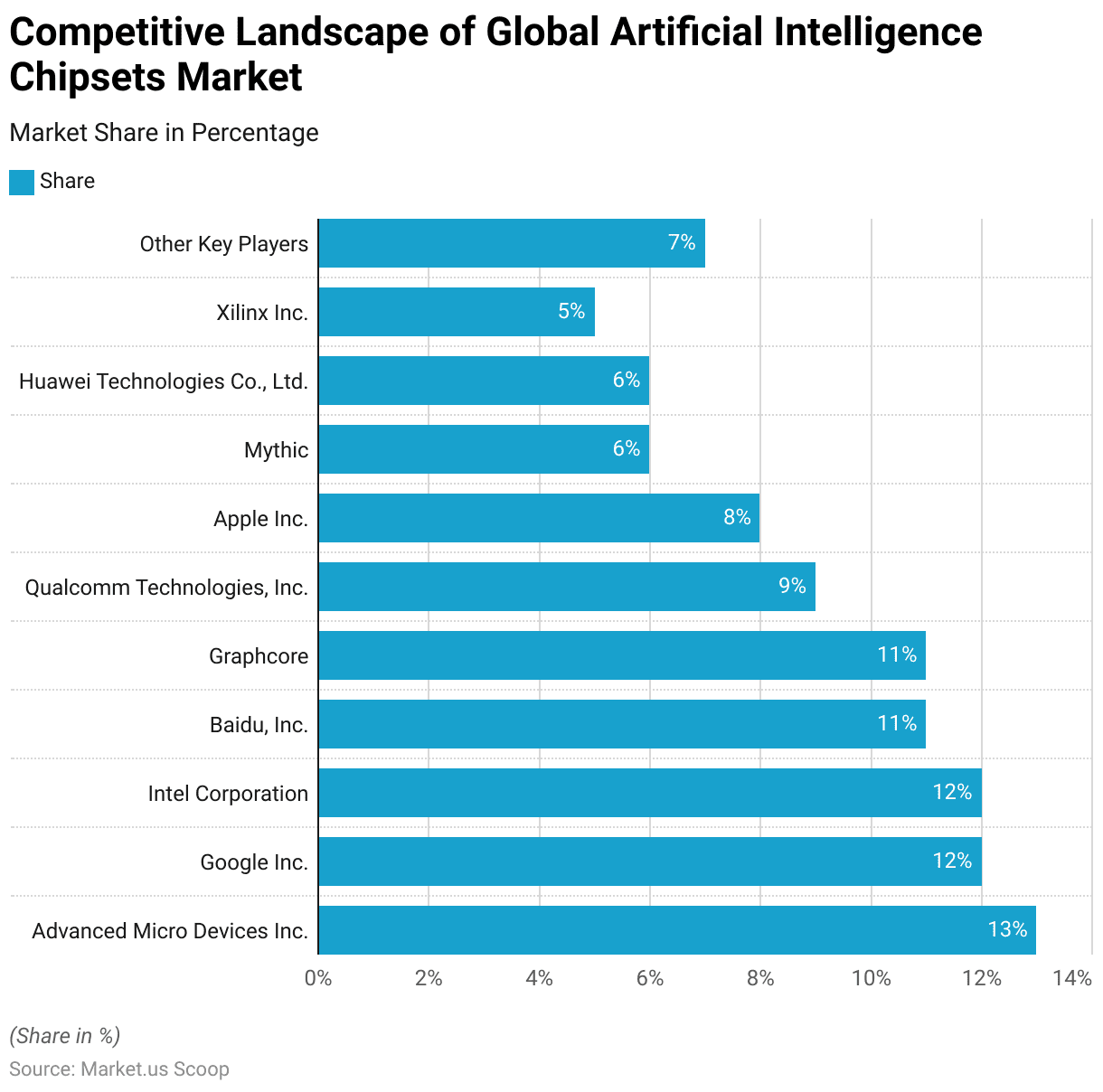
Global Artificial Intelligence Chipset Market Share – By Region Statistics
- In 2021, the global Artificial Intelligence (AI) chipsets market exhibited a diverse regional distribution.
- North America held the largest market share, accounting for 37.4% of the total market.
- This was followed by the Asia-Pacific (APAC) region, which captured 28.0% of the market.
- Europe represented 23.0% of the market share, while South America contributed 8.0%.
- The Middle East and Africa (MEA) region held the smallest share, with 3.6%.
- These regional figures highlight the dominance of North America in the AI chipsets market, with significant contributions from APAC and Europe as well.
(Source: market.us)
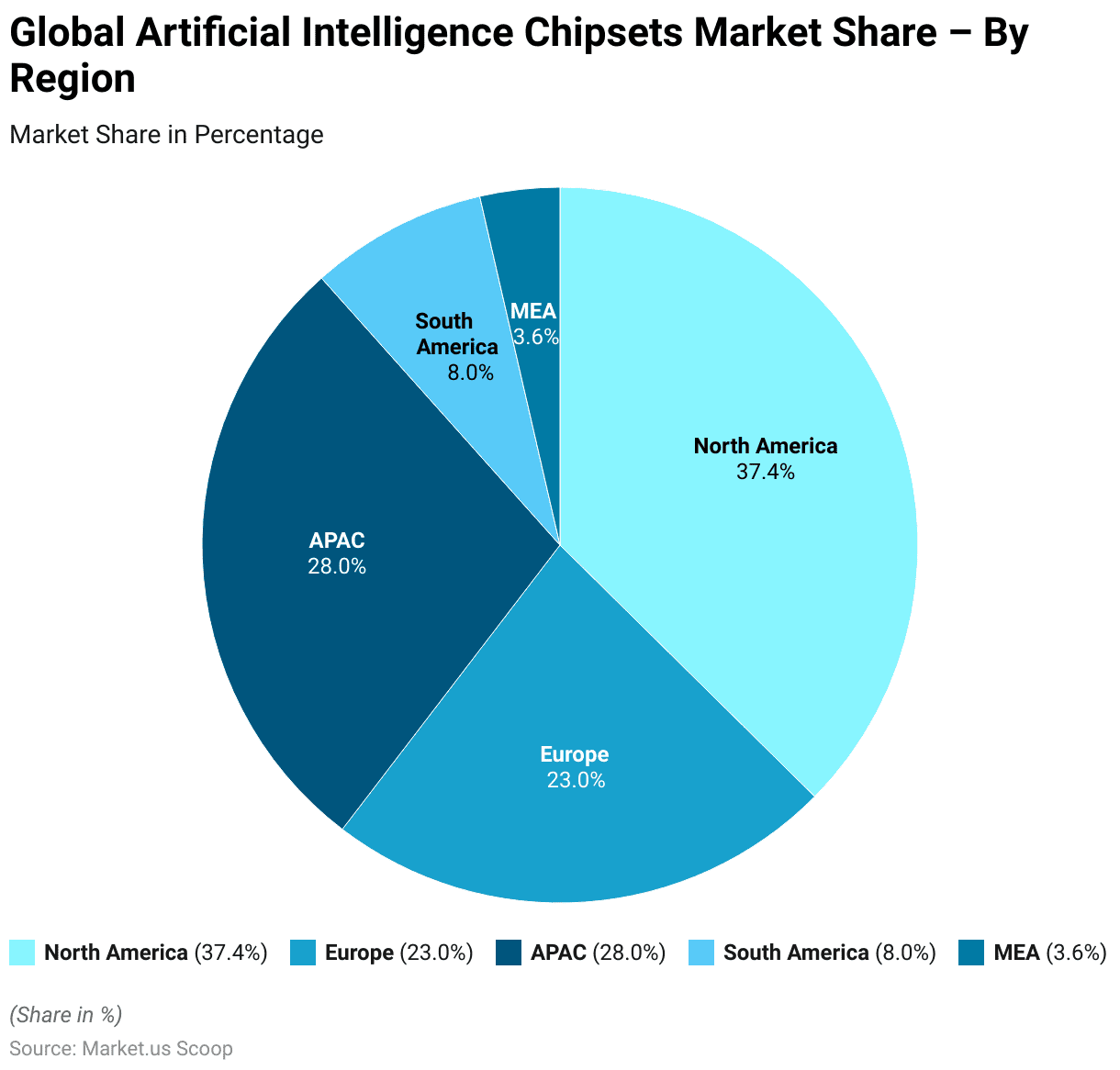
AI Chip Revenue Statistics
Artificial Intelligence Chipset Chip Market Revenue Worldwide Statistics
- The global Artificial Intelligence (AI) chip market is projected to grow significantly in the coming years.
- In 2023, the market size was estimated at USD 53.66 billion, with expectations of further expansion to USD 71.25 billion in 2024.
- By 2025, the market is anticipated to reach USD 91.96 billion.
- This growth reflects the increasing demand for AI chips, driven by the adoption of AI technologies across various industries.
