Table of Contents
Introduction
According to AI chips Statistics, AI chips, also called AI accelerators, are specialized hardware designed to enhance the speed and efficiency of artificial intelligence tasks. Unlike traditional CPUs, they’re optimized for parallel processing and specific AI operations, like matrix calculations in neural networks.
Types include GPUs, TPUs, and ASICs. These chips boost performance metrics like FLOPS, INT8/INT4 ops, and memory bandwidth to balance power efficiency and computation power. They’re a crucial part of the AI ecosystem, aiding tasks from image recognition to natural language processing and driving AI research and application advancements.
Editor’s Choice
- The Artificial Intelligence Chipsets Market is expected to reach USD 429.35 billion at a CAGR of 36.8%
- The AI chip market was valued at 20 billion USD in 2022, expanding at a compound annual growth rate (CAGR) of approximately 30.3%.
- It is anticipated to reach new heights, culminating in a valuation of 165 billion USD by 2030.
- In 2017, Intel became the first AI chip manufacturer to exceed $1 billion in sales.
- NVIDIA’s GPUs have consistently pushed the boundaries of FLOPS performance. For instance, the NVIDIA A100 GPU, released in 2020, delivered over 19 teraflops (TFLOPS) of single-precision (FP32) performance and around 9.7 TFLOPS of double-precision (FP64) performance.
- Venture capital spending in the AI sector followed a dynamic trajectory over the years. In the first quarter of 2018, the investment amounted to $282 million, marking the inception of this financial trend.
- Venture capital spending in the AI sector followed a dynamic trajectory over the years. In the first quarter of 2018, the investment amounted to $282 million, marking the inception of this financial trend.
- Based on a survey conducted in 2022, the global implementation of quantum computing surpassed the adoption rate of artificial intelligence (AI).
AI Chip Market Overview
- The AI chip market has witnessed a remarkable growth trajectory over the years, with its market size consistently expanding at a compound annual growth rate (CAGR) of approximately 30.3%.
- In 2022, the market was valued at 20 billion USD, demonstrating the industry’s potential.
- Building on this momentum, the market experienced substantial growth, reaching 28 billion USD in 2023 and a remarkable 39 billion USD in 2024.
- Looking ahead, the AI chip market is anticipated to reach new heights, culminating in a valuation of 165 billion USD by 2030.
Market Players and Key Companies
- Nvidia has an extensive history in manufacturing graphics processing units (GPUs) for the gaming sector, with a presence dating back to the 1990s. The company is also actively developing AI chips such as Volta, Xavier, and Tesla. Recent outstanding performance in generative AI contributed to impressive outcomes in Q2 2023, resulting in a valuation surpassing one trillion.
- Intel, a significant player in the industry, boasts a rich heritage in technology advancement. In 2017, the company became the first AI chip manufacturer to exceed $1 billion in sales.
- Google’s Cloud TPU is meticulously crafted to accelerate machine learning and powers various Google products, including Translate, Photos, and Search. This capability can be accessed through the Google Cloud platform. Another offering, Google Alphabet’s Edge TPU, is tailored for smaller devices such as smartphones, tablets, and IoT devices, delivering efficient edge computing solutions.
- AMD, a chip manufacturer, provides various products encompassing CPUs, GPUs, and AI accelerators. For instance, their Alveo U50 data center accelerator card stands out with an impressive 50 billion transistors. It excels in tasks like efficiently managing embedding datasets and swiftly executing graph algorithms.
Performance Metrics
Floating-Point Operations Per Second (FLOPS)
- NVIDIA’s GPUs have consistently pushed the boundaries of FLOPS performance. For instance, the NVIDIA A100 GPU, released in 2020, delivered over 19 teraflops (TFLOPS) of single-precision (FP32) performance and around 9.7 TFLOPS of double-precision (FP64) performance.
- Google’s Tensor Processing Units (TPUs) are known for their high AI-related FLOPS. The TPU v3, introduced in 2018, was reported to provide around 420 TFLOPS of AI performance.
- Intel’s Nervana Neural Network Processor for Training (NNP-T) aimed to provide strong AI performance. The Intel NNP-T 1000, released in 2020, targeted 119 TFLOPS of AI performance.
- AMD GPUs, such as the Radeon Instinct MI100, introduced in 2020, promised around 11.5 TFLOPS of double-precision performance.
- Custom chips, like Apple’s M1, introduced in 2020, showcased impressive AI capabilities with around 2.6 TFLOPS of throughput.
Memory Bandwidth and Cache Size
- On-chip memory stands out for its impressive bandwidth and efficiency despite its capacity limitations. Notable instances, such as Cerebras achieving remarkable benchmarks of 9 petabytes/sec memory bandwidth and 18GB on-chip memory, effectively bolster its 400,000 AI-focused cores.
- Prominent offerings like AMD’s Radeon RX Vega 56, NVIDIA’s Tesla V100, Fujitsu’s A64FX processor featuring 4 HBM2 DRAMs, and NEC’s Vector Engine Processor equipped with 6 HBM2 DRAMs, underline the preference for HBM2 in supercomputing scenarios.
- GDDR6 exhibits notably higher power consumption (between three and a half to four times) on the System-On-Chip (SoC) PHY when juxtaposed with HBM2.
- Additionally, GDDR6 occupies a larger PHY area (one and a half to one and three-quarters times) on the SoC.
Power Efficiency Metrics
- In April 2023, Qualcomm Inc’s AI chips performed better than Nvidia Corp’s in two out of three measurements related to power efficiency.
- A significant cost factor pertains to power consumption. Qualcomm capitalized on its expertise in chip design for battery-dependent devices, such as smartphones, to create the Cloud AI 100 chip with a strong emphasis on reducing power usage.
