Table of Contents
Report Overview
As per Market.us report, The Global Natural Language Processing in Finance Market is set to reach an impressive USD 59.7 billion by 2033, a significant leap from its USD 5.43 billion valuation in 2023. This growth, driven by a compound annual growth rate (CAGR) of 27.10%, is expected to unfold between 2024 and 2033. In 2023, North America led the market, commanding over 36% of the total share and generating a revenue of USD 1.95 billion.
Natural Language Processing (NLP) in finance is a rapidly growing field that leverages advanced computational techniques to analyze, interpret, and extract valuable insights from vast amounts of unstructured textual data within the financial industry. NLP enables computers to understand and process human language, allowing financial institutions to efficiently manage data from a variety of sources, such as news articles, earnings reports, market sentiment, and social media.
The growth of Natural Language Processing (NLP) in the financial sector is driven by its ability to extract valuable insights from unstructured data like news, reports, and social media, which were previously analyzed manually. Advancements in AI and machine learning have enhanced NLP tools, enabling tasks such as sentiment analysis, risk management, and regulatory compliance, helping financial institutions stay ahead of market trends, make informed investment decisions, and improve customer service.
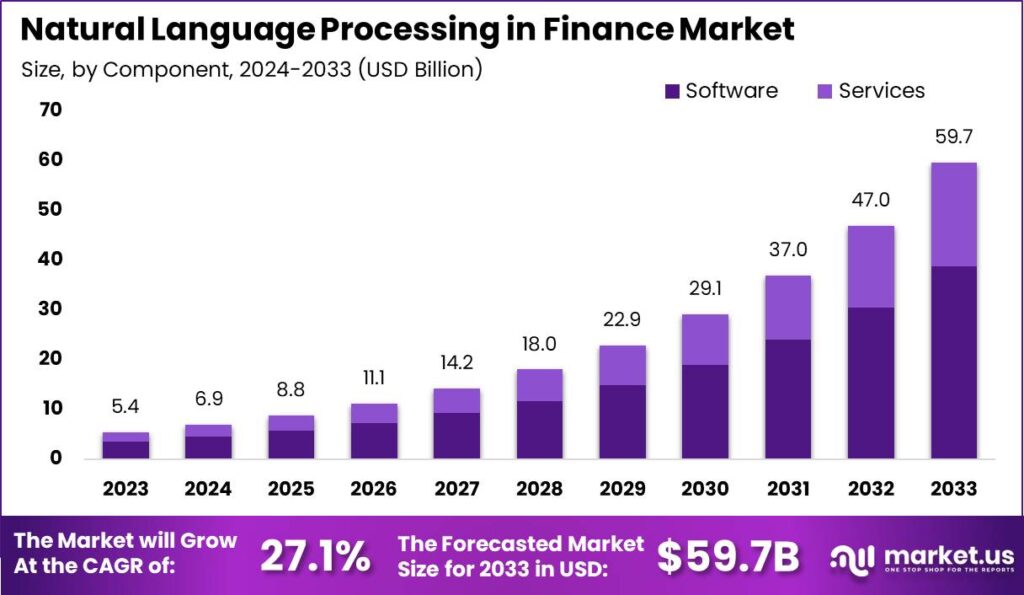
Key applications of NLP include evaluating credit risks by analyzing unstructured data, using techniques like sentiment analysis and named entity recognition to assess borrowers’ creditworthiness. It also automates regulatory document monitoring to ensure compliance and reduce manual effort. Additionally, NLP helps financial analysts extract actionable insights from large data sets, supporting more informed investment decisions.
Despite its advantages, NLP in finance faces challenges such as the need for large amounts of high-quality, current, and well-annotated data, which is often restricted by privacy concerns and data regulations. Additionally, the complexity of financial language, with its nuanced and context-dependent terminology, makes it difficult for NLP systems to accurately analyze. The rapidly changing nature of the financial sector also requires NLP tools to continuously adapt to new terms and market conditions.
