Table of Contents
As per the latest insights from Market.us, The global machine learning in fraud detection market is projected to reach USD 302.9 billion by 2034, growing significantly from USD 14.2 billion in 2024 at a CAGR of 35.80% during the forecast period from 2025 to 2034. This rapid expansion is driven by the rising need for advanced fraud prevention solutions across banking, e-commerce, and financial sectors.
In 2024, North America emerged as the leading region, holding over 34.7% of the market share, with revenues reaching USD 4.9 billion. The region’s strong position can be attributed to the widespread adoption of AI-driven fraud detection systems, a high concentration of financial institutions, and increasing digital transactions.
Machine learning (ML) has revolutionized fraud detection by providing advanced, adaptable solutions that can handle the complexity and variety of modern fraud scenarios, such as identity theft, credit card fraud, and insider trading. Traditional rule-based systems struggle to keep up with the sophistication of these activities, making ML an essential tool. By leveraging algorithms like logistic regression, decision trees, and neural networks, ML models can detect subtle, unusual patterns in data that may indicate fraudulent activities. These systems improve over time, learning from new data to quickly adapt to emerging fraud threats without human intervention.
According to Seon, Citibank cut phishing attacks by 70% by using machine learning tools that track suspicious behavior and detect scam attempts before they can harm users. This shows how businesses are turning to AI to strengthen security and build customer trust. In retail, Walmart reduced shoplifting by 25% through real-time video analysis powered by machine learning, helping the company lower losses while creating a safer shopping experience. The impact of AI is also clear in eCommerce, where fraud detection algorithms have reached up to 96% accuracy, according to a University of Jakarta whitepaper.
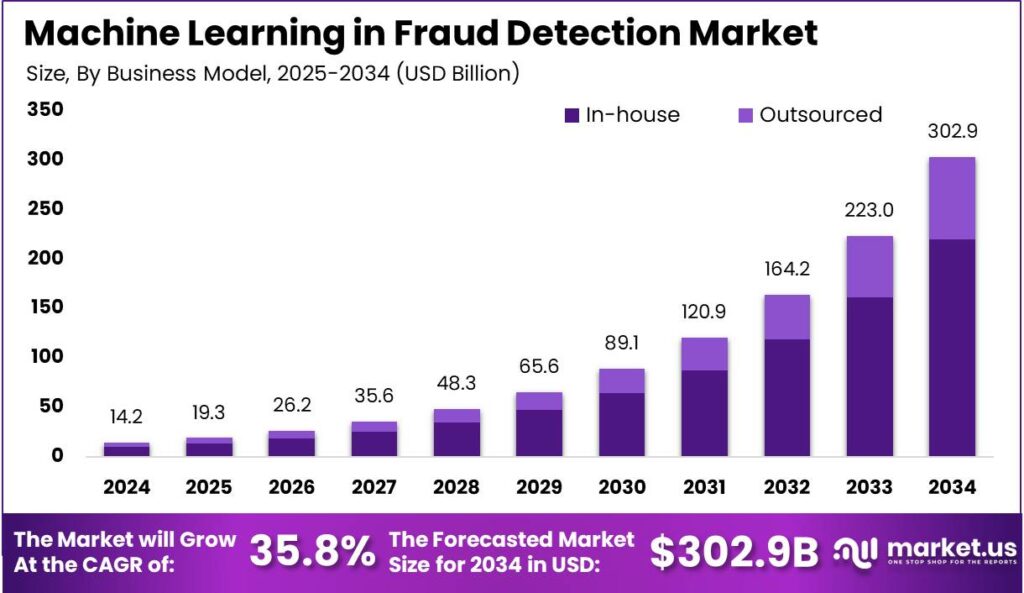
The market for ML in fraud detection is experiencing rapid growth, driven by an increasing demand for effective fraud prevention measures. The sector is set to expand significantly, with projections indicating an annual growth rate of 21.8% from 2024 to 2032. This surge is fueled by greater awareness among organizations and the widespread adoption of advanced technologies like ML, cloud computing, and big data analytics. As fraud techniques become more complex, the reliance on ML to safeguard assets and maintain consumer trust is intensifying.
Key drivers for the adoption of ML in fraud detection include the escalating complexity and frequency of fraud attempts and the limitations of traditional detection methods, which often fail to adapt to new and evolving tactics. Additionally, the digital transformation of services has expanded the attack surface for fraudulent activities, making conventional methods insufficient. Organizations are increasingly recognizing the need for dynamic, sophisticated ML models that not only detect but also learn and adapt to new fraud patterns in real-time.