(Source: Statista)
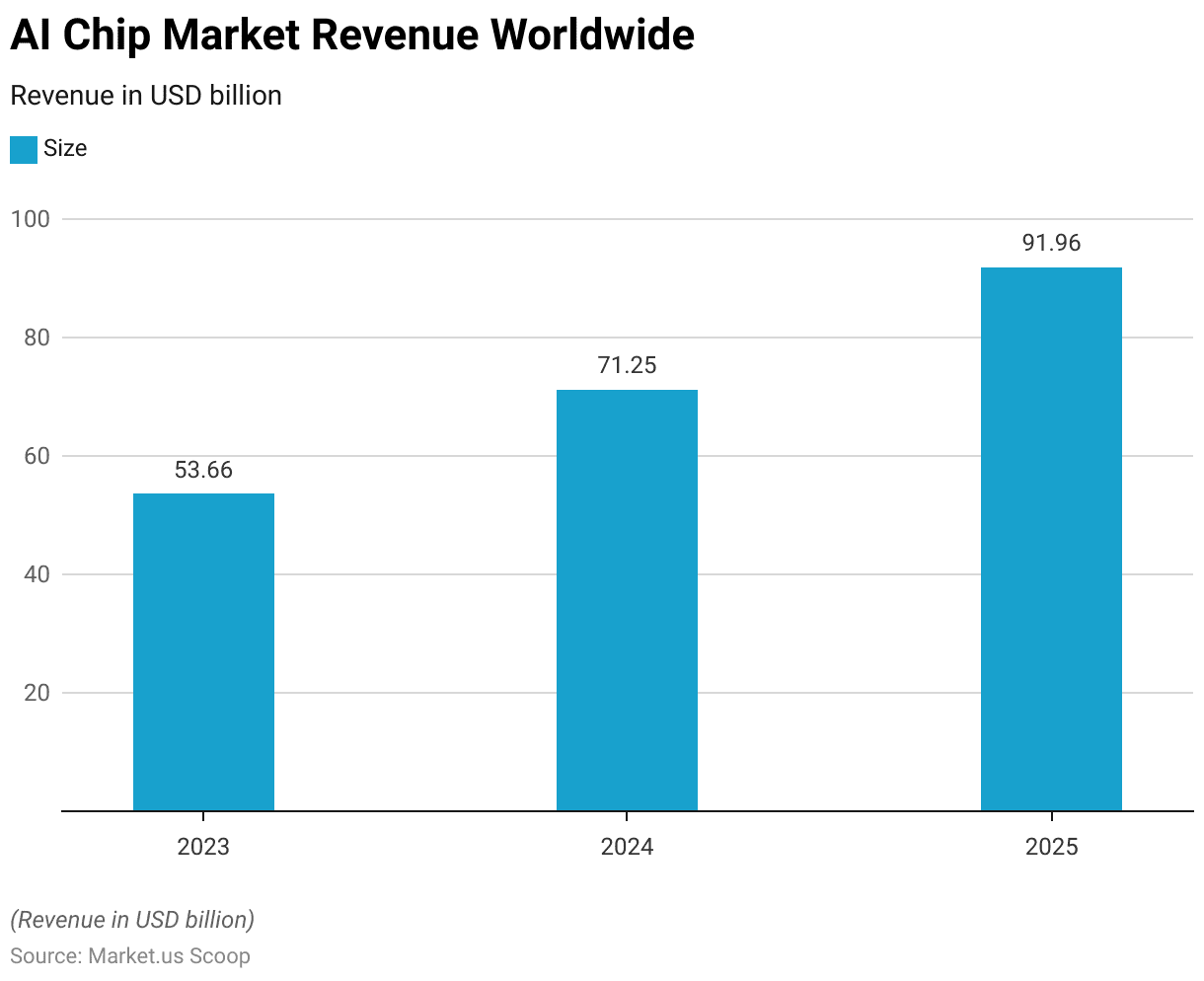
Revenue of Artificial Intelligence Chipset Chips Used in Data Centers and Edge Computing Statistics
- In 2017, the revenue generated from Artificial Intelligence (AI) chips used in data centers was USD 6 billion, while revenue from AI chips in edge computing was significantly smaller, at USD 0.2 billion.
- By 2025, both segments are expected to experience substantial growth.
- The revenue from AI chips in data centers is projected to reach USD 15 billion, while edge computing is anticipated to see a sharp increase, with revenue rising to USD 6 billion.
- This shift reflects the growing demand for AI-powered solutions in both centralized data environments and decentralized edge computing applications.
(Source: Statista)
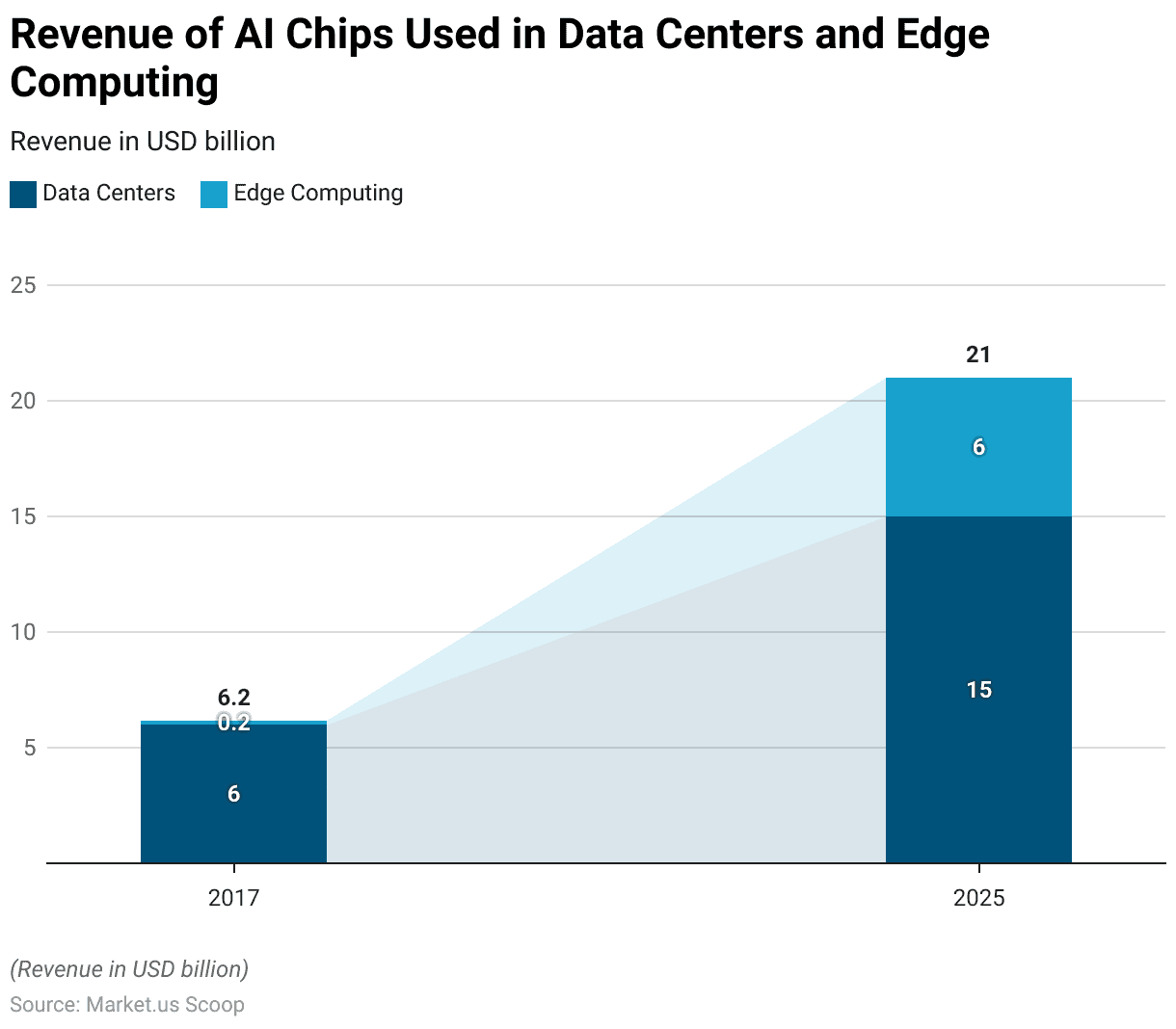
AI Chipset Specifications
Artificial Intelligence Chipset Statistics by Qualcomm
- Qualcomm offers a range of AI chipsets with varying specifications designed for different use cases.
- The AI 100 Ultra, in a PCIe FH3/4L form factor (111.2mm x 237.9mm), operates at 150W, delivering up to 870 TOPS in peak integer operations (INT8) and 288 TFLOPS in peak floating point operations (FP16).
- It is equipped with 576 MB of SRAM, 128 GB of DRAM with LPR4x, and a DRAM bandwidth of 548 GB/s, with a PCIe Gen4 interface supporting 16 lanes.
- The AI 80 Ultra, also in a PCIe FH3/4L form factor, has similar power requirements (150W) but offers up to 618 TOPS and 222 TFLOPS, with each SoC providing 155 TOPS and 56 TFLOPS, respectively.
- It also includes 576 MB of SRAM, 128 GB of DRAM, and the same DRAM bandwidth and host interface as the AI 100 Ultra.
- The AI 100 Pro, in a smaller PCIe HHHL form factor (68.9mm x 169.5mm), runs at 75W, offering up to 400 TOPS and 200 TFLOPS, with 144 MB of SRAM and 32 GB of LPR4x DRAM at 137 GB/s bandwidth, connected via PCIe Gen4 with eight lanes.
- Similarly, the AI 100 Standard, in the same HHHL form factor, operates at 75W, providing up to 350 TOPS and 175 TFLOPS with 126 MB of SRAM and 16 GB of DRAM at 137 GB/s.
- Lastly, the AI 80 Standard, also at 75W, offers up to 190 TOPS and 86 TFLOPS, with 126 MB of SRAM and 16 GB of DRAM, connected via PCIe Gen4 with eight lanes.
(Source: Qualcomm)
Cerebras Artificial Intelligence Chipset Statistics
- The Cerebras WSE-3 outperforms the Nvidia H100 in several key areas, including AI-optimized cores, memory speed, and on-chip fabric bandwidth.
- The WSE-3 features an impressive chip size of 46,225 mm², significantly larger than the Nvidia H100’s 814 mm², making it 57 times larger.
