Table of Contents
Market Overview
According to research conducted by Market.us, the Generative AI in Material Science Market is projected to grow significantly over the next decade. The market size is expected to reach around USD 11.7 billion by 2034, a sharp increase from USD 1.1 billion in 2024. This impressive growth represents a compound annual growth rate (CAGR) of 26.4% during the forecast period from 2025 to 2034. In 2024, North America emerged as the leading region in this market, accounting for more than 36% of the total share, generating USD 0.3 billion in revenue.
Generative AI in material science is revolutionizing the field by enabling the rapid discovery and optimization of new materials. This technology utilizes advanced algorithms to simulate and predict material behaviors under various conditions, which significantly speeds up the process of material innovation. Generative AI models, such as those based on deep learning and adversarial networks, can generate new material structures, predict properties, and even suggest improvements to existing materials.
The integration of these AI tools allows researchers to explore a vast array of material combinations and configurations with precision and efficiency, leading to groundbreaking developments in industries ranging from automotive to aerospace. The market for generative AI in material science is expanding rapidly, driven by the technology’s ability to enhance the speed and reduce the costs of material development. This growth is spurred by increasing demand for high-performance materials across various sectors, including electronics, automotive, and renewable energy.
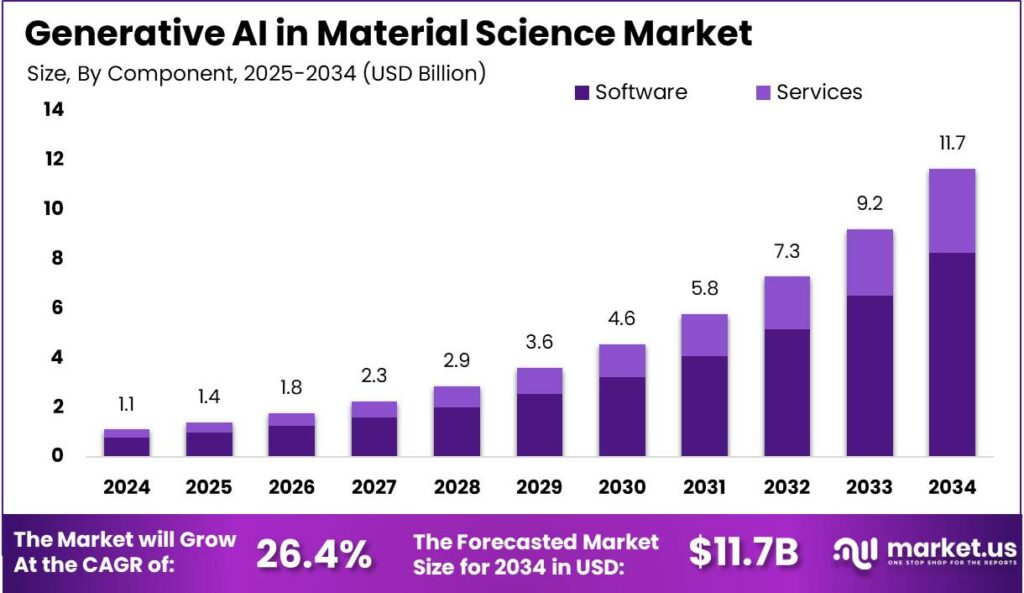
Companies and research institutions are investing heavily in AI capabilities to gain a competitive edge by developing innovative materials that meet specific industrial requirements more efficiently than ever before. The primary drivers of the generative AI in material science market include the pressing need for sustainable and efficient material solutions and the ongoing digital transformation in research and development processes.
According to Julien Florkin, the impact of AI on material science is transformative, with clear benefits across multiple key areas. Accelerated Discovery: Research indicates that AI can shorten the timeline for discovering new materials by up to 50%, compared to traditional approaches. Increased Precision: Predictive models powered by AI are achieving accuracy rates of over 90% in forecasting material properties, ensuring more reliable outcomes. Cost Reduction: The integration of AI into material science workflows has resulted in cost savings of around 30%, driven by fewer experimental failures and more efficient processes.
The ability of generative AI to reduce time-to-market for new materials, lower R&D costs, and enhance material properties to meet specific needs plays a crucial role in its adoption across industries. There is a significant demand for generative AI in sectors that rely on advanced materials, such as renewable energy, consumer electronics, and automotive industries. These sectors require materials with customized properties like enhanced conductivity, mechanical strength, and thermal stability.