Technological innovations in NLP, especially with advanced large language models like GPT-4, are revolutionizing the financial sector by providing more accurate and nuanced interpretations of financial language. Trained on diverse datasets, these models can handle a wide range of financial documents. Additionally, integrating NLP with technologies like blockchain and the Internet of Things (IoT) enhances NLP capabilities and opens up new possibilities for secure and efficient financial transactions.
The expansion of the NLP market in finance shows no signs of slowing down as technological advancements make NLP tools more accessible and effective. The rise of digital financial services in emerging markets presents new opportunities for deployment, while the increasing interconnectedness of the global finance landscape amplifies the need for multilingual NLP solutions. These solutions, capable of serving diverse markets, are broadening the scope and reach of NLP applications, further fueling growth in the sector.
Key Takeaways
- In 2023, the Software segment was the dominant player in the NLP in Finance Market, holding over 65% of the market share.
- The Sentiment Analysis segment also secured a significant position, capturing more than 31% of the market share.
- The Banks segment led the NLP in Finance Market, accounting for over 46% of the market share.
- North America emerged as the top region, commanding more than 36% of the market share, with total revenues reaching approximately USD 1.95 billion.
Impact Of AI
- Improved Customer Service with Chatbots and Virtual Assistants: AI-powered chatbots and virtual assistants are transforming customer interactions in financial institutions by understanding natural language queries and providing instant responses. This reduces wait times, improves user experience, and allows AI to assist with account inquiries, transaction processing, and personalized financial advice.
- Enhanced Sentiment Analysis for Market Insights: NLP, combined with AI, can process and analyze large volumes of news articles, social media posts, and financial reports to gauge market sentiment. Financial analysts and investors can use this AI-driven sentiment analysis to make better decisions by understanding market moods and predicting trends. For instance, analyzing Twitter posts and news headlines can help in predicting stock price movements.
- Efficient Fraud Detection and Risk Management: AI and NLP technologies are also used to identify patterns that signal potential fraudulent activity. By analyzing transaction records and communication patterns (such as emails or chat logs), AI systems can detect irregularities in real-time, alerting security teams before a problem escalates. This helps financial institutions manage risks better and protect customers from financial fraud.
- Streamlining Regulatory Compliance: AI-driven NLP systems help financial institutions navigate complex regulations by scanning and interpreting legal documents, filings, and contracts. This automation reduces human error, accelerates compliance checks, and ensures firms meet all regulatory requirements efficiently.
- Better Document Automation and Processing: AI-based NLP systems automate the extraction and processing of data from financial documents like contracts, invoices, and loan agreements, reducing manual effort and errors. This results in faster processing, improved accuracy, and more efficient resource use, such as extracting financial data for easier storage and analysis.
Regional Analysis
In 2023, North America emerged as the leading region in the Natural Language Processing (NLP) market for finance, securing over 36% of the global market share. This dominance is reflected in the region’s significant revenue generation, which amounted to approximately USD 1.95 billion. The region’s commanding position can be attributed to several key factors, including the presence of a large number of financial institutions, technology firms, and AI research hubs. North American financial services have been quick to adopt advanced NLP technologies, utilizing them for various purposes such as sentiment analysis, risk management, and regulatory compliance.
Moreover, the rapid advancements in artificial intelligence (AI) and machine learning (ML) technologies within the region have enabled financial firms to implement more sophisticated NLP solutions. These innovations have helped financial institutions in North America stay ahead of the curve in terms of analytics and decision-making. The widespread use of NLP in customer service applications, such as chatbots and virtual assistants, further contributes to the region’s substantial share.
Additionally, government and regulatory support for technology adoption, coupled with high investments in research and development, have played a pivotal role in driving the growth of NLP in the financial sector across the region. With its established infrastructure, robust financial markets, and continued push for digital transformation, North America is expected to maintain its leadership in the NLP in finance market for the foreseeable future, continuing to capitalize on emerging technologies to improve operational efficiency, customer experience, and compliance management.