- It boasts 900,000 cores, vastly surpassing the H100’s 16,896 FP32 cores and 528 Tensor cores, a 52-fold increase.
- In terms of on-chip memory, the WSE-3 is equipped with 44 gigabytes, compared to the Nvidia H100’s 0.05 gigabytes, offering an 880-fold advantage.
- The WSE-3 also leads in memory bandwidth, with 21 petabytes per second, a massive improvement over the H100’s 0.003 petabytes per second, providing a 7,000-fold increase.
- Finally, the WSE-3 achieves 214 petabits per second in fabric bandwidth, far surpassing the H100’s 0.0576 petabits per second, resulting in a 3,715-fold improvement.
- This makes the Cerebras WSE-3 an exceptional processor in AI applications, with a substantial edge over the Nvidia H100 across multiple performance metrics.
(Source: Cerebras)
Artificial Intelligence Chipset Statistics by NVIDIA
- The NVIDIA DGX A100 640GB system is a powerful AI platform designed for high-performance computing.
- It features 8 NVIDIA A100 80GB Tensor Core GPUs, providing a total of 640GB of GPU memory.
- The system delivers an impressive five petaFLOPS of AI performance and ten petaOPS of INT8 processing power.
- 6 NVIDIA NVSwitches facilitate connectivity, and the system is powered by dual AMD Rome 7742 CPUs, offering 128 cores with a base clock of 2.25 GHz and a max boost of 3.4 GHz.
- The DGX A100 is equipped with 2TB of system memory and supports networking speeds of up to 200 Gb/s with NVIDIA ConnectX-7 and ConnectX-6 VPI for InfiniBand and Ethernet connectivity.
- Storage includes two 1.92TB M.2 NVMe drives for the OS and 30TB of internal storage via 8x 3.84TB U.2 NVMe drives.
- The system runs on Ubuntu Linux OS but also supports Red Hat Enterprise Linux and CentOS.
- With a maximum system weight of 271.5 lbs (123.16 kgs) and a packaged weight of 359.7 lbs (163.16 kgs), its dimensions are 10.4 inches in height, 19.0 inches in width, and 35.3 inches in length.
- The DGX A100 operates within a temperature range of 5ºC to 30ºC (41ºF to 86ºF), making it suitable for demanding environments.
(Source: NVIDIA)
Intel Artificial Intelligence Chipset Statistics
- The Intel® Z890 chipset, designed for Intel® Core™ Ultra Desktop Processors (Series 2), offers a range of high-performance features.
- It provides 34 high-speed I/O lanes and 8 DMI Gen 4 lanes for fast data transfer.
- The chipset supports up to 24 PCIe 4.0 lanes, 8 SATA 3.0 ports, and a variety of USB ports, including 14 USB 2.0 ports, 5 USB 3.2 (20G) ports, 10 USB 3.2 (10G) ports, and 10 USB 3.2 (5G) ports. It also supports up to 4 eSPI chip selects.
- For processors, the chipset supports PCI 5.0 lane configurations such as 1×16 + 1×4, 2×8 + 1×4, or 1×8 + 3×4, and PCI 4.0 with 1×4 configuration.
- The system memory configuration is two channels per DIMM, with no support for ECC memory.
- The chipset allows simultaneous independent display support for up to 4 displays, as well as both IA and BLCK overclocking, along with memory overclocking capabilities.
- Storage features include Intel® Rapid Storage Technology 20.x, Intel® Volume Management Device, PCIe storage support, and RAID 0,1,5,10 support for both PCIe and SATA.
- Manageability options include Intel® Management Engine Firmware (Intel® ME 19.0 Consumer) but do not support Intel® vPro® with Active Management Technology or Intel® Standard Manageability.
- For security, the Z890 chipset includes Intel® Platform Trust Technology and Intel® Boot Guard, ensuring enhanced system integrity.
(Source: Intel)
Artificial Intelligence Chipset Statistics by Huawei – CMC Microsystems
- The CMC Microsystems Core offers various specifications across different versions of its architecture.
- The Davinci Max version supports a maximum of 8192 operations per cycle with vector operations per cycle of 256.
- It features execution units that are not bottlenecked, with memory bandwidth scaling at 3TB/s for A:8192, 2TB/s for B:2048, and 192GB/s for L2, distributed by 32, 8, and 2 lanes, respectively.
- The Davinci Lite version, designed for lower power applications, supports 4096 operations per cycle with a vector operation per cycle of 128 and a memory bandwidth of 38.4GB/s.
- The Davinci Tiny version is optimized for minimal operations, with 512 operations per cycle and a vector operation of 32, offering memory bandwidth for A:2048 and B:512 but no set bandwidth for L2.
- The architecture is designed to set performance baselines, minimize vector bounds, and avoid bottlenecks by limiting the NoC (Network on Chip) usage, ensuring that any of these constraints do not limit performance.
(Source: CMC Microsystems)
Trends in the Number of Chipmakers and Photolithography Companies
- Between 1999 and 2020, the number of chipmakers and photolithography companies experienced significant shifts, reflecting changes in the semiconductor industry.
- In 1999, there were 32 chipmakers and four photolithography companies.
- This number surged to 94 chipmakers in 2001, while the number of photolithography companies remained steady at 3.
- However, the landscape began to consolidate in the following years, with the number of chipmakers dropping to 72 by 2004 and 48 by 2006, while the number of photolithography companies declined to 2 in both cases.
- By 2009, the number of chipmakers had further decreased to 36, and this trend continued, with the number reaching 26 in 2011, 20 in 2014, and 16 in 2015.
- The decline accelerated in the subsequent years, with only 11 chipmakers remaining by 2017 and just five by 2018.
- By 2020, the number of chipmakers had decreased to a mere 3, while the number of photolithography companies dropped to 1.
- This data highlights the ongoing consolidation in the semiconductor industry, particularly in the context of advanced manufacturing technologies.
(Source: Center for Security and Emerging Technology – Georgetown University)
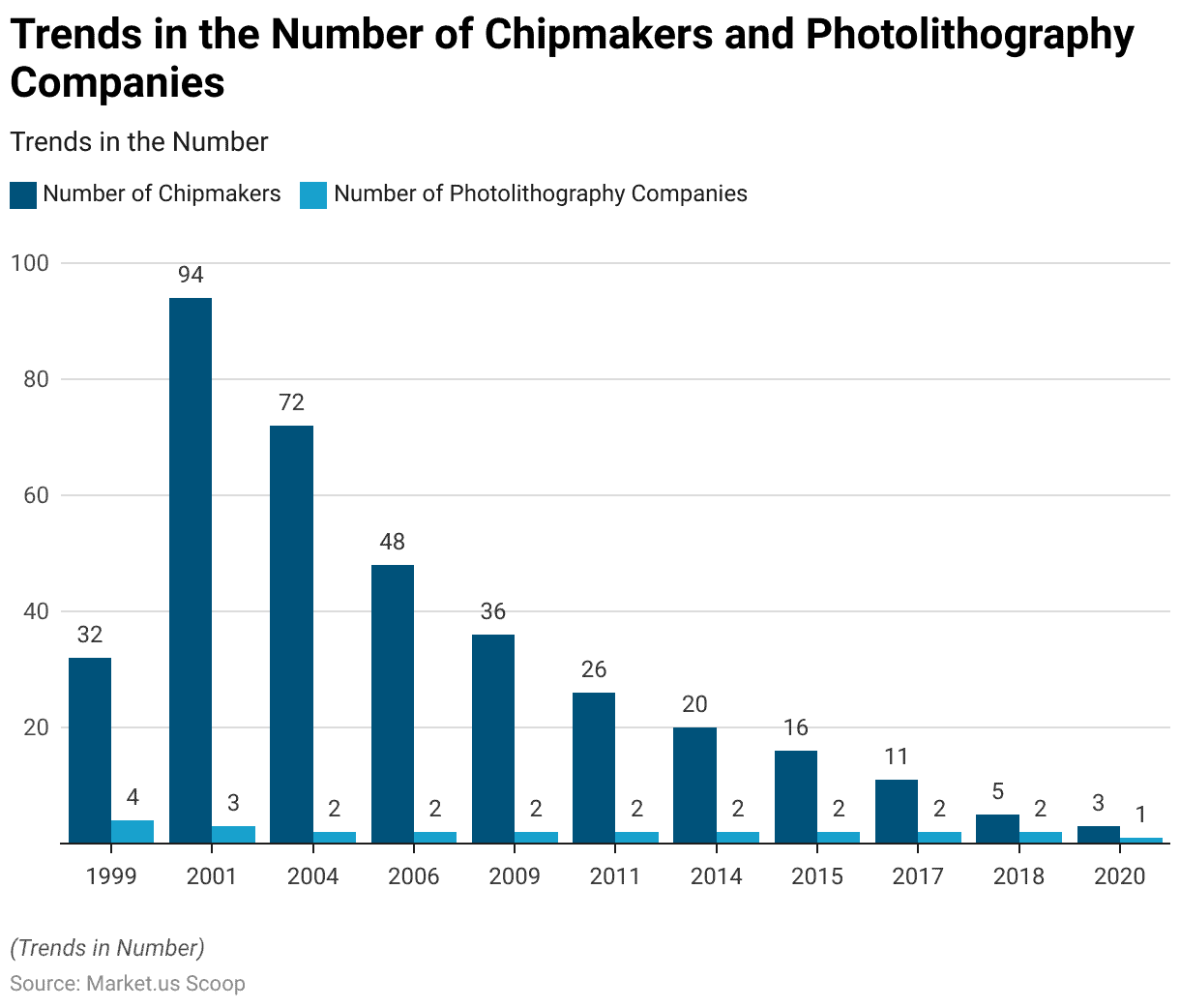
Cost Involved in Chip Designing
Chip Design Costs at Each Node
- The cost of chip design increases significantly as the node size shrinks.