- Regarding power efficiency, Qualcomm’s chips achieved 227.4 server queries per watt, surpassing Nvidia’s 108.4 queries per watt.
- Qualcomm also exceeded Nvidia in object detection, achieving a score of 3.8 queries per watt compared to Nvidia’s 2.4 queries per watt.
Notable AI Chips and Their Specifications
NVIDIA GPUs
Tesla V100
- Having 640 Tensor Cores, the Tesla V100 stands out as the first GPU globally to surpass the 100 teraFLOPS (TFLOPS) mark in deep learning performance.
- The upcoming iteration of NVIDIA NVLink establishes connections between multiple V100 GPUs, achieving speeds of up to 300 GB/s, thereby crafting the planet’s most potent computing servers.
- Engineered for optimal effectiveness within present hyperscale server racks, the Tesla V100 is tailored to provide peak performance.
- At its core, the Tesla V100 GPU prioritizes AI, resulting in a remarkable 30-fold increase in inference performance compared to a CPU server.
- The NVIDIA Tesla V100 boasts an array of specifications across different variants, such as Tesla V100 for NVLink, Tesla V100 for PCIe, and Tesla V100S for PCIe.
- Memory-wise, the GPUs are equipped with CoWoS Stacked HBM2, with capacities ranging from 16 GB to 32 GB and bandwidths ranging from 900 GB/s to 1134 GB/s.
A100
- Their comprehensive specifications distinguish the A100 80GB PCIe and the A100 80GB SXM. In terms of computational prowess, these GPUs deliver 9.7 TFLOPS in FP64, 19.5 TFLOPS in FP64 Tensor Core, 19.5 TFLOPS in FP32, and impressive figures like 156 TFLOPS (or 312 TFLOPS) in Tensor Float 32 (TF32), 312 TFLOPS (or 624 TFLOPS) in BFLOAT16 Tensor Core, and 312 TFLOPS (or 624 TFLOPS) in FP16 Tensor Core.
- INT8 Tensor Core operation reaches 624 TOPS (or 1248 TOPS).
- Both GPUs have 80GB of HBM2e GPU memory, boasting remarkable bandwidths of 1,935 GB/s and 2,039 GB/s, respectively.
- Their thermal design power (TDP) varies with the A100 80GB PCIe at 300W and the A100 80GB SXM at 400W.
- These GPUs support multi-instance GPU configurations with up to 7 MIGs at 10GB each.
Google TPUs
- The series of Tensor Processing Units (TPUs), including TPUv1, TPUv2, TPUv3, TPUv4, and Edge v1, were introduced in 2016, 2017, 2018, 2021, and 2018 respectively.
- The clock speeds of these TPUs have experienced adjustments over time, reaching up to 1050 MHz for TPUv4.
- With TPUv4 housing 32 GiB of HBM memory, memory capacity has increased, offering a substantial memory bandwidth of 1200 GB/s.
- In terms of power consumption, TPUv4 holds the lowest TDP at 170W. These TPUs have shown remarkable improvements in processing capabilities, with TPUv4 leading with 275 TOPS (Tera Operations Per Second).
- Efficiency has also increased significantly, with TPUv4 achieving 1.62 TOPS/W, marking a remarkable advancement from TPUv1’s 0.31 TOPS/W.
AMD GPUs
Radeon Instinct MI100
- The Radeon Instinct MI100 has a graphics processor known as Arcturus, under the variant Arcturus XL, which operates on the CDNA 1.0 architecture.
- Produced by TSMC using a 7nm process, it encompasses 25,600 million transistors, yielding 34.1 million per mm² density within a substantial die size of 750 mm².
- The graphics card, part of the Radeon Instinct generation, was released on November 16th, 2020, marking it as an active production.
- Accompanied by a 16 KB L1 cache per compute unit and an 8 MB L2 cache, its theoretical performance is notable, with a pixel rate of 96.13 GPixel/s and a texture rate of 721.0 GTexel/s.
Ongoing Trends
Venture capital (VC) Spending On AI and ML Semiconductors
- Venture capital spending in the AI sector followed a dynamic trajectory over the years. In the first quarter of 2018, the investment amounted to $282 million, marking the inception of this financial trend.
- Closing the year on a positive note, the fourth quarter of 2020 reported an impressive $828 million investment, showcasing a rebound in investor confidence.
- The first quarter of 2021 marked a remarkable pinnacle, with venture capital spending soaring to $1,767 million, underscoring the industry’s rapid expansion and enduring appeal to investors.
Quantum Computing’s Impact on AI
- Based on a survey conducted in 2022, the global implementation of quantum computing surpassed the adoption rate of artificial intelligence (AI).
- Almost half of the participants (49%) expressed that their adoption of quantum computing was more rapid than AI, while only 17% mentioned a slower progression.
- This trend was especially pronounced in North America, where 62% of the surveyed indicated a quicker pace of adopting quantum computing than AI.
Discuss your needs with our analyst
Please share your requirements with more details so our analyst can check if they can solve your problem(s)
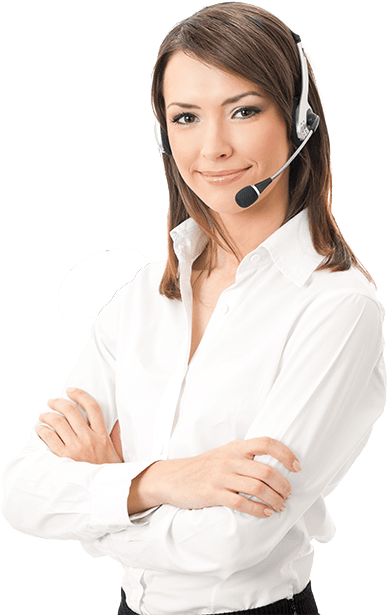