Table of Contents
- Report Overview
- Analyst’s Viewpoint
- Key Takeaways
- Impact Of AI
- Regional Analysis
- Market Segmentation
- Component Analysis
- Deployment Mode Analysis
- Optimization Type Analysis
- Network Type Analysis
- End-User Analysis
- Emerging Trends
- Top Use Cases
- Major Challenges
- Competitive Landscape
- Market Opportunities for Key Players
- Recent Developments
- Conclusion
Report Overview
The AI and RAN Traffic Optimization Market is projected to soar from USD 2.2 billion in 2024 to an impressive USD 27.2 billion by 2034, reflecting a robust compound annual growth rate (CAGR) of 28.60% during the forecast period from 2025 to 2034. In 2024, North America holds a commanding lead in the market, accounting for over 44.8% of the global share, with revenues reaching USD 0.9 billion, solidifying its dominant position in this rapidly expanding sector.
Artificial intelligence (AI) in Radio Access Network (RAN) traffic optimization represents a significant evolution in telecommunications, enhancing network management and service quality. This integration of AI with RAN optimizes data traffic flow across networks, ensuring efficient use of network resources, minimizing congestion, and improving user experience. In addition to optimizing data traffic flow, AI in Radio Access Network (RAN) traffic optimization also enables predictive maintenance and real-time network analytics.
The AI and RAN Traffic Optimization market is experiencing rapid growth, driven by several key factors. As data traffic surges due to the rise of internet-connected devices, video streaming, and real-time data needs, the demand for more sophisticated network management solutions has never been greater. AI’s ability to predict network loads and optimize data routing not only reduces latency but also significantly enhances the reliability of mobile networks. At the same time, the escalating threat of cyberattacks is spurring the adoption of AI, which plays a crucial role in strengthening cybersecurity.
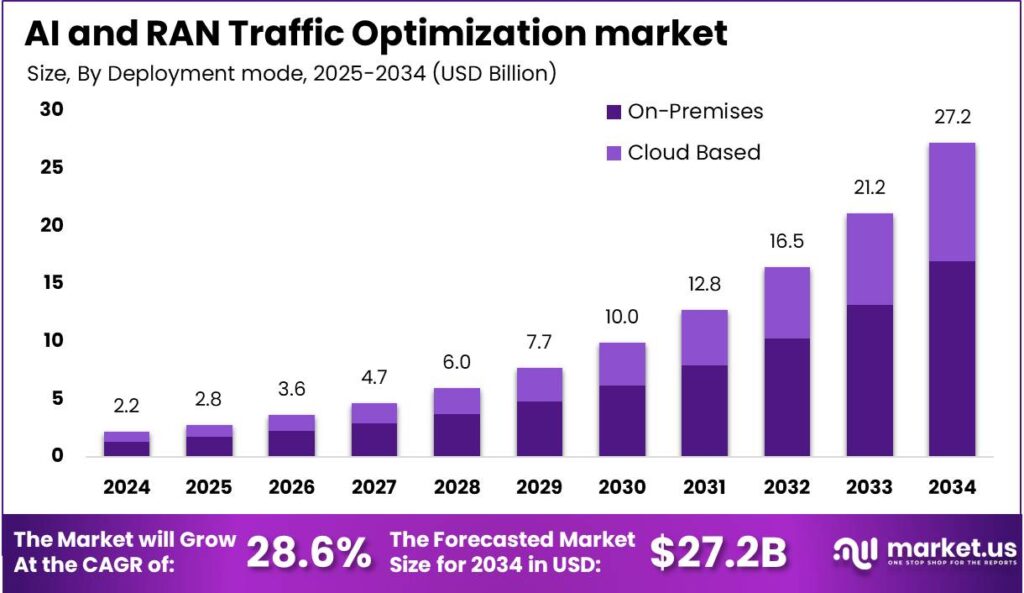
Emerging trends in AI and RAN include the deployment of machine learning algorithms for predictive maintenance, which anticipates and rectifies network faults before they affect service. The growth of 5G technology accelerates the adoption of AI in RAN, with AI-enabled applications such as dynamic spectrum management and energy efficiency improvements becoming increasingly prevalent. Moreover, AI facilitates the shift towards virtualized RAN (vRAN) architectures that offer enhanced scalability and flexibility.