There’s a robust demand for innovative ML solutions in fraud detection across various sectors, including banking, e-commerce, and healthcare. This demand creates substantial market opportunities for technology providers to develop and deploy advanced ML models that offer enhanced accuracy and faster response times. The ability of ML systems to analyze vast datasets and identify anomalies presents significant opportunities for reducing financial losses and improving security measures across industries.
Technological advancements are continually shaping the ML fraud detection landscape. The integration of artificial intelligence (AI) with ML has led to the development of sophisticated models like deep learning and neural networks, which excel in detecting complex fraud patterns. Innovations such as adversarial learning, federated learning, and explainable AI (XAI) are enhancing the capabilities of fraud detection systems, making them more effective, secure, and transparent.
Key Takeaways
- The Global Machine Learning in Fraud Detection Market is on a strong growth trajectory, projected to reach USD 302.9 billion by 2034, up from USD 14.2 billion in 2024. This expansion is driven by a remarkable CAGR of 35.80% over the forecast period from 2025 to 2034.
- Transactional Fraud Detection dominated the market, holding a 34.7% share, reflecting the increasing need for real-time fraud prevention in digital transactions.
- The In-house deployment model remained the preferred choice, securing 72.5% of the market share, as companies prioritize internal control over fraud detection systems.
- Large Enterprises took the lead, capturing more than 74.4% of the market, as these organizations heavily invest in advanced fraud prevention solutions.
- The Banking, Financial Services, and Insurance (BFSI) sector led the industry, holding a 45.7% market share, driven by rising fraud risks in online banking and financial transactions.
- North America emerged as the dominant regional player, contributing over 34.7% of the market, with revenues reaching USD 4.9 billion, fueled by strong investments in fraud prevention technologies.
U.S. ML in Fraud Detection Market
The US Machine Learning in Fraud Detection Market size was exhibited at USD 3.94 Bn in 2024 with CAGR of 36.3%. The United States and North America at large are leading in the machine learning in fraud detection market primarily due to several key factors. First, the region boasts a highly developed technological infrastructure, which is essential for the advanced computing power required by machine learning systems. This allows for the rapid deployment and scaling of AI-driven solutions across multiple sectors including banking, insurance, and e-commerce, which are significantly affected by fraudulent activities.
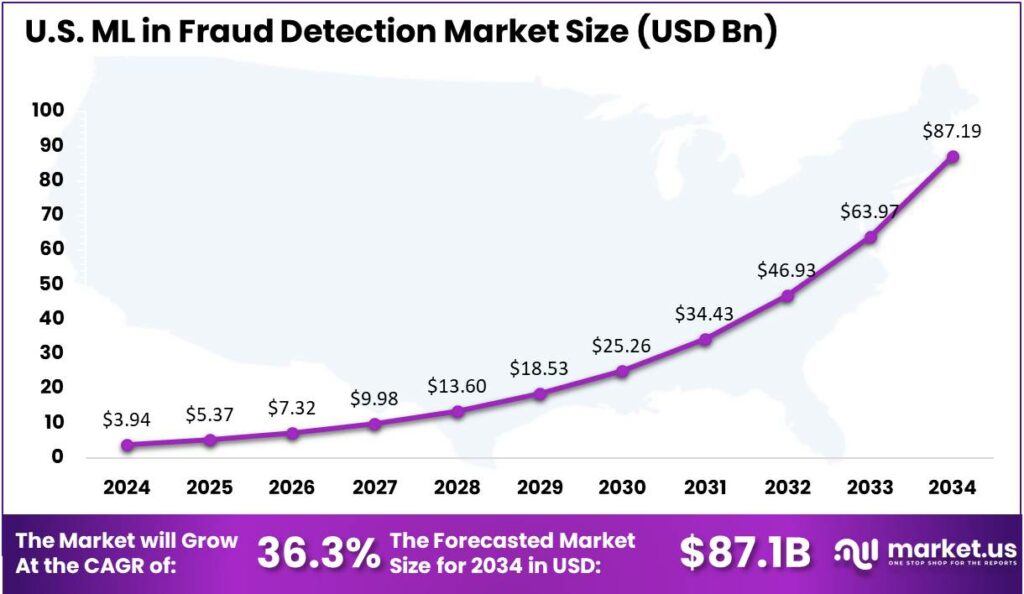
Moreover, In 2024, North America held a dominant position in the machine learning in fraud detection market, capturing more than a 34.7% share, with revenues reaching USD 4.9 billion. North America has a robust regulatory framework that encourages the adoption of innovative technologies to enhance fraud detection and prevention. For instance, regulations such as the Payment Card Industry Data Security Standard (PCI DSS) and the Sarbanes-Oxley Act compel companies to safeguard financial data, driving the demand for effective machine learning solutions that can identify and mitigate potential threats in real-time.