Technological advancements in AI algorithms and computing power have dramatically improved the capabilities of generative AI in material science. Improved data analytics, machine learning models, and the integration of AI with robotic automation are pivotal in advancing material discovery and development. These technologies enable the simulation and testing of thousands of materials configurations, leading to faster and more accurate selection of optimal materials.
Incorporating generative AI into material science processes offers substantial business benefits, including enhanced innovation capabilities, improved speed of research and development, and significant cost reductions. Companies utilizing AI in material development report substantial decreases in time and financial investment needed for R&D, along with increased rates of innovation and the ability to quickly adapt to market needs.
Key Takeaways
- The Generative AI in Material Science Market is set to witness remarkable growth, reaching an estimated value of USD 11.7 billion by 2034, up from USD 1.1 billion in 2024. This indicates a strong compound annual growth rate (CAGR) of 26.4% during the forecast period of 2025 to 2034.
- In 2024, North America emerged as the leading region, contributing over 36% of the market share with revenue of approximately USD 0.3 billion. This dominance is driven by the presence of top technology companies and increased funding for research and development in the field.
- The Software Segment led the market, accounting for more than 71% of the total revenue in 2024. The widespread use of AI-driven tools for simulations, predictive modeling, and data analysis in material science is a key factor behind this segment’s growth.
- Within the market, the Material Discovery Segment represented over 40% of the market share in 2024. Generative AI is transforming material discovery by enabling faster identification of innovative materials with unique properties, helping industries accelerate innovation.
- The Aerospace and Defense sector stood out as the largest application area, contributing over 30% of the market share in 2024. The growing demand for lightweight, durable, and high-performance materials in aerospace technology has significantly boosted the adoption of generative AI solutions in this sector.
Regional Analysis
In 2024, North America solidified its position as a leader in the generative AI in material science market, capturing over 36% of the global share with revenues reaching approximately USD 0.3 billion. This dominance can be attributed to several key factors that uniquely position North America at the forefront of this innovative technology.
Firstly, North America is home to some of the world’s leading technology companies and research institutions which actively invest in AI and materials science. These entities often collaborate, creating a synergistic environment that fuels technological advancement and innovation. For instance, major U.S. tech firms and Canadian research universities have poured resources into developing and applying AI tools in materials research, pushing the boundaries of what’s possible in material discovery and optimization.
Secondly, the region benefits from robust government support and favorable policies that encourage research and development in advanced technologies. The U.S. government, for example, has launched several initiatives aimed at fostering innovation in AI and material sciences, providing grants and tax incentives to support these endeavors. This governmental backing not only secures financial resources but also establishes a framework within which researchers can operate more freely and innovatively.
Moreover, North America has a strong industrial base that demands the continuous development of new materials to enhance product performances and introduce innovations in various sectors such as aerospace, automotive, and electronics. This demand drives the market for advanced material solutions, where generative AI plays a critical role by accelerating the development cycle and reducing costs associated with material innovations.