- For instance, at the 65 nm node, chip design costs are approximately $28.5 million, while at the 40 nm node, the cost rises to $37.7 million.
- As technology progresses, the costs escalate further, with the 28 nm node requiring $51.3 million for chip design.
- The 22 nm node sees an even larger increase to $70.3 million. At the 16/14/12 nm nodes, the design cost reaches $106.3 million.
- The 10 nm node marks a substantial jump in costs, reaching $174.4 million, while the 7 nm node pushes the cost to $297 million.
- Finally, at the 5 nm node, chip design costs soar to $542.2 million.
- This data illustrates the exponential growth in costs associated with advancing semiconductor technology.
(Source: Center for Security and Emerging Technology – Georgetown University)
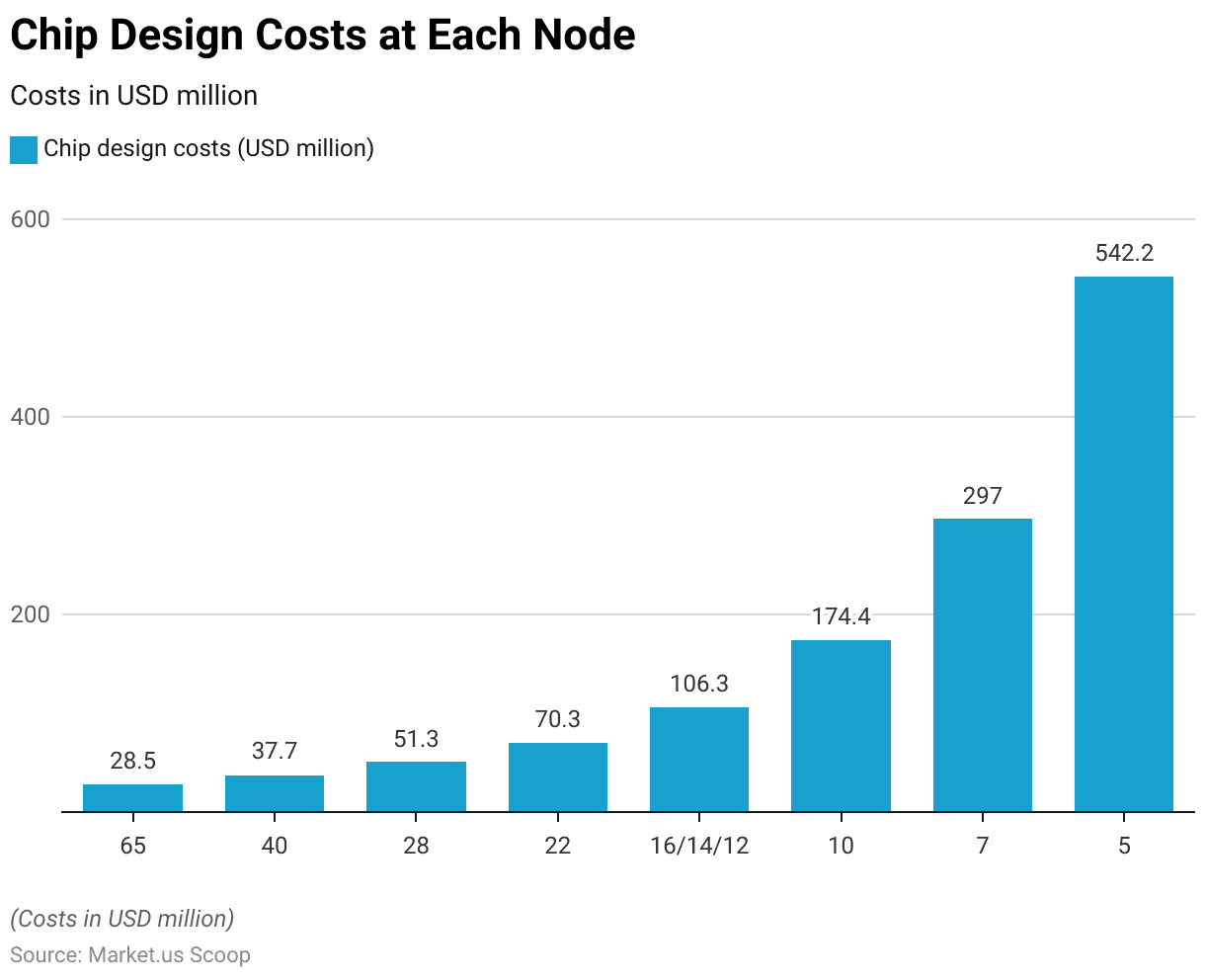
Chip Production and Operational Costs Across Different Nodes
- The production and operational costs of semiconductor chips have evolved significantly across different process nodes from 2004 to 2020.
- In 2004, with the 90nm node, the total production cost per chip was $3,877, with the foundry sale price to fabless firms at $2,433 and design costs of $630. As the process nodes shrank, the costs of manufacturing chips decreased substantially.
- By 2006, the 65nm node reduced total production costs to $2,298, with the foundry sale price at $1,428 and design costs of $392.
- By 2009, the 40nm node saw further reductions, with production costs dropping to $1,152 and assembly, test, and packaging costs falling to $239.
- The trend continued with the 28nm node in 2011, where the total production cost was $740, while the foundry sale price decreased to $453.
- In 2014, the 20nm node saw further reductions, with total production costs at $652 and design costs at $119.
- The 16/12nm nodes in 2015 had a production cost of $577, while the foundry sale price decreased to $331.
- By 2017, with the 10nm node, the total cost had fallen to $487, with assembly, test, and packaging costs at $92.
- In 2018, the 7nm node brought the total production cost to $421, with design costs of $110.
- Finally, by 2020, with the 5nm node, production costs were reduced to $426, while energy costs to operate the chip also saw a sharp decrease from $9,667 at 90nm to $194 at 5nm.
(Source: Center for Security and Emerging Technology – Georgetown University)
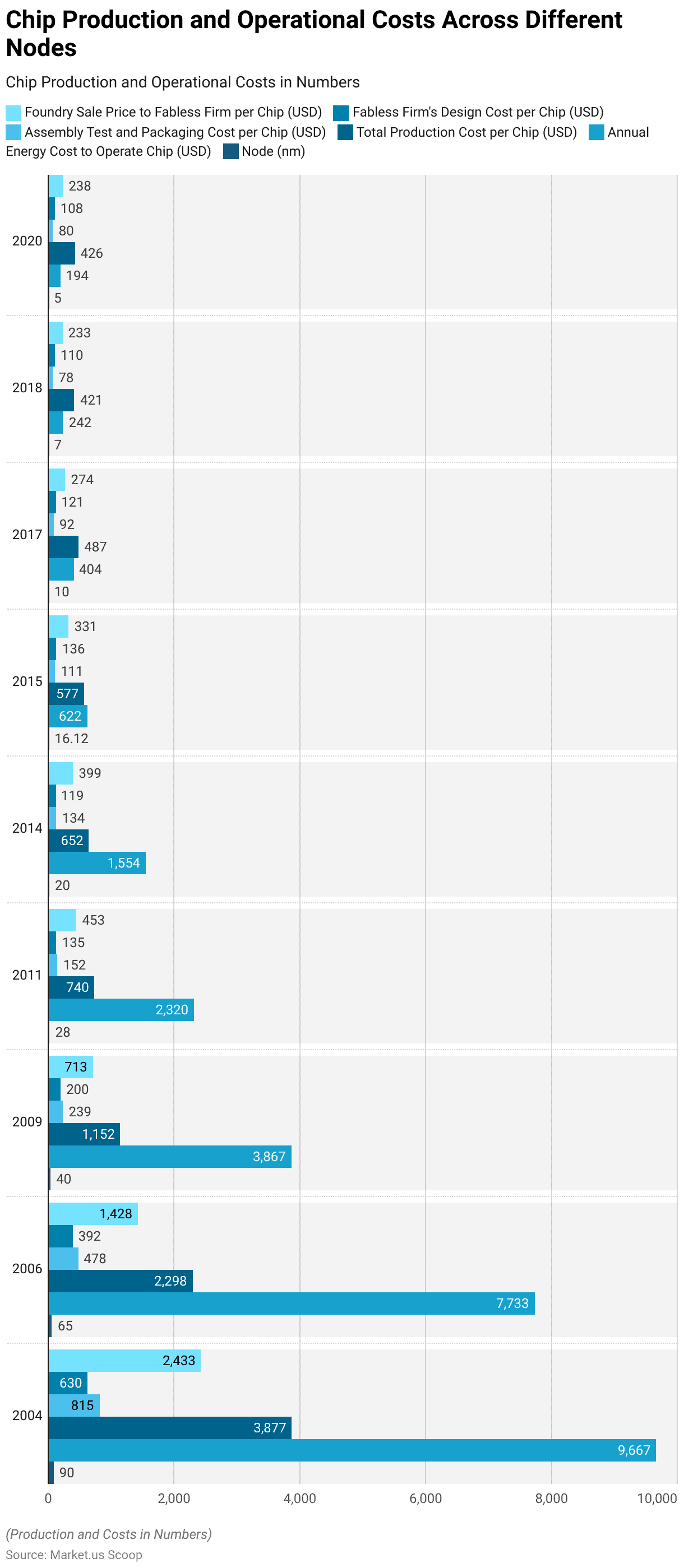
Leading U.S. and Chinese AI chips
- The leading U.S. and Chinese firms in the AI chips market represent a diverse range of chip types and manufacturing processes.
- In the GPU category, notable firms include U.S.-based AMD with its Radeon Instinct 7 nm chip fabricated by TSMC and Nvidia with its Tesla V100 (12 nm) also produced by TSMC.
- In China, Jingjia Micro offers the JM7200 (28 nm), though the fabrication details remain unspecified.
- The FPGA segment is led by U.S. firms like Intel, with its Agilex (10 nm), and Xilinx, with the Virtex (16 nm) made by TSMC.
- Chinese companies such as Efinix produce the Trion (40 nm) via SMIC, while Shenzhen Pango’s Titan (40 nm) remains fabless.
- In the ASIC category, U.S. companies lead with Cerebras’s Wafer Scale Engine (16 nm) and Google’s TPU v3 (16/12 nm), both made by TSMC, alongside Intel’s Habana (16 nm), also produced by TSMC.
- Samsung fabricates Tesla’s FSD computer (10 nm).
- Chinese players in the ASIC field include Cambricon’s MLU100 (7 nm) and Huawei’s Ascend 910 (7 nm), both manufactured by TSMC.
- Horizon Robotics offers Journey 2 (28 nm), which TSMC produces, while Intellifusion’s NNP200 (22 nm) is fabless.
(Source: Center for Security and Emerging Technology – Georgetown University)
Studies Performed to Analyze AI Chip Efficiency and Speed
Recent
- AI chip efficiency and speed have been extensively benchmarked across various studies comparing different hardware platforms for Deep Neural Networks (DNNs).
- For example, the Harvard-1 study (2019) compared the Nvidia Tesla V100 GPU with the Intel Skylake CPU, focusing on training Fully Connected Neural Networks (FCNN) with a performance improvement ranging from 1x to 100x.
- In the same year, Google’s TPU v2/v3 ASIC was compared to the Tesla V100 GPU for training Convolutional Neural Networks (CNN), Recurrent Neural Networks (RNN), and FCNN, achieving efficiency improvements from 0.2x to 10x.
- The MLPerf (2019) benchmark compared Google’s TPU v3 with the Nvidia Tesla V100 GPU for various training tasks like ResNet and Transformer, showing efficiency gains of 0.8x to 1.2x.
- Graphcore’s IPU ASIC outperformed GPUs in training Transformer and Multi-Layer Perceptron (MLP) models by 1x to 26x, while for inference tasks, it showed up to 43x improvement.
- In other studies, Google’s TPU v1 ASIC outperformed Intel Haswell CPU by 196x in training and 50x in inference for tasks like MLP and CNN.
- Other comparisons, such as Stanford (2017), showed Nvidia Tesla K80 GPUs outperforming Intel Broadwell vCPUs by 2x to 12x in training ResNet and 5x to 3x in inference.
- For instance, Hong Kong Baptist University (2017) found Nvidia GTX 1080 GPUs outperforming Intel Xeon CPUs by a factor of 7x to 572x in training FCNN, CNN, RNN, and ResNet.
- Similarly, the Harvard-2 study (2016) reported performance improvements of up to 1,700x for CNN and RNN training on Nvidia GeForce GTX 960 GPUs versus Intel Skylake CPUs.
Less Recent
- Bosch (2016) and Stanford/Nvidia (2016) observed Nvidia GPUs outperforming Intel CPUs for both training and inference tasks by up to 29x and 16x, respectively.
- The EIE ASIC demonstrated an impressive 1,052x improvement over the Nvidia GeForce Titan X GPU in training, with a 4x advantage in inference.
- Other studies, such as Rice (2016), compared Nvidia Jetson TK1 GPUs with CPUs, showing 4x improvements in inference, while Texas State (2016) found Nvidia Titan X GPUs outperforming Intel Xeon CPUs by 12x in training CNN.
- UCSB/CAS/Cambricon (2016) demonstrated that the Cambricon-ACC ASIC outperformed Nvidia K40M GPUs by 131x in training and 3x in inference for a variety of DNN tasks.
- Finally, Michigan-1 (2015) highlighted the Nvidia GTX 770 GPU achieving 7x to 25x faster inference than the Intel Haswell CPU for general DNN tasks.
- These studies collectively highlight the significant efficiency and speed improvements that AI chips, especially GPUs and ASICs, can provide over traditional CPUs in DNN training and inference tasks.
(Source: Center for Security and Emerging Technology – Georgetown University)
Sales Price of Chips
- The calculation of foundry sale prices per chip in 2020 by node size involves multiple factors such as capital investment, depreciation, and production costs.
- For each node size, the capital investment per wafer processed per year increases progressively from $4,649 for the 90 nm node to $16,746 for the 5 nm node.
- Net capital depreciation at the start of 2020 is applied at a rate of 65% for most nodes, except for the 7 nm and 5 nm nodes, which have depreciation rates of 55.1% and 0%, respectively.
- The remaining undepreciated capital per wafer processed at the start of 2020 varies, with the 90 nm node at $1,627 and the 5 nm node at $16,746.
- Capital consumed per wafer processed in 2020, which accounts for the depreciation and other factors, ranges from $411 for the 90 nm node to $4,235 for the 5 nm node.
- Additionally, other costs and markup per wafer, including operational expenses, also increase with smaller node sizes, from $1,293 for the 90 nm node to $12,753 for the 5 nm node.
- The foundry sale price per wafer follows a similar trend, ranging from $1,650 for the 90 nm node to $16,988 for the 5 nm node.
- Correspondingly, the foundry sale price per chip, which includes the total wafer cost distributed among the number of chips produced, decreases as the node size shrinks, from $2,433 for the 90 nm node to $238 for the 5 nm node.
(Source: Center for Security and Emerging Technology – Georgetown University)
Key Investment Statistics
Artificial Intelligence (AI) Investment Growth
- Between 2015 and 2025, global investments in artificial intelligence (AI) have seen significant growth.
- In 2015, worldwide AI investments stood at $10.27 billion, with the United States accounting for $6.67 billion and China for $2.07 billion.
- By 2016, global investments had risen to $17.76 billion, driven largely by increases in both the U.S. ($8.92 billion) and China ($3.92 billion).
- The trend continued upward, reaching $25.53 billion in 2017, with the U.S. and China investing $12.49 billion and $6.64 billion, respectively.
- In 2018, global AI investment surged to $38.05 billion, while the U.S. and China increased their investments to $17.41 billion and $11.05 billion, respectively.
- By 2019, global investment rose to $42.12 billion, with the U.S. and China investing $22.94 billion and $13.51 billion, respectively.
- The global AI investment reached $48.16 billion in 2020, with the U.S. investing $28.17 billion and China $10.28 billion.
- In 2021, there was a dramatic spike, with global investment reaching $93.54 billion, driven by investments from the U.S. ($52.88 billion) and China ($17.21 billion).
- However, in 2022, global investment slightly decreased to $91.9 billion, with the U.S. contributing $47.4 billion and China investing $20.63 billion.
- By 2023, global AI investment had increased again to $110.19 billion, with the U.S. contributing $56.83 billion and China $24.74 billion.
- The upward trend is expected to continue, with global investment projected to reach $132.12 billion in 2024, with the U.S. and China investing $68.14 billion and $29.66 billion, respectively.
- By 2025, global AI investments are expected to reach $158.41 billion, with the U.S. contributing $81.7 billion and China $35.57 billion.
(Source: Statista)
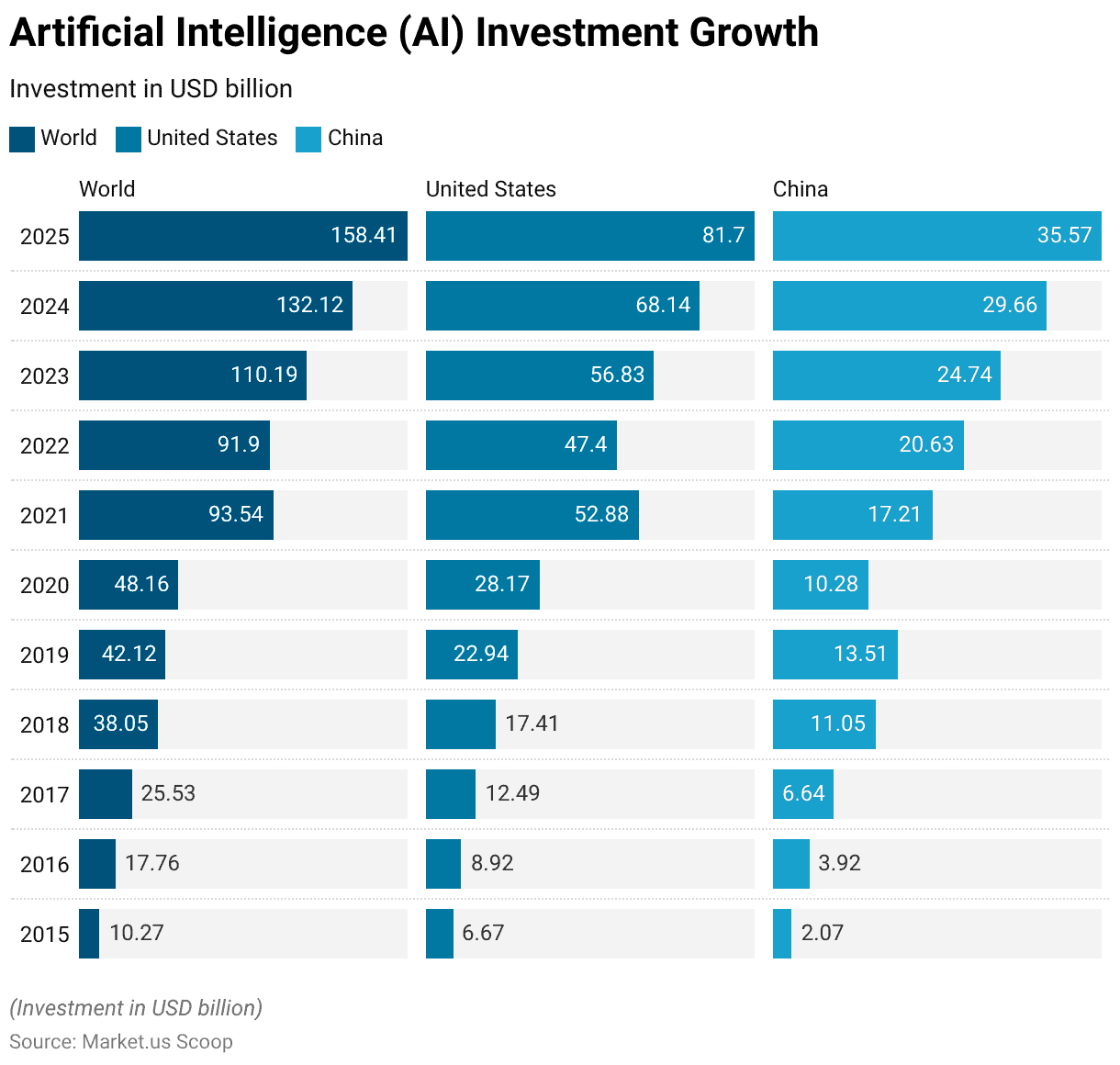
Global Private Investments in AI – By Region
- From 2013 to 2023, private investments in artificial intelligence (AI) worldwide were led by the United States, which received a total of USD 335.24 billion.
- China followed with USD 103.65 billion in AI investments.
- Other significant contributors included the United Kingdom with USD 22.25 billion, Israel at USD 12.83 billion, and Canada with USD 10.56 billion.
- Germany and India also saw considerable investments, with totals of USD 10.35 billion and USD 9.85 billion, respectively.
- France received USD 8.31 billion, while South Korea and Singapore saw USD 7.25 billion and USD 6.25 billion in investments, respectively.
- Japan’s AI sector attracted USD 4.81 billion, followed by Australia with USD 3.4 billion, Switzerland at USD 3.28 billion, and Hong Kong with USD 3.15 billion.
- Sweden rounded out the list with USD 2.88 billion in private AI investments.
- These figures highlight the global distribution of AI investments, with the United States holding a dominant share.
(Source: Statista)
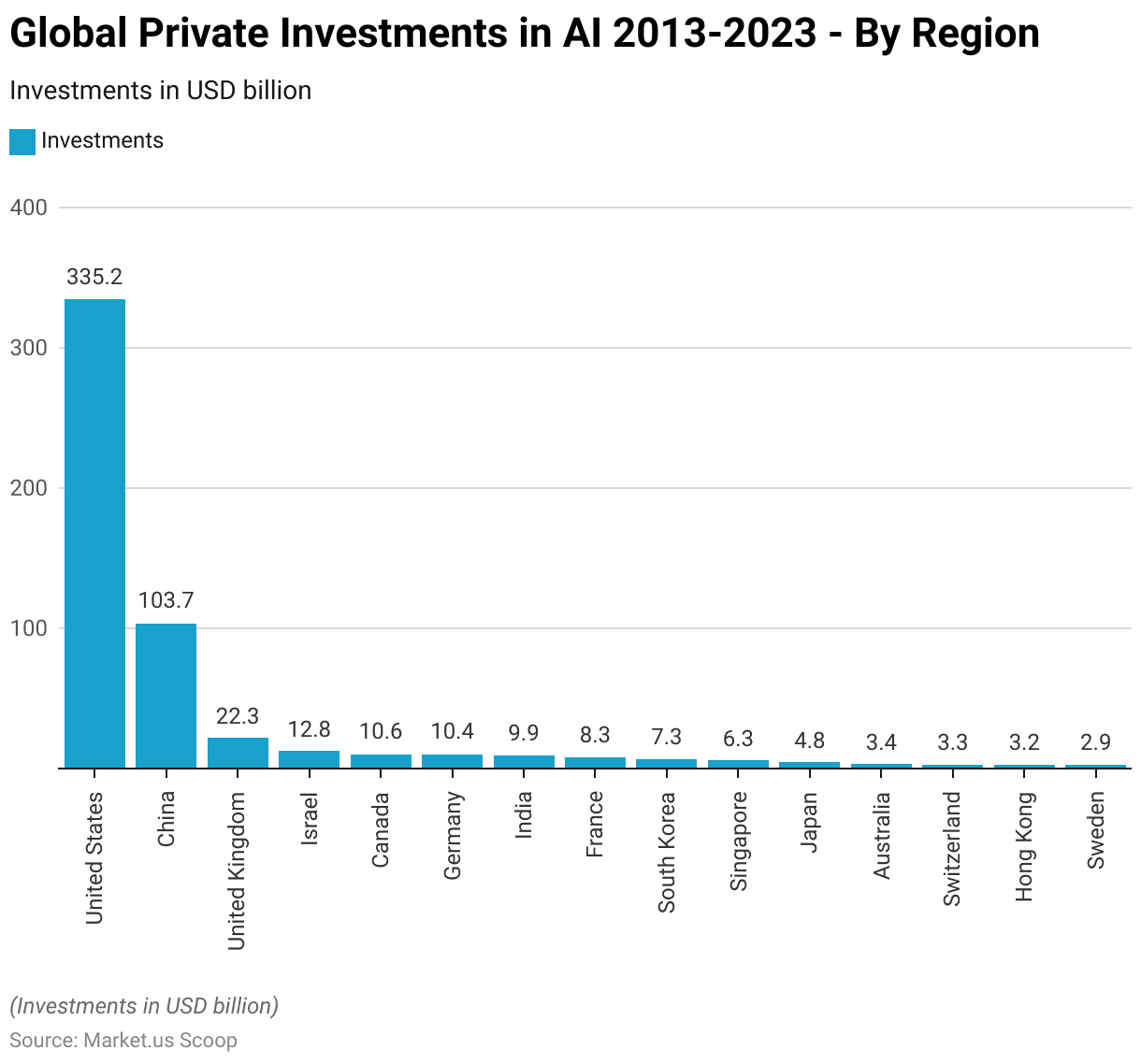
AI Startup Company Funding Worldwide
- From 2020 to 2023, funding for artificial intelligence (AI) startup companies worldwide showed notable fluctuations every quarter.
- In 2020, the funding remained relatively stable, with amounts ranging from USD 7.1 billion in Q1 and Q2 to a significant increase of USD 11.7 billion in Q4.
- The following year, 2021, saw a peak in funding, reaching USD 19.6 billion in Q1, followed by USD 17.8 billion in Q2, USD 18.8 billion in Q3, and USD 18.4 billion in Q4.
- In 2022, however, the funding began to decline, with Q1 seeing USD 16 billion, Q2 at USD 13.1 billion, and Q3 dropping further to USD 8.5 billion.
- Q4 2022 saw a slight recovery, with funding reaching USD 9.7 billion.
- In 2023, the funding continued to fluctuate with USD 15.9 billion in Q1, USD 10 billion in Q2, USD 9.5 billion in Q3, and a sharp decline to USD 7.1 billion in Q4.
- Overall, while AI startup funding was strong through much of 2021, the years following have seen a downward trend in investments.
(Source: Statista)
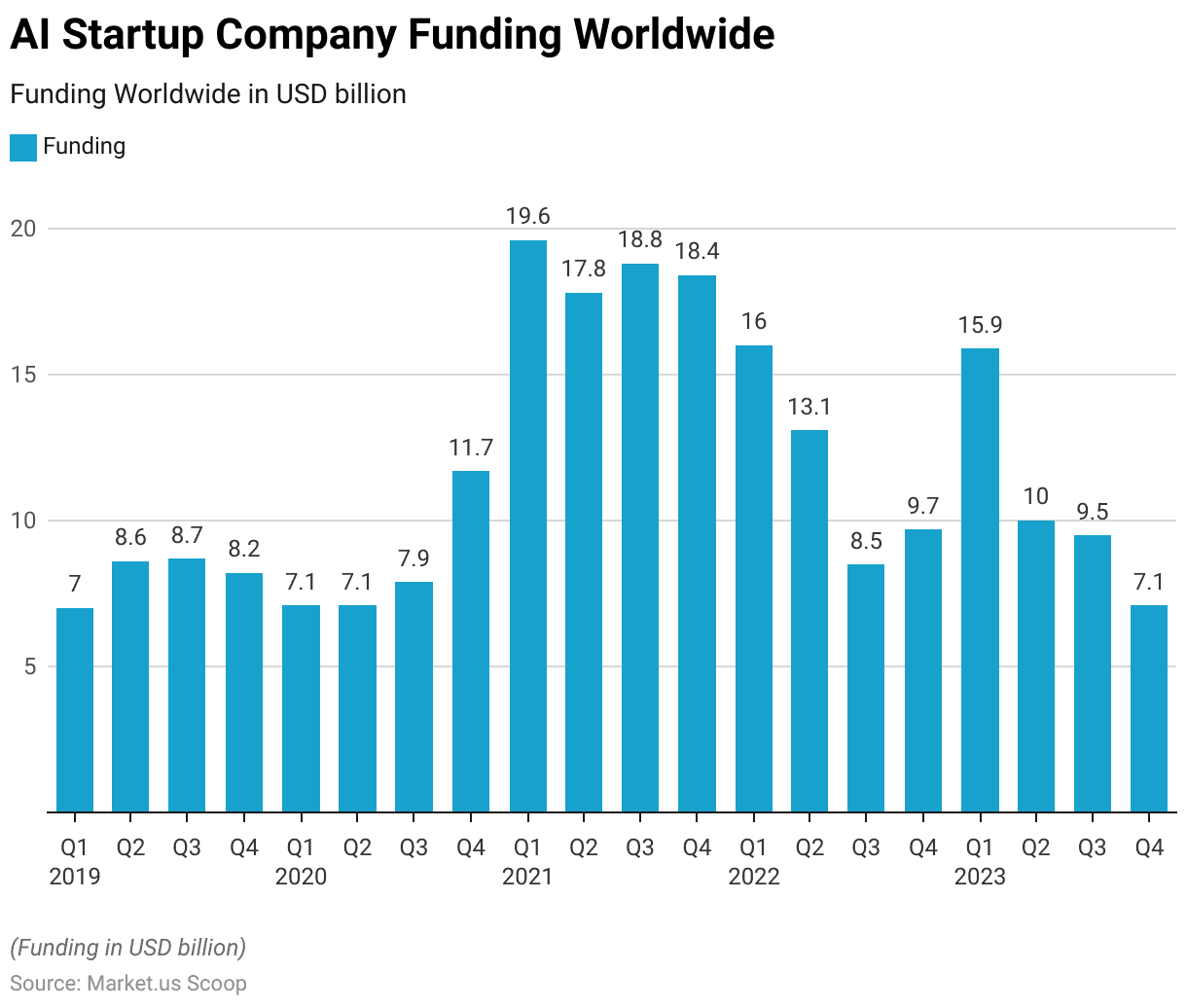
Most Funded Artificial Intelligence (AI) Chip Startup Companies Worldwide
- In 2021, several artificial intelligence (AI) chip startup companies attracted significant funding.
- SambaNova Systems led the list with a remarkable $1.14 billion in funding.
- Graphcore followed with $682 million, while Groq secured $362.28 million.
- Wave Computing raised $203.31 million, and Mythic received $155.15 million.
- These companies represent some of the most heavily funded startups in the AI chip sector, highlighting the growing interest and investment in AI hardware technologies.
(Source: Statista)
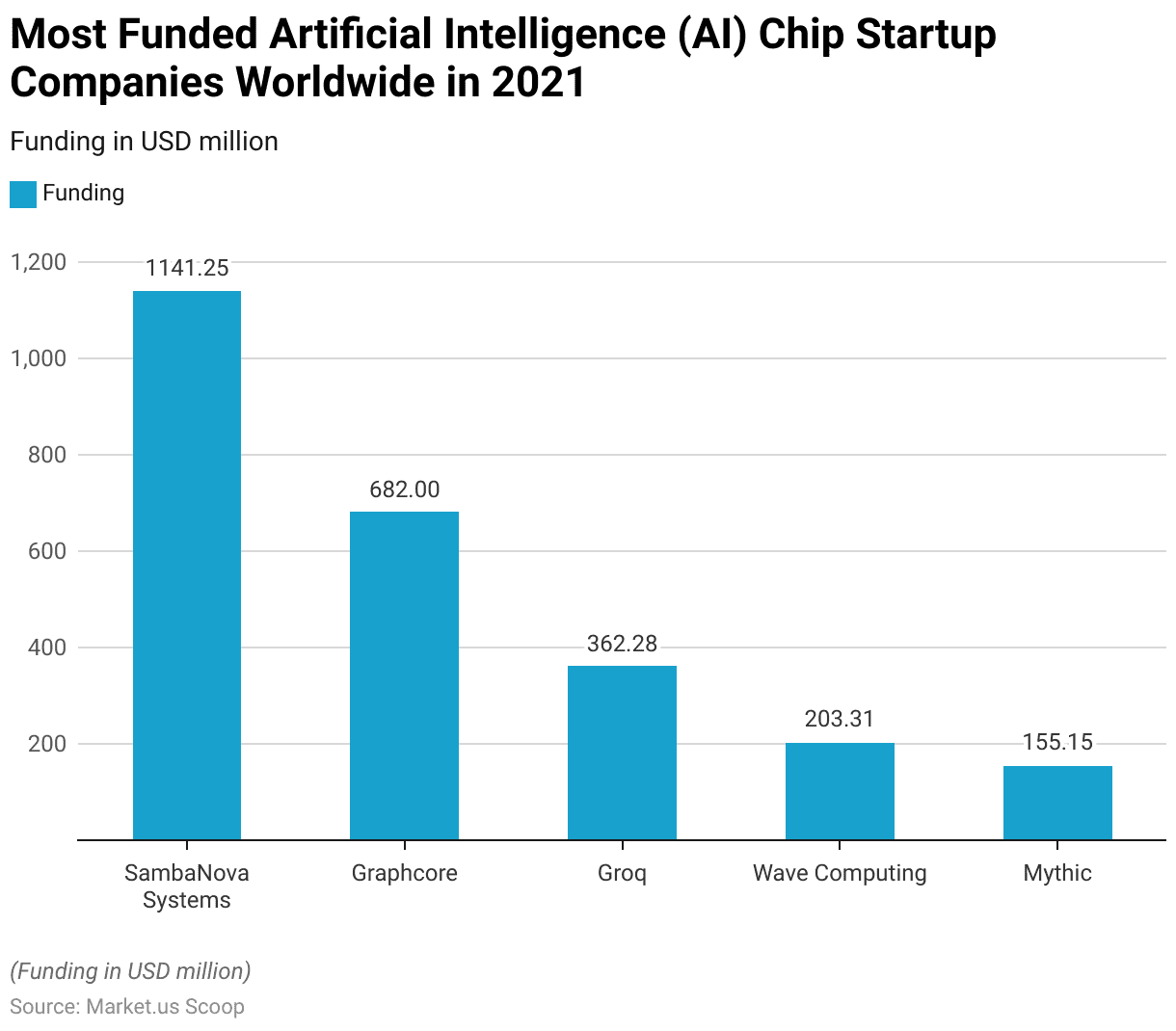
AI Global Return on Investment
- From 2015 to 2019, the return on investment (ROI) for AI deployments globally, based on respondents’ perceptions, showed a significant shift over the years.
- In 2015, 14% of respondents reported returns far exceeding expectations, while 40% said they experienced more than expected ROI, and 36% saw returns as expected.
- However, by 2016, the percentage of those reporting returns far exceeding expectations dropped to 8%, with 42% indicating more than expected ROI and 48% experiencing returns as expected.
- In 2017, only 2% of respondents saw returns far beyond expectations, while the majority (48%) reported returns as expected, with another 48% claiming returns were more than expected.
- By 2018, the trend continued, with just 1% reporting far better returns, 32% reporting more than expected, and 63% experiencing the expected ROI, with only 4% reporting less than expected returns.
- By 2019, the percentage of respondents seeing returns far better than expected had dropped to 1%, and only 12% reported returns more than expected, while a significant 82% saw returns as expected, and 5% experienced less than expected returns.
- This shift reflects a growing trend toward AI projects delivering returns as anticipated rather than exceeding expectations.
(Source: Statista)
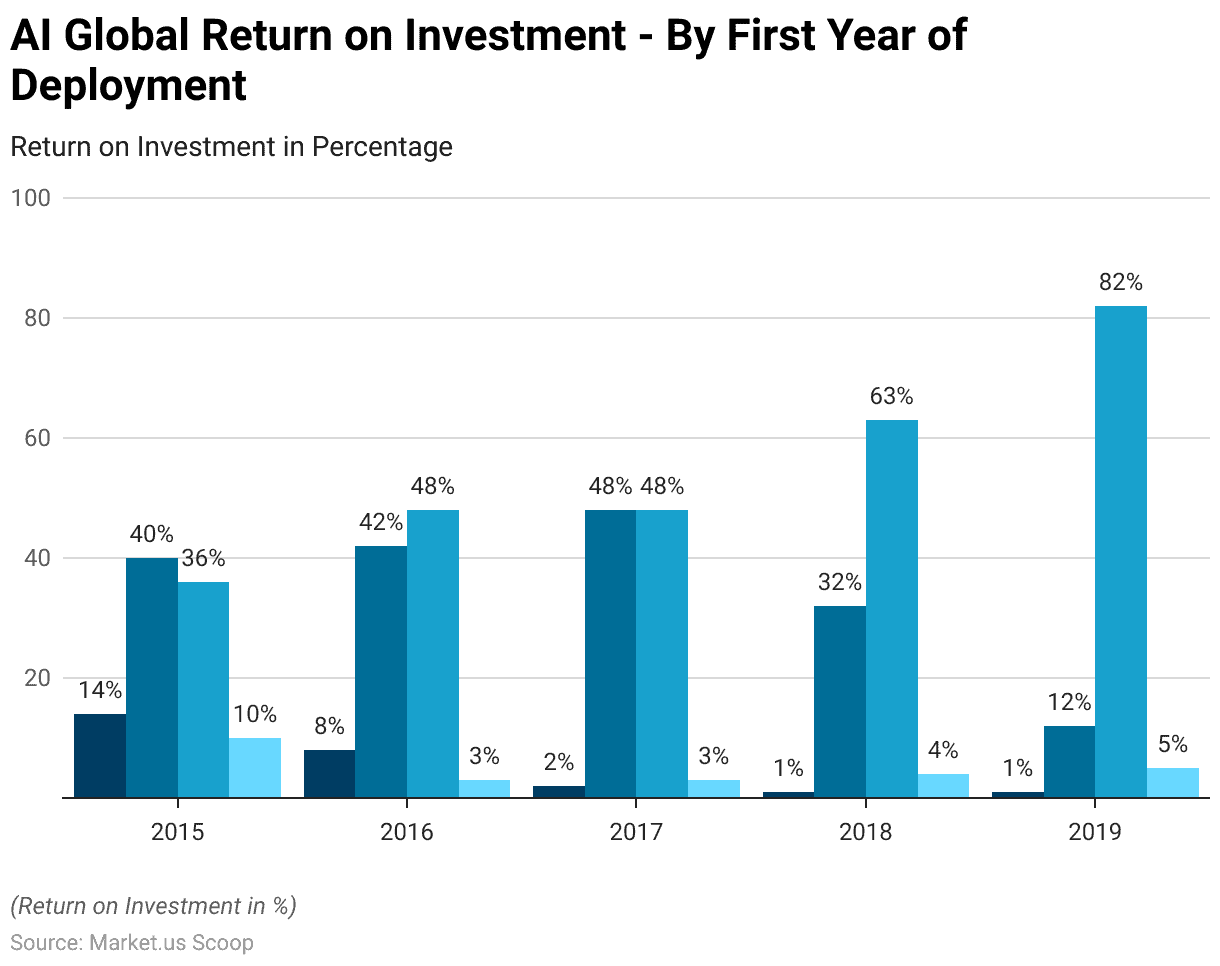
Innovations and Developments in AI Chipsets
- In 2024, the field of AI chipsets is witnessing remarkable innovations, largely driven by the escalating demands for more efficient and powerful AI applications across various industries.