The concentration of major technology players in the US, such as IBM, Microsoft, and Google, who are at the forefront of developing machine learning algorithms, also contributes to the region’s dominance. These companies invest heavily in research and development, pushing the boundaries of what machine learning can achieve in terms of data processing and pattern recognition – critical components in fraud detection.
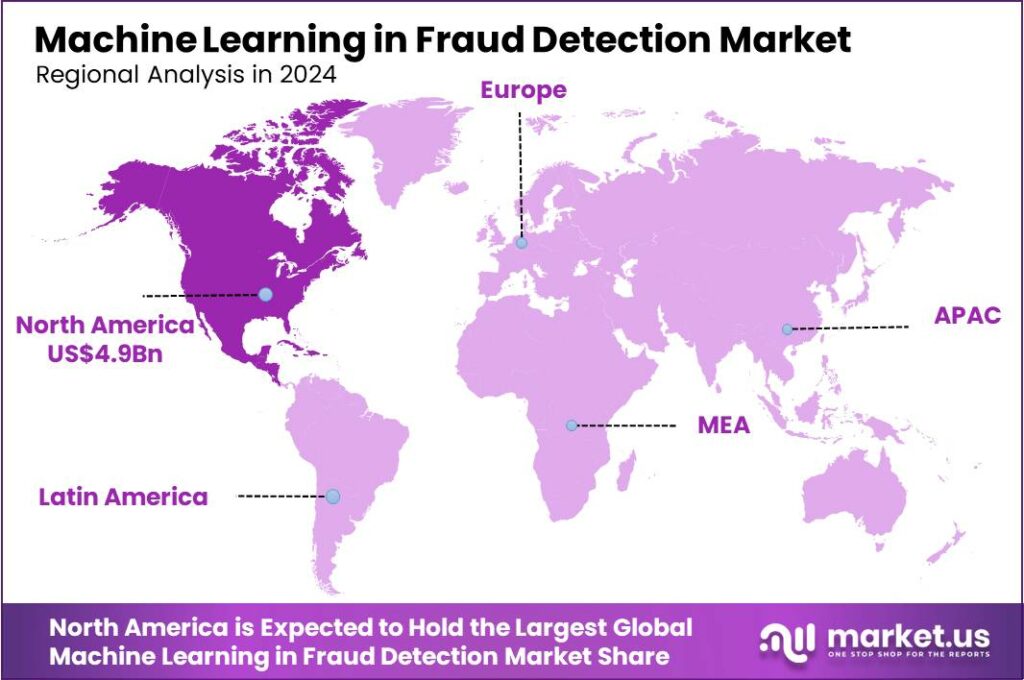
Report Segmentation
Type Analysis
The Transactional Fraud Detection segment emerged as a leader, accounting for over 34.7% of the market share. This growth is attributed to the increasing sophistication of cyberattacks, prompting businesses in sectors like banking, e-commerce, and healthcare to adopt advanced fraud detection solutions. The rise in online transactions has also significantly contributed to this growth, as more consumers engage in digital payments, creating opportunities for fraudsters to exploit vulnerabilities.
Business Model Analysis
The in-house segment dominated the market, capturing more than 72.5% of the share. Organizations are increasingly investing in internal fraud detection systems to maintain control over sensitive data and customize solutions to their specific needs. This approach allows for tailored strategies and quicker response times to emerging threats.
Enterprise Size Analysis
Large Enterprises held a commanding position, securing over 74.4% of the market share. Their substantial resources enable them to implement comprehensive fraud detection frameworks, integrating advanced technologies such as artificial intelligence and machine learning to monitor and analyze vast amounts of transactional data in real-time.