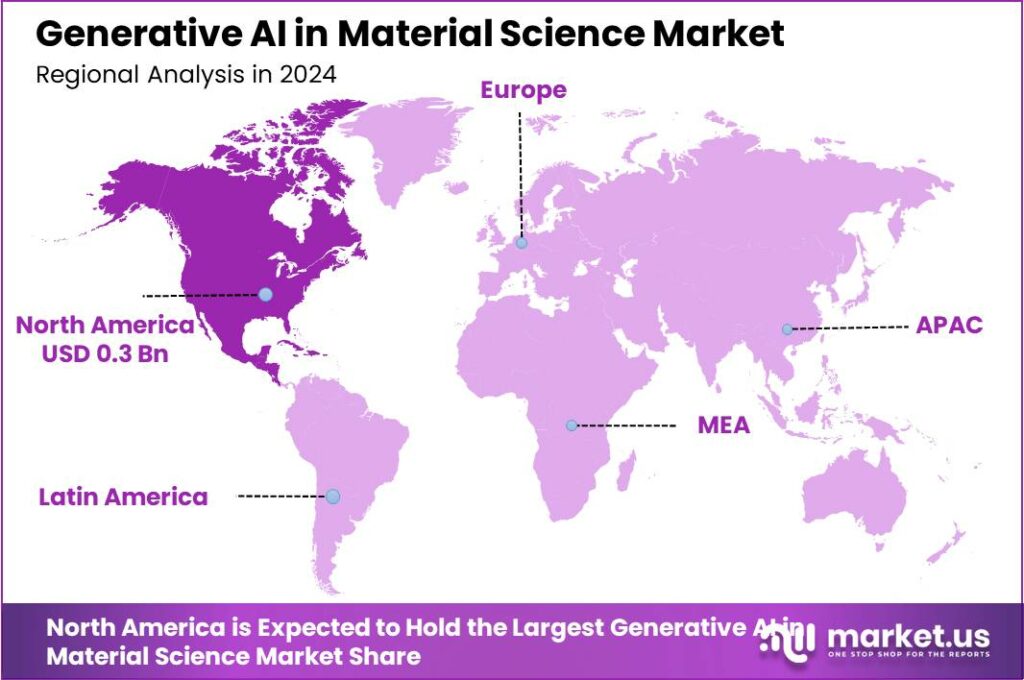
Report Segmentation
Component Analysis
In 2024, the Software segment held a dominant position in the Generative AI in Material Science market, capturing over 71% of the market share. This segment’s prominence is largely due to the pivotal role software plays in the operational frameworks of generative AI systems.
Software solutions are integral to creating, simulating, and analyzing material properties, which are crucial for accelerating innovation and reducing time-to-market across various industries such as automotive, aerospace, and electronics. The growth in this segment is propelled by the increasing sophistication of algorithms that enhance the ability to predict material behaviors under different conditions, making software an indispensable tool in material science research and development.
Application Analysis
The Material Discovery segment also saw significant dominance in the market, accounting for over 40% of the market share in 2024. Generative AI’s impact here is transformative, significantly accelerating the process of identifying new materials with unique properties. This application is critical in industries that demand high-performance materials such as pharmaceuticals, energy, and consumer electronics. The ability of generative AI to rapidly predict and simulate material properties using extensive data sets reduces the reliance on slower experimental methods, enhancing both the speed and precision of material discovery.
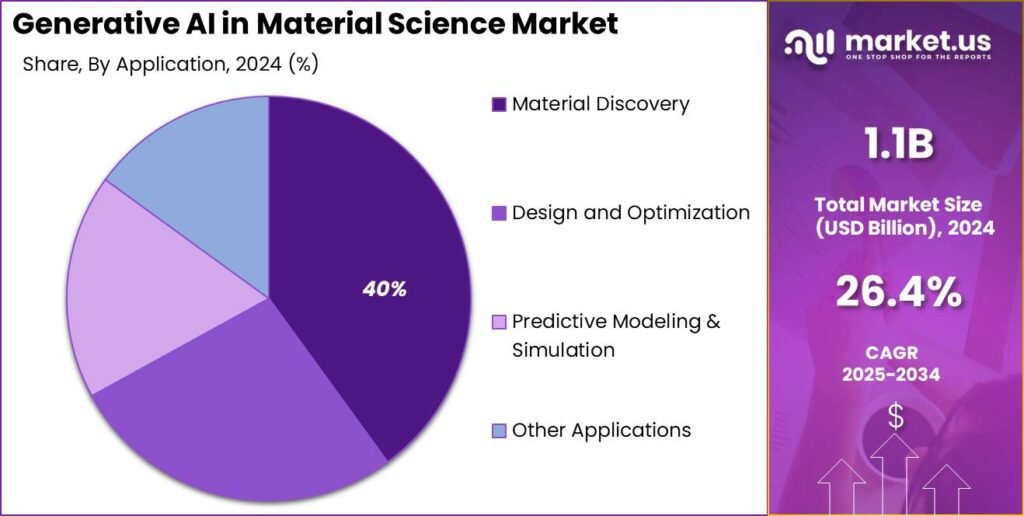
Industry Vertical Analysis
In the aerospace and defense sectors, generative AI has become particularly influential, with these industries accounting for over 30% of the market share. This segment benefits from generative AI due to the stringent demands for materials that must perform under extreme conditions while meeting high safety and durability standards.