Implementing AI in RAN optimization brings a wide array of business advantages. By automating key processes, telecom operators can significantly cut operational costs while boosting efficiency. The result is not only enhanced service reliability and connectivity, which directly improves customer satisfaction, but also a greater ability to scale operations swiftly. This flexibility allows operators to innovate their service offerings at a faster pace, giving them a competitive edge in the rapidly evolving telecommunications market.
Analyst’s Viewpoint
Opportunities in the telecommunications market are expanding as Artificial Intelligence (AI) is integrated into Radio Access Network (RAN) traffic optimization. AI-driven solutions offer a transformative approach, boosting network efficiency by predicting traffic patterns and dynamically allocating resources. This shift is accelerating with the adoption of next-generation networks like 5G, where AI’s ability to manage growing data traffic becomes essential.
Despite the promising advancements, the AI and RAN optimization sector faces several challenges. The complexity of RAN systems and the need for continuous algorithm improvements require substantial investment in R&D. Security concerns around AI algorithms and data privacy continue to pose risks. Additionally, the integration of AI into existing infrastructures demands significant technological and cultural shifts within organizations, making the adoption process potentially slow and costly.
Recent technological innovations in AI and RAN traffic optimization include the development of AI-powered applications such as predictive analysis tools and dynamic management systems. These innovations aim to enhance the performance of mobile networks by optimizing traffic distribution and resource management in real-time. Companies are developing advanced machine learning models that can adapt to changing network conditions, thus ensuring optimal performance across diverse environments and traffic scenarios.
Key Takeaways
- Hardware Segment: In 2024, the Hardware segment led the AI and RAN Traffic Optimization market, securing over 40.0% of the market share.
- On-Premises Segment: Dominating the market with more than 62.4% of the share, the On-Premises segment held a strong position in 2024.
- Traffic Load Balancing Segment: This segment captured more than 28.7% of the AI and RAN Traffic Optimization market in 2024, maintaining a significant market presence.
- 4G/LTE Networks Segment: In 2024, 4G/LTE Networks accounted for over 34.4% of the market share in AI and RAN Traffic Optimization.
- Telecommunication Service Providers: The Telecommunication Service Providers segment dominated the market in 2024, holding more than 62.9% of the total market share.
- Geographical Leadership: North America: North America led the global AI and RAN Traffic Optimization market in 2024, capturing more than 44.8% of the market share, with revenues reaching USD 0.9 billion.
- US Market Insights: The US AI and RAN Traffic Optimization market was valued at USD 0.84 billion in 2024, with a remarkable CAGR of 29%.
Impact Of AI
- Enhanced Uplink Traffic Management: AI applications contribute to a noticeable increase in uplink data traffic, as they require substantial data exchange between devices and network servers. This increase in uplink traffic necessitates robust network management and optimization strategies to handle the data efficiently.
- Customer Service Automation: AI-driven chatbots in telecommunications can automate routine customer inquiries, reducing response times and operational costs. This not only improves customer satisfaction but also optimizes network resource allocation by handling large volumes of queries without human intervention.
- Fraud Detection Improvements: AI algorithms are increasingly used in telecommunication networks to detect and prevent fraudulent activities, such as spam calls and SMS phishing. This application of AI helps in safeguarding revenue and enhancing network security.
- Network Performance Optimization: AI technologies, such as those developed by Fujitsu, enable real-time monitoring and dynamic optimization of network quality. This involves predicting network traffic patterns and adjusting resources proactively to maintain service quality without overusing energy.