Industry Analysis
The Banking, Financial Services, and Insurance (BFSI) sector maintained a dominant stance, representing more than 45.7% of the market share. The critical need to safeguard financial transactions and comply with stringent regulatory standards drives the adoption of sophisticated fraud detection solutions within this industry. The increasing volume of digital transactions and the rising sophistication of cyber threats further contribute to this trend.
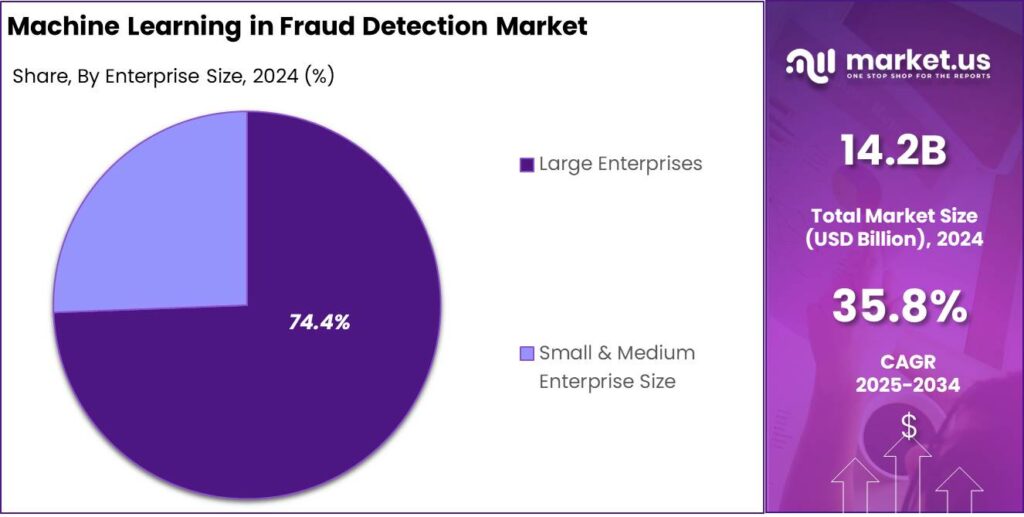
Key Market Segments
By Type
- Transactional Fraud Detection
- Identity Fraud Detection
- Insider Fraud Detection
- Network Fraud Detection
- Document Fraud Detection
- Others
By Business Model
- In-house
- Outsourced
By Enterprise Size
- Small & Medium Enterprise Size (SME’s)
- Large Enterprises
By Industry
- Banking, Financial Services and Insurance (BFSI)
- Government and Public Sector
- Aerospace & defense
- Healthcare
- IT and Telecom
- Automotive
- Retail and E-commerce
- Others (Gaming and Entertainment, Education, etc.)
Top Key Players in the Market
- Ravelin Technology Ltd.
- SEON Technologies Ltd.
- TrustDecision
- Trustpair
- AltexSoft
- SoulPage IT Solutions
- Stripe, Inc.
- SocioVerse Tech Pvt Ltd.
- Inscribe
- Anaptyss
- Experion Technologies
- Others
Conclusion
The machine learning-driven fraud detection market in 2024 is characterized by a strong emphasis on real-time transactional fraud detection, in-house security frameworks, and large enterprise adoption. The Transactional Fraud Detection segment has gained significant traction due to the rise in online transactions and increasing cyber threats.
In-house fraud detection models are preferred by organizations to maintain control over sensitive data and customize solutions to their specific needs. Large enterprises dominate the market as they have the resources to invest in cutting-edge fraud detection technologies, while the BFSI sector remains the primary driver of market demand, given its high exposure to financial fraud.
Discuss your needs with our analyst
Please share your requirements with more details so our analyst can check if they can solve your problem(s)
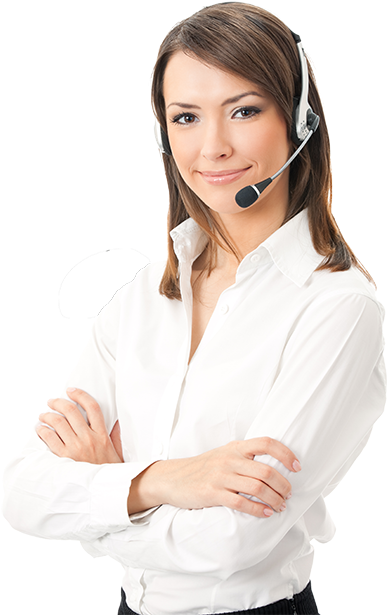