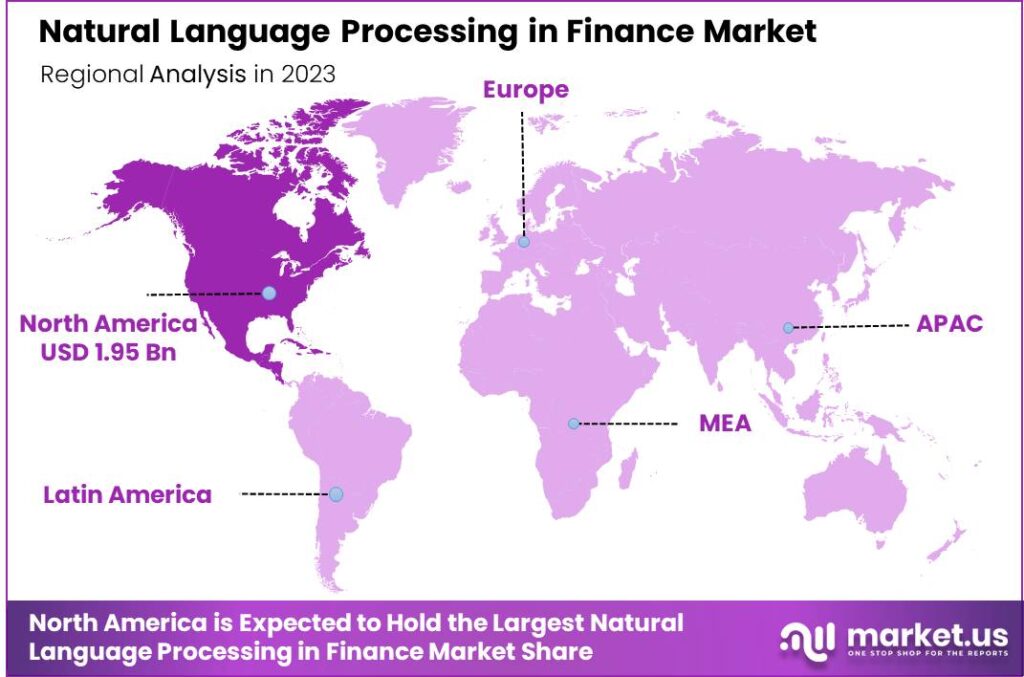
Market Segmentation
Component Analysis
In 2023, the Software segment emerged as the dominant player in the NLP in Finance market, holding over 65% of the market share. This dominance is primarily driven by the increasing adoption of artificial intelligence (AI) and machine learning (ML) technologies across financial institutions. NLP software solutions allow for better data processing and interpretation of unstructured financial data such as emails, reports, news articles, and social media.
The efficiency and accuracy provided by these software tools in automating tasks like risk assessment, fraud detection, and customer support have made them indispensable to financial institutions, contributing to their market leadership.
Application Analysis
The Sentiment Analysis segment captured more than 31% of the NLP in Finance market share in 2023, solidifying its position as a critical component. Sentiment analysis, which involves extracting and understanding emotions or opinions from textual data, has become essential for financial institutions to gauge market sentiment, assess public opinions on stock movements, and predict economic trends.
By leveraging NLP techniques, financial entities can quickly analyze vast amounts of unstructured data from social media, news, and financial reports to inform their decision-making processes. This capability has made sentiment analysis a significant tool in financial forecasting and strategy.
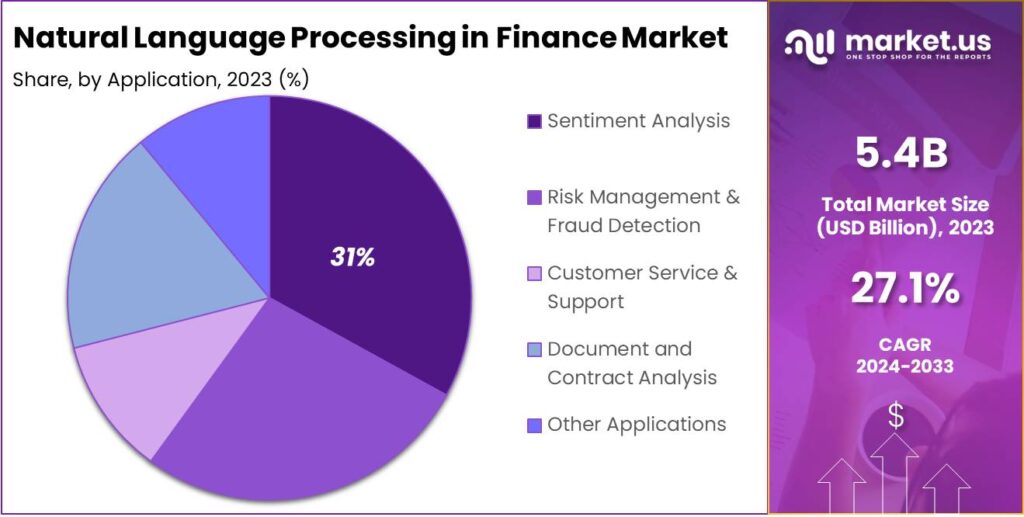
End-User Analysis
The Banks segment led the NLP in Finance market in 2023, accounting for over 46% of the total market share. Banks have been at the forefront of adopting NLP-driven technologies to streamline operations, enhance customer experiences, and improve decision-making.