- Innovation in Network Management: AI integration into RAN is spearheading innovations such as the development of new algorithms for load balancing and resource allocation. These AI-driven approaches outperform traditional methods, providing more efficient network management and better handling of user demands.
Regional Analysis
In 2024, the AI and RAN Traffic Optimization Market sees North America at the forefront, commanding a significant 44.8% of the global market share. Within this region, the United States alone contributes a substantial USD 0.84 billion, underscoring its pivotal role in the market’s dynamics. The total revenue for North America reaches approximately USD 0.9 billion, confirming its dominant position in this swiftly growing industry.
North America’s impressive growth can be traced to a combination of pivotal factors. Leading the charge is a robust technological infrastructure, bolstered by significant investments in AI and cutting-edge network technologies. At the heart of this growth are major tech companies, whose relentless innovation in AI algorithms is transforming traffic optimization.
Furthermore, the market’s expansion is fueled by the increasing demand for seamless mobile connectivity and the burgeoning number of internet-connected devices. As 5G deployment accelerates, the need for sophisticated RAN traffic management solutions becomes more pronounced, propelling the market forward. North America’s proactive stance on adopting new technologies and its robust regulatory environment also contribute to creating a favorable landscape for growth and innovation in the AI and RAN Traffic Optimization sector.
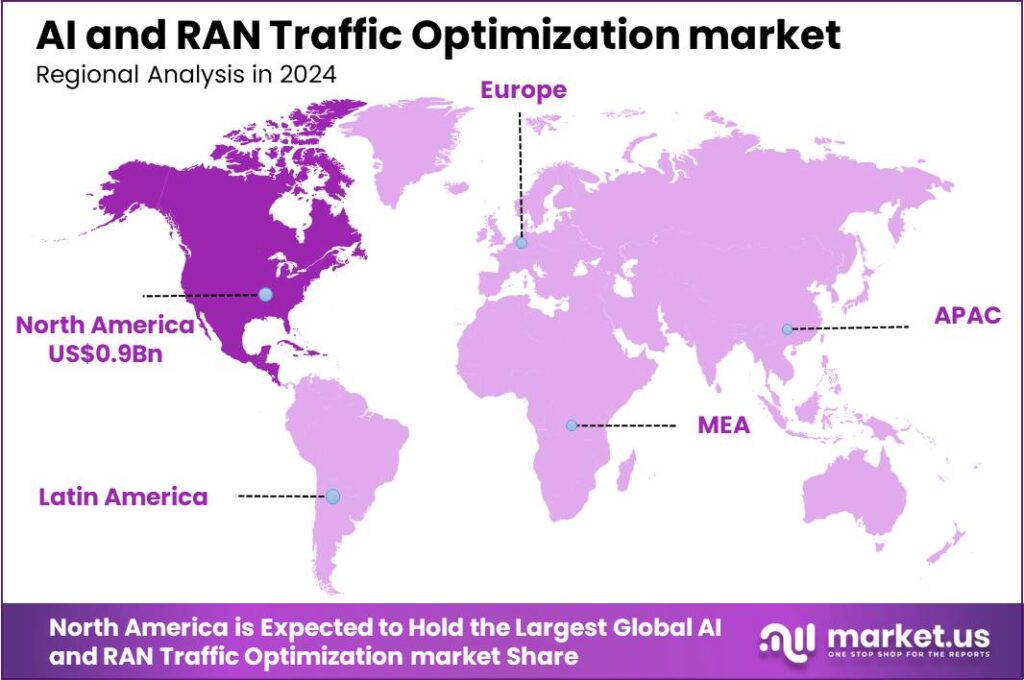
Market Segmentation
Component Analysis
In 2024, the Hardware segment was the leading contributor to the AI and RAN Traffic Optimization market, holding a substantial share of over 40.0%. This dominance can be attributed to the increasing reliance on advanced hardware solutions for optimizing network performance, particularly as AI-driven traffic management and resource allocation are becoming integral to modern telecommunications infrastructures. The need for high-performance servers, routers, and specialized processing units has contributed significantly to this market share.
