Table of Contents
AI in Data Quality market refers to the use of artificial intelligence to enhance the quality and integrity of data across industries. AI-driven tools enable businesses to automate the processes of data cleaning, validation, and anomaly detection. This technology helps organizations ensure that their data is accurate, consistent, and reliable, which is essential for making informed business decisions. With businesses increasingly relying on data for strategic initiatives, maintaining high data quality has become a competitive advantage. The growing complexity of data systems and the need for real-time data processing are fueling the adoption of AI in data quality management.
The AI in Data Quality Market is experiencing rapid growth, driven by several key factors. First, the increasing volume of data generated by businesses and the rise of big data analytics have made it more challenging for organizations to manage data manually. With companies relying on accurate and clean data to drive decision-making, the demand for automated data quality solutions powered by AI has surged. Additionally, industries such as healthcare, finance, and e-commerce are increasingly adopting AI-based tools to improve data governance and ensure compliance with regulatory standards.
Another significant factor driving the market is the growing importance of data-driven decision-making. Companies across various sectors are leveraging AI to unlock the full potential of their data, but this is only possible if the underlying data is of high quality. AI technologies, including machine learning (ML) and natural language processing (NLP), are being integrated into data quality processes to automate complex tasks and improve efficiency.
The demand for AI-based data quality solutions is rising rapidly, fueled by the need to manage and derive insights from large and complex datasets. As businesses move toward digital transformation, data quality becomes critical for the successful implementation of AI, analytics, and machine learning models. AI tools can ensure that the data feeding into these systems is accurate, relevant, and free from biases or errors, which is crucial for delivering reliable results.
Technological advancements in AI algorithms are also helping improve data quality management. New capabilities, such as self-learning algorithms and predictive analytics, enable AI tools to identify potential data quality issues before they affect business operations. Moreover, advancements in cloud-based solutions are making AI in data quality more accessible to businesses of all sizes, allowing them to adopt cost-effective and scalable solutions.
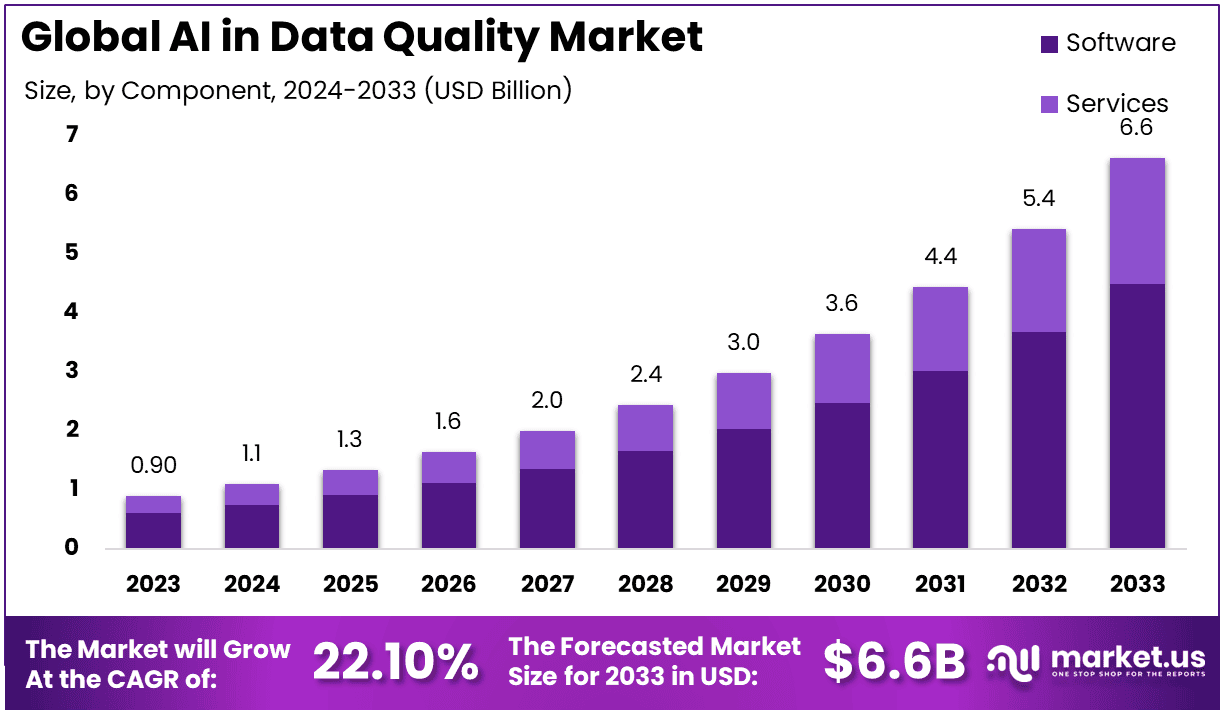
The global AI in Data Quality Market is expected to reach USD 6.6 Billion by 2033, up from USD 0.9 Billion in 2023, growing at a CAGR of 22.10% during the forecast period from 2024 to 2033. This growth presents numerous opportunities for technology providers to capitalize on the increasing adoption of AI for data quality management.
One key opportunity lies in the healthcare industry, where the need for accurate and secure patient data is critical for improving patient outcomes and regulatory compliance. Similarly, industries like financial services, which deal with large volumes of sensitive data, are adopting AI to ensure data integrity and compliance with evolving regulations.
There is also an opportunity in the small and medium-sized enterprise (SME) sector. As AI-powered data quality solutions become more affordable and scalable, SMEs can leverage these technologies to improve their data management practices, thereby enhancing decision-making and operational efficiency.