Through NLP, banks are able to automate document processing, optimize chatbots for customer service, detect fraudulent activities through transaction monitoring, and support more efficient compliance monitoring. Additionally, NLP applications help in customer sentiment analysis, portfolio management, and risk mitigation, making them a key driver of market growth within the financial sector.
Top Use Cases
- Automated Customer Support: Banks and financial institutions are using chatbots and virtual assistants powered by NLP to provide 24/7 customer service. These systems can understand and respond to customer queries in natural language, offering solutions for account management, transactions, and other basic financial services.
- Financial Document Analysis: Processing financial reports, legal contracts, and regulatory filings can be time-consuming. NLP algorithms can read and interpret these documents quickly, extracting key data points such as revenue, profit margins, and compliance status, which helps in making informed investment decisions.
- Risk Management: Financial institutions use NLP to evaluate potential risks by analyzing public and private datasets, including news, blogs, and financial statements. By processing this large volume of unstructured data, NLP systems can identify emerging risks that could impact a company’s performance.
- Algorithmic Trading: NLP is also used in algorithmic trading to analyze market news, reports, and social media posts in real time. This helps trading algorithms make quicker, data-driven decisions, reacting to market shifts much faster than human traders could.
- Customer Insights and Personalization: NLP helps financial institutions understand customer needs by analyzing customer feedback, reviews, and survey responses. This allows banks to tailor their products and services to individual preferences, providing a more personalized experience for their clients.
Major Challenges
- Understanding Complex Financial Jargon: Financial documents often contain complex terms and industry-specific jargon that can be difficult for NLP systems to interpret accurately, leading to potential errors. Ensuring NLP systems fully understand specialized language, such as “collateralized mortgage obligations” or “derivative contracts,” is a significant challenge.
- Data Privacy and Security Concerns: The finance sector handles sensitive information, including personal, financial, and transaction data. When using NLP to process customer data (e.g., emails, chat logs, or contracts), there is a significant risk to privacy and security. Financial institutions must ensure that their NLP systems comply with strict data protection regulations, such as GDPR, while safeguarding sensitive customer information from potential breaches.
- Contextual Understanding of Financial Texts: One of the key difficulties in applying NLP in finance is ensuring that systems understand context in financial documents. In many cases, words or phrases might have multiple meanings depending on their context. For example, the term “short” could refer to “short selling” in a trading context or simply mean “brief” in everyday language. Misinterpreting these nuances can lead to faulty analysis and decisions.
- Data Quality and Inconsistencies: NLP systems require large amounts of data to be effective. In the financial industry, data quality is a common issue due to inconsistent formats, incomplete records, or noisy data (such as irrelevant information in customer feedback). If the data fed into NLP systems is not clean or structured properly, the system may produce inaccurate results, affecting financial analysis and decision-making.
- Adapting to Constantly Changing Financial Regulations: The financial industry’s evolving regulations require NLP systems to adapt continuously to stay compliant. Financial institutions must regularly update their models to interpret new laws and policies accurately, as failure to do so could result in compliance risks and legal consequences.