Deployment Mode Analysis
The On-Premises segment held a commanding position in the market in 2024, capturing more than 62.4% of the AI and RAN Traffic Optimization market share. This is indicative of the preference for on-site infrastructure and solutions, where businesses and telecommunication service providers prioritize control, security, and reliability in their operations. On-premises systems offer advantages such as lower latency and the ability to manage data and traffic directly within their own facilities, which likely explains this significant market dominance.
Optimization Type Analysis
The Traffic Load Balancing segment also stood out in 2024, with a commanding market share of more than 28.7%. Traffic load balancing plays a crucial role in ensuring efficient distribution of data traffic across networks, preventing bottlenecks and improving the overall user experience. As the demand for high-speed and reliable data services grows, the importance of optimizing traffic flows through advanced balancing techniques has become a key driver for the growth of this segment.
Network Type Analysis
In 2024, the 4G/LTE Networks segment held a dominant share of over 34.4% of the AI and RAN Traffic Optimization market. Despite the ongoing rollout of 5G, 4G/LTE networks continue to play a major role in traffic optimization due to their widespread infrastructure and usage. Many regions and networks still rely heavily on 4G/LTE technologies, which necessitate ongoing optimization efforts to handle high data traffic volumes and ensure seamless connectivity, thereby sustaining the significance of this market segment.
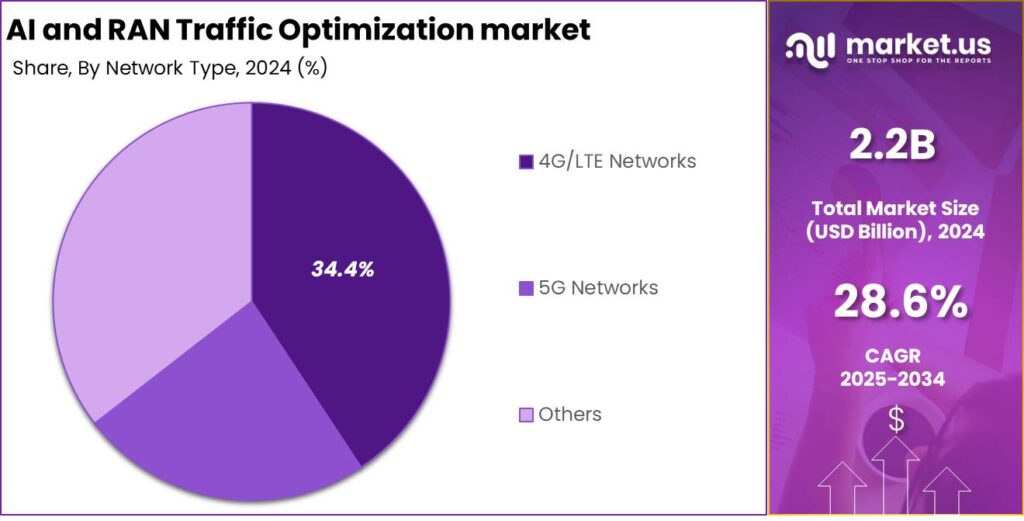
End-User Analysis
The Telecommunication Service Providers segment maintained a dominant market position in 2024, capturing more than 62.9% of the AI and RAN Traffic Optimization market share. This dominance is driven by the critical role service providers play in managing vast amounts of network traffic, including both AI-driven traffic optimization and RAN operations. As the primary stakeholders in deploying and maintaining telecommunications networks, service providers are the largest adopters of AI and RAN optimization technologies to ensure smooth and efficient operations across their networks.
Emerging Trends
- Real-Time Traffic Management: AI helps networks adjust instantly to changing data demands, ensuring smooth connections even during peak times. For example, Google’s AI traffic light system, tested in Israel, India, and the US, reduces stop-and-go traffic by up to 10-20%.
- Predictive Maintenance: By analyzing patterns, AI can foresee equipment issues before they happen, allowing for timely repairs and reducing unexpected outages. This proactive approach keeps networks running smoothly and improves user satisfaction.
- Energy Efficiency: AI optimizes power use in networks by managing resources based on real-time needs, which lowers energy consumption and supports environmental sustainability. This approach is crucial for reducing the carbon footprint of expanding network infrastructures.
- Enhanced Quality of Service (QoS): AI ensures that critical services like emergency calls or video streaming receive priority, maintaining high-quality connections by dynamically allocating network resources where they’re needed most.
- Open RAN Integration: The move towards Open RAN allows AI to be more effectively incorporated into network systems, promoting innovation and flexibility by enabling networks to use components from different vendors. This openness leads to more customized and efficient network solutions.
Top Use Cases
- Traffic Steering: AI helps distribute network traffic efficiently by directing data through the best available paths. This ensures users experience consistent service quality, even during peak times.
- Quality of Experience (QoE) Optimization: By analyzing user data, AI can predict and enhance the quality of experience for services like video streaming. This means fewer interruptions and better service for users.
- Massive MIMO Optimization: AI fine-tunes the performance of multiple-input and multiple-output (MIMO) systems, which use multiple antennas to improve communication. This leads to better coverage and faster data speeds for users.
- Handover Management: AI improves how mobile devices switch between network cells, ensuring seamless connectivity during movement. This reduces dropped calls and maintains consistent data connections.
- RAN Slice SLA Assurance: AI monitors and ensures that different network slices meet their service level agreements (SLAs). This is crucial for applications that require guaranteed performance, like emergency services.
Major Challenges
- Data Quality and Standardization: Telecom data varies widely and often lacks standard formats, making it hard for AI systems to process effectively. Ensuring consistent and high-quality data is essential for accurate AI-driven optimizations.
- Trustworthiness: AI models must be highly reliable and safe to manage critical network operations. Without meeting strict reliability standards, these models may not be trusted for essential tasks.
- Resource Requirements: Training AI models demands significant computational power, data storage, and energy. This high resource consumption can lead to increased operational costs and necessitate substantial infrastructure investments.
- Contextual Understanding: AI applications need to interpret data within the specific context of telecom operations. Without this understanding, AI models may misinterpret data, leading to incorrect decisions and inefficiencies in network management.
- Integration with Existing Systems: Incorporating AI into current RAN architectures requires seamless integration. Achieving this without disrupting ongoing services or requiring extensive overhauls is a significant challenge.
Competitive Landscape
- Qualcomm Technologies has emerged as a leader in the development of AI-driven solutions for mobile networks. The company integrates advanced AI algorithms into its chipsets, enabling efficient RAN traffic management. By focusing on 5G and IoT technologies, Qualcomm enhances data throughput and helps operators optimize network resources in real-time, thereby ensuring better service quality and user satisfaction.
- Nokia is a key player in RAN traffic optimization, known for its cutting-edge networking hardware and software solutions. The company uses AI to improve network efficiency and automate tasks such as load balancing and interference management. With its focus on next-gen 5G technology, Nokia enables operators to maximize their network capacity while reducing operational costs, ultimately delivering superior connectivity experiences.
- Telefonaktiebolaget LM Ericsson is a well-established player in the telecom sector, providing robust AI and machine learning-based solutions for RAN optimization. The company’s innovations are designed to streamline network management, enhance data processing, and enable predictive maintenance. Ericsson’s ability to integrate AI with its RAN infrastructure helps telecom operators optimize traffic, reduce congestion, and improve overall network performance.
- Huawei Technologies is one of the global leaders in telecommunications and AI-driven RAN optimization. The company utilizes AI algorithms to enhance the performance of its RAN solutions, focusing on improving network efficiency and reducing latency. Huawei’s strong presence in both hardware and software enables telecom operators to better manage their traffic load, paving the way for high-speed, reliable, and cost-effective 5G networks.
Market Opportunities for Key Players
- Enhanced Network Efficiency and Cost Savings: As telecom networks evolve, AI-driven solutions offer significant improvements in network management and operational efficiency. This includes predictive maintenance, which can minimize downtime and reduce maintenance costs.
- AI-Powered Customer Service Solutions: The deployment of AI in customer service, particularly through virtual assistants and chatbots, provides telecom companies the opportunity to enhance customer interaction and satisfaction while reducing operational costs. These AI tools can handle numerous customer queries simultaneously, offering round-the-clock service and reducing the burden on human agents.
- Fraud Detection and Security: AI technologies is instrumental in enhancing security within telecom networks by detecting and preventing fraud. Implementation of AI for real-time monitoring can analyze patterns and predict fraudulent activities, thus safeguarding revenues and enhancing trust in telecom services.
- Network Traffic Management: With the continuous increase in data traffic, particularly driven by AI applications, there is a growing need for advanced traffic management solutions. AI can optimize traffic flow and improve the quality of service by anticipating and mitigating congestion before it occurs. This is crucial as AI applications tend to generate bursty and unpredictable traffic patterns that can strain network resources.
- The Power of Collaborative Ecosystems and Alliances: The growing significance of collaborative efforts in the telecom industry is highlighted by the formation of alliances like the AI-RAN Alliance. These strategic partnerships enable companies to pool resources, share insights, and collectively drive innovation.
Recent Developments
- June 2024: Huawei announced plans to integrate AI into its networks through the RAN Intelligent Agent initiative, targeting increased productivity across various regions by optimizing network operations and energy efficiency.
- October 2024: ZTE and Smartfren achieved a breakthrough with their Native-AI-based RAN Computing commercialization, enhancing user experience through integrated communication and computing capabilities directly at base stations.
- December 2024: Nokia deployed its MantaRay Cognitive SON solution in stc Group’s network, achieving a 30% utilization rate increase on loaded cells and a 10% improvement in user throughput during peak traffic periods. This AI-powered solution automates RAN operations, significantly enhancing network performance.
- December 2024: Samsung accelerated its integration of AI into RAN technologies, demonstrating improvements in data speeds and energy efficiency at the Silicon Valley Future Wireless Summit. The company aims to develop AI-native wireless networks as part of its future telecommunications strategy.
Conclusion
In summary, the AI and RAN (Radio Access Network) traffic optimization market is experiencing significant growth, driven by the increasing demand for efficient network management and improved user experience. AI technologies, such as machine learning and data analytics, are being increasingly integrated into RAN systems to optimize traffic flow, reduce latency, and enhance network performance. This transformation is particularly crucial with the rollout of 5G networks, which require advanced optimization to handle the massive surge in data traffic and the growing number of connected devices.
The market’s expansion is also fueled by the rising need for automation and real-time decision-making in network operations. As telecom operators strive to meet the complexities of modern communication, AI-driven solutions provide the scalability and flexibility required for future-proofing their networks. Ultimately, the integration of AI in RAN traffic optimization is poised to revolutionize the telecommunications industry by enabling more reliable, cost-effective, and adaptive network infrastructures.
Discuss your needs with our analyst
Please share your requirements with more details so our analyst can check if they can solve your problem(s)
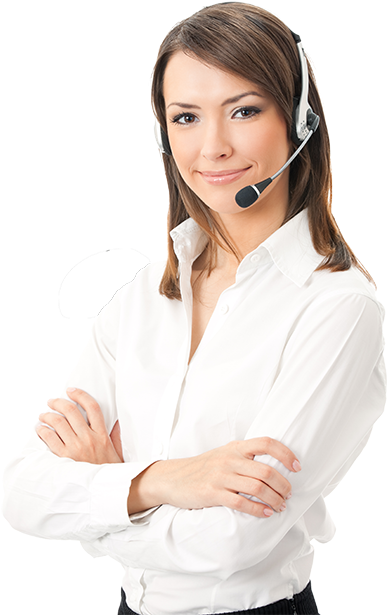