Generative AI facilitates the development of new composites and materials that contribute to advancements in fuel efficiency, emissions reduction, and overall performance of aerospace components. The technology’s ability to rapidly prototype and validate material performance under varied conditions plays a crucial role in shortening development cycles and enhancing material efficacy.
Emerging Trends
- Material Property Prediction: Advanced AI models like DALL-E 3 and MatterGen are now capable of predicting the properties of hypothetical materials, significantly expediting the discovery and validation of new materials.
- AI-Driven Hypothesis Generation: Large language models are being used to formulate novel hypotheses in fields ranging from chemistry to environmental science, broadening the scope of scientific discovery.
- Robotic Automation: The integration of AI with robotics in labs allows for the rapid execution of numerous experiments, substantially reducing the development time for new materials.
- Customized Solutions through AI: Generative AI tools can create materials with specific desired properties, reducing the need for trial-and-error in materials design.
- Cross-Disciplinary Integration: AI tools now enable a single researcher to handle multifaceted materials science challenges that would have traditionally required a team of specialists.
Top Use Cases
- Accelerated Design Processes: AI models have reduced the time from concept to prototype, enabling faster iterations and innovations in material development.
- Enhanced Data Analysis: AI can sift through vast databases of chemical properties and experimental data to identify potential new materials or formulations.
- Optimization of Material Characteristics: Through models like GANs, AI is able to generate materials tailored for specific functional requirements.
- Energy Efficiency Improvements: AI is helping to develop materials that are not only effective but also more environmentally friendly and energy-efficient.
- Quality Control: AI is being integrated into the manufacturing processes to enhance quality control and compliance with regulatory standards.
Major Challenges
- Data Quality and Availability: The success of AI models heavily relies on the availability of high-quality, comprehensive datasets.
- Integration with Existing Systems: Merging AI into established industrial processes requires substantial changes to workflows and systems.
- Interpretability and Trust: There is a need for better tools to interpret AI decisions, especially in high-stakes areas like material safety.
- Cost of Implementation: While AI promises cost reductions over time, the initial setup and customization for specific industrial applications can be costly.
- Skill Gap: There is a growing need for professionals who are trained to work at the intersection of AI and material science.
Attractive Opportunities
- New Material Discovery: AI can identify materials with potentially groundbreaking properties, opening up new applications in industries like aerospace and biotechnology.
- Sustainability Initiatives: By optimizing material properties, AI contributes to the development of more sustainable and less resource-intensive products.
- Personalized Production: AI’s ability to tailor material properties at scale allows for more customized and efficient production lines.
- Simulation and Testing: AI-driven simulations can predict how materials will perform under various conditions, reducing the need for costly real-world testing.
- Educational and Training Programs: As AI reshapes material science, there is increasing value in educational programs that combine AI knowledge with traditional scientific training.
Conclusion
Generative AI in material science marks a transformative leap forward in the development and optimization of new materials, reshaping industries by offering enhanced efficiency, innovation, and sustainability. As businesses increasingly adopt these AI technologies, they stand to gain from reduced R&D cycles and costs, more precise material customization, and the accelerated discovery of novel materials tailored to modern technological demands.
This evolution not only drives economic growth but also contributes to a more sustainable approach to material utilization and waste reduction. Moving forward, as the technology matures and integrates further with industry practices, the impact of generative AI on material science is poised to expand, promising even greater advancements and efficiencies in material research and applications.
Discuss your needs with our analyst
Please share your requirements with more details so our analyst can check if they can solve your problem(s)
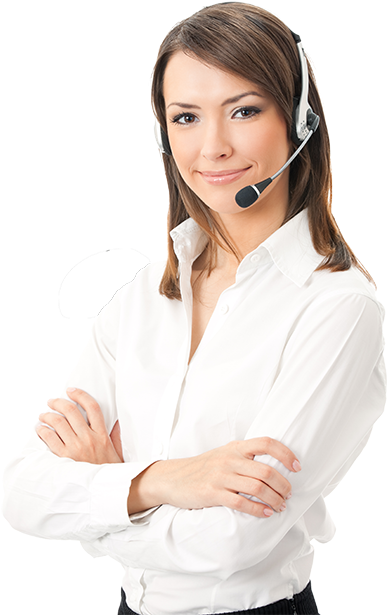