Market Opportunities for Key Players
- Automated Customer Support and Chatbots: To meet the demand for quicker responses, banks and financial institutions are using NLP-driven chatbots to automate customer service, handling queries on account balances, transaction histories, loan rates, and more, while reducing reliance on human agents. This shift not only reduces costs but also boosts customer satisfaction with 24/7 support, contributing to significant growth in the global chatbot market within banking.
- Sentiment Analysis for Investment Decisions: NLP can analyze large volumes of text from sources like news articles, earnings calls, financial reports, and social media to assess market sentiment, helping companies predict stock movements, identify opportunities, and spot risks. Financial firms are increasingly using NLP for competitive advantage in high-frequency trading and predictive analytics, and as data volume grows, NLP’s role in decision-making will continue to expand.
- Fraud Detection and Risk Management: Financial institutions are using NLP to detect fraud and improve risk management by analyzing unstructured text for unusual patterns. This real-time detection helps prevent losses and ensures compliance with stricter regulations, reducing fraudulent claims for banks and insurers.
- Regulatory Compliance and Document Processing: NLP automates the review and processing of financial documents, helping banks comply with regulations like contract analysis, AML screening, and KYC. This reduces errors, improves efficiency, and meets the growing demand for NLP-based solutions to minimize compliance risks.
- Personalized Financial Advice and Wealth Management: Financial advisors are using NLP-driven tools to improve their advisory services, by better understanding client needs and offering more precise investment strategies. With the rise of robo-advisors, this area is expected to grow significantly. NLP can power a new generation of wealth management solutions that can offer highly personalized services without the need for traditional human advisors.
Top Key Players in the Market
- Intel Corporation
- Nvidia Corporation
- Nuance Communications
- IBM Corporation
- Lexalytics
- Yseop
- Verint Systems
- Numenta
- Luminoso Technologies
- OpenText
- NLP Logix
- Megvii Technologies
- DigitalGenius
- Other Key Players
Recent Developments
- April 2024: Lexalytics launched a new sentiment analysis tool designed for investment firms. This tool enables users to analyze large volumes of news articles and social media posts to better understand public sentiment regarding specific stocks or market trends.
- June 2024: OpenText formed strategic partnerships with fintech companies to integrate its NLP capabilities into their platforms, enhancing data extraction and analysis processes for improved decision-making in finance.
Conclusion
In conclusion, Natural Language Processing (NLP) plays a significant role in the finance market by enabling the extraction of insights from vast amounts of unstructured data, such as news articles, financial reports, social media, and earnings calls. NLP tools help analysts track market sentiment, detect trends, and make data-driven decisions by processing textual data at scale. For example, sentiment analysis models can assess public sentiment towards a particular stock or market event, helping traders anticipate price movements. NLP algorithms also power automated trading strategies, allowing systems to react to market changes in real-time based on news and other textual data sources.
NLP is revolutionizing finance by improving decision-making, risk management, and trading efficiency. By automating the analysis of textual data, it provides valuable insights that are otherwise time-consuming to extract manually. As technology continues to advance, the integration of NLP in finance is expected to deepen, enhancing predictive capabilities and offering a competitive edge to those leveraging this innovative technology. However, challenges like data quality, ethical concerns, and regulatory hurdles need to be carefully managed for optimal outcomes.
Discuss your needs with our analyst
Please share your requirements with more details so our analyst can check if they can solve your problem(s)
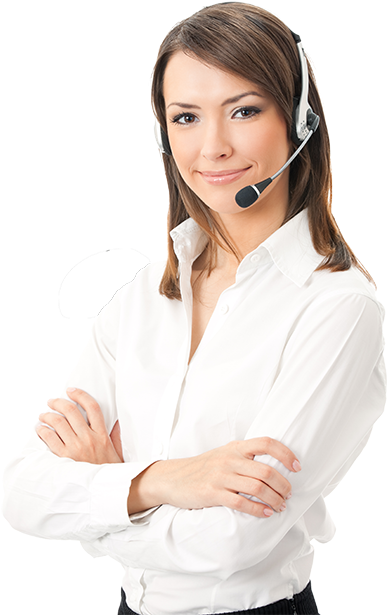