- Companies like Nvidia and Graphcore are at the forefront of this technological advancement.
- Nvidia’s introduction of the Blackwell GPU and its NVLink interconnect technology showcases significant enhancements in processing speeds and energy efficiency, which is crucial for applications in areas such as the healthcare and automotive sectors.
- For instance, Nvidia’s technology has been instrumental in improving medical imaging processes and expediting the development cycles for electric vehicles by major automotive manufacturers.
- Graphcore has also made significant strides with its Colossus Mk2 IPU, designed to enhance the efficiency of AI and machine learning tasks through its advanced architecture that supports massive parallel processing. This innovation is particularly beneficial for complex AI research and the development of next-generation AI applications.
- Additionally, AWS is making its mark with Trainium, which optimizes AI model training across various applications and is fully integrated within AWS’s extensive cloud infrastructure, thereby providing a robust platform for AI deployment and training.
- Princeton University’s research, supported by DARPA, is redefining AI chip technology by focusing on creating compact, energy-efficient chips that can operate powerful AI systems with significantly less energy consumption.
- This breakthrough is expected to enable the deployment of AI technologies in more dynamic environments, from mobile devices to larger infrastructure.
- Overall, the innovations in AI chipsets are poised to transform a broad spectrum of sectors by delivering more efficient, specialized, and energy-conscious solutions that align with the evolving needs of both current and emerging AI applications.
(Sources: RedSwitches, Quantain Intelligence, Princeton Engineering)
Regulations for AI Chipsets
- In the evolving landscape of AI chipset regulations, countries have adopted varied approaches to ensure compliance and foster innovation while addressing ethical concerns.
- The United States has implemented stringent export controls, particularly affecting trade with China, emphasizing restrictions on the sale and distribution of high-end AI chips used in advanced computing applications.
- Concurrently, the EU‘s AI Act represents a comprehensive approach, setting stringent standards for AI systems deemed high-risk, thereby establishing a regulatory framework that balances innovation with user safety.
- China has been proactive with its regulations focused on generative AI applications, emphasizing control over AI-powered recommendation systems and content generation, ensuring compliance with societal values and intellectual property rights.
- This reflects a broader, globally observed trend where nations are increasingly amending existing laws or introducing new legislation tailored to the unique challenges posed by AI technologies.
- These range from transparency in AI operations to more specific aspects like data protection and the ethical use of AI, as seen in countries like Canada and Switzerland, which also emphasize aligning AI practices with human rights and safety standards.
- Overall, as AI technologies permeate various sectors, these regulatory measures illustrate a global shift towards a more regulated digital environment where the focus is equally distributed between fostering technological advancements and safeguarding fundamental ethical standards.
(Sources: The National Law Review, WIRED, Tech Monitor, CIRSD, Fairly AI, Legal Nodes)
Recent Developments
Acquisitions and Mergers:
- NVIDIA Acquires Arm Holdings (2022): NVIDIA’s acquisition of Arm Holdings for $40 billion, announced in 2020 and completed in 2022, significantly boosted its position in the AI chipset space. Arm’s chip architecture is widely used in mobile devices, and the acquisition is expected to enable NVIDIA to expand its AI and data center presence by integrating Arm’s power-efficient chip designs.
- Qualcomm Acquires Nuvia (2021): Qualcomm acquired AI startup Nuvia for $1.4 billion in a strategic move to enhance its chipset offerings, particularly for AI processing. This acquisition will enable Qualcomm to develop more powerful, energy-efficient chips tailored for AI workloads across various industries, including automotive and mobile devices.
Product Launches:
- Intel’s Habana Gaudi2 AI Chipset (2023): In 2023, Intel launched its Habana Gaudi2 AI chipset, designed specifically for deep learning and AI workloads. Gaudi2 is optimized for high-performance training of AI models and promises up to 20x higher performance compared to previous solutions. Intel’s investment in AI infrastructure is expected to enhance its position in the rapidly growing AI hardware market, which is projected to reach $45.8 billion by 2027.
- Apple’s M2 AI Chipset (2024): Apple introduced its new M2 AI chipset in 2024, designed to power its MacBook Pro and other high-performance devices. The M2 chip offers a significant upgrade in AI processing with 50% more processing power compared to its predecessor. Apple’s chip is focused on delivering faster machine learning (ML) and AI applications, particularly for creative professionals and enterprise environments.
Funding and Investments:
- Graphcore Raises $222 Million in Series E Funding (2022): Graphcore, a leading AI chipset startup, raised $222 million in Series E funding in 2022, bringing its total funding to over $710 million. The company specializes in creating chips optimized for machine learning and AI. This funding is expected to help Graphcore expand its product offerings, including the next-generation IPU (Intelligence Processing Unit) chips for AI workloads in cloud data centers and edge computing.
- SambaNova Systems Raises $676 Million (2021): SambaNova, an AI hardware startup, secured $676 million in a Series D funding round in 2021, bringing its total funding to over $1 billion. The company focuses on developing AI chips and systems designed for high-performance computing and deep learning tasks. SambaNova’s AI chipsets are expected to play a crucial role in accelerating AI applications across industries like healthcare, automotive, and finance.
Regulatory Developments:
- China’s Investment in AI Hardware: In 2023, the Chinese government unveiled new policies to accelerate the development of AI technologies, including dedicated funding for AI chip startups. As part of its strategy to become a global leader in AI by 2030, China has committed to investing heavily in AI chip development and has supported domestic firms like Huawei and SMIC in advancing AI hardware research and production.
Conclusion
Artificial Intelligence Chipset Statistics – The AI chipset market has seen significant growth, driven by the rising demand for high-performance computing in sectors like healthcare, automotive, and finance.
Key players such as NVIDIA, Intel, and AMD are leading innovations in AI hardware, focusing on more powerful and energy-efficient chipsets.
While the market faces challenges like high manufacturing costs and supply chain issues, the increasing adoption of AI technologies and the growth of AI-driven automation are expected to fuel further investment and development in AI chipsets, with strong demand anticipated in the coming years.
FAQs
AI chipsets are specialized hardware components designed to accelerate artificial intelligence tasks, such as machine learning, deep learning, and data analytics. They include processors like GPUs (Graphics Processing Units), TPUs (Tensor Processing Units), and other AI-specific chips that optimize the performance of AI applications.
AI chipsets are critical for enhancing the speed and efficiency of AI algorithms, enabling real-time processing of large data sets. They are essential for applications such as autonomous vehicles, speech recognition, facial recognition, and AI-driven automation across various industries.
The most common types of AI chipsets are GPUs, TPUs, and FPGAs (Field-Programmable Gate Arrays). GPUs, which are used for parallel processing, are widely used in AI workloads, while TPUs are specifically designed by Google for deep learning tasks. FPGAs offer customizable hardware for specific applications.
Leading companies in the AI chipset market include NVIDIA, Intel, AMD, Google (with its TPUs), and Apple. These companies are at the forefront of developing powerful AI hardware solutions for various sectors.
The main challenges include high manufacturing costs, ongoing advancements in semiconductor technology, and supply chain constraints. Additionally, optimizing AI chipsets for different use cases, such as edge computing and autonomous systems, requires continuous innovation.
Discuss your needs with our analyst
Please share your requirements with more details so our analyst can check if they can solve your problem(s)
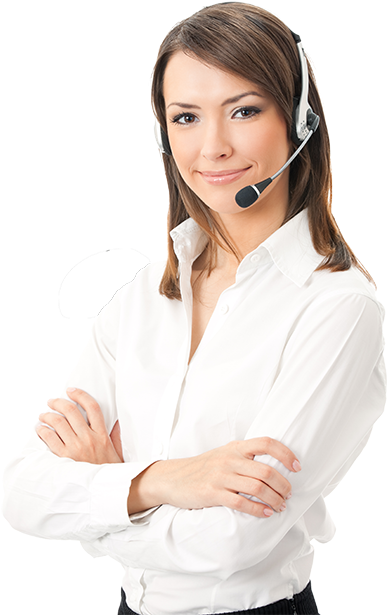