Artificial Intelligence (AI) is revolutionizing data quality management by automating processes, enhancing accuracy, and enabling predictive analytics. According to a recent survey, approximately 64% of organizations plan to invest in AI technologies to improve their data platforms. AI significantly streamlines the data cleaning process by identifying and rectifying errors such as duplicates, inconsistencies, and missing values. For instance, machine learning algorithms can reduce manual intervention in data cleansing by up to 70%, allowing data teams to focus on more strategic tasks.
Moreover, AI excels in real-time anomaly detection, identifying outliers or unusual patterns that may indicate data entry errors or fraudulent activities. This capability is crucial for maintaining data integrity and preventing costly mistakes; studies show that companies can save up to 30% in operational costs by leveraging AI for anomaly detection. Additionally, AI’s predictive analytics capabilities enable organizations to forecast missing or unknown values within datasets by analyzing existing patterns, improving overall data completeness and accuracy.
The benefits of AI-driven data quality management are substantial. Organizations utilizing AI can achieve up to a 50% improvement in data accuracy and completeness, leading to better strategic decision-making. As companies increasingly adopt these technologies, they will be better equipped to handle vast amounts of information while ensuring high standards of data quality. In summary, the integration of AI into data quality statistics not only enhances current practices but also sets the stage for innovative approaches to managing and utilizing data effectively.
AI algorithms can identify and correct errors in data much more efficiently than manual methods, leading to a higher overall accuracy of the dataset. Machine learning models can effectively flag outliers and anomalies in data, which might indicate potential errors or inconsistencies. AI can automate repetitive data cleaning tasks like duplicate removal, missing value imputation, and data formatting, saving time and resources.
AI can be used to validate data against predefined rules and standards, ensuring data integrity and consistency. A study might show that implementing an AI-based data cleaning process reduced the percentage of missing values in a customer database from 15% to 2%. An AI model could identify and flag 90% of outliers in a financial dataset, significantly improving data quality for analysis. An AI-powered system might achieve a 95% success rate in automatically identifying and removing duplicate records from a large customer database.
AI in Data Quality Statistics
- The global AI in Data Quality market was valued at USD 0.9 billion in 2023 and is projected to reach USD 6.6 billion by 2033, growing at a robust CAGR of 22.10%.
- North America holds a significant market share, contributing 38.2% of the global revenue, with an estimated USD 0.34 billion in revenue in 2023.
- The Software segment is the dominant component, accounting for 67.9% of the market share, reflecting the growing adoption of AI-powered software solutions for data quality management.
- Cloud-based deployment is the leading mode, representing 65.1% of the market share. Cloud solutions enable scalability and real-time data processing, driving their widespread adoption.
- The Large Enterprises segment leads the market with a 68.0% share, as large organizations increasingly invest in AI-driven data quality tools to manage complex data sets.
- The BFSI (Banking, Financial Services, and Insurance) industry holds a strong position, accounting for 21.5% of the market, as financial institutions prioritize data accuracy for compliance and decision-making.
- The global AI in Data Quality market is experiencing rapid growth due to increasing data complexity, rising regulatory demands, and a surge in big data analytics across industries.
- Continued innovations in machine learning and natural language processing (NLP) are enhancing the capabilities of AI in improving data accuracy and integrity, further fueling market growth.
Emerging Trends
- Increased Adoption of Automated Data Cleaning Tools
One of the most prominent trends in AI in the data quality market is the growing adoption of automated data-cleaning tools. Businesses are increasingly leveraging AI to eliminate human errors and improve the efficiency of data cleansing processes. AI algorithms are now capable of automatically detecting and correcting data discrepancies in real time, reducing the time and resources spent on manual data management.
- Rise of Machine Learning and NLP for Data Validation
Machine learning (ML) and natural language processing (NLP) technologies are being integrated into AI data quality solutions to enhance data validation capabilities. These tools can analyze large volumes of unstructured data and detect inconsistencies or errors more effectively than traditional methods. This trend is improving the overall accuracy and reliability of business-critical data across various industries.
- AI-Powered Predictive Data Quality
Predictive analytics, driven by AI, is becoming a significant trend in data quality management. AI systems can now forecast potential data issues before they occur, allowing organizations to take preventive actions. By analyzing historical data patterns and trends, these systems can predict anomalies, errors, and inconsistencies in future data, helping businesses maintain high data quality proactively.
- Integration with Cloud-Based Platforms
With the rise of cloud computing, AI in data quality is increasingly being deployed on cloud-based platforms. Cloud solutions offer scalability, flexibility, and cost-efficiency, allowing organizations of all sizes to implement AI-driven data quality solutions without investing heavily in on-premise infrastructure. This trend is expected to fuel the market growth, as more companies migrate to the cloud and seek AI-powered data management tools.
- Growing Focus on Real-Time Data Quality Monitoring
As businesses rely more on real-time data for decision-making, there is a growing demand for real-time data quality monitoring. AI-powered systems are now capable of continuously assessing data quality in real-time, ensuring that businesses can address data issues as they arise. This trend is particularly beneficial in sectors such as finance, healthcare, and retail, where timely and accurate data is crucial to operations.
Top Use Cases
- Automated Data Cleaning and Correction
AI in data quality is widely used for automating data cleaning and correction processes. Machine learning algorithms can identify anomalies, inconsistencies, and missing values in large datasets, automatically flagging or correcting errors. This reduces human intervention and improves the speed and accuracy of data preparation. For example, AI can automate the detection of duplicate records or identify outlier data points, improving data accuracy for businesses. The global AI in data cleaning market is projected to grow by over USD 1 billion by 2027, showcasing its growing importance.
- Real-Time Data Validation for Financial Institutions
Financial institutions are increasingly using AI for real-time data validation, ensuring that the data entered into their systems is accurate, consistent, and compliant with industry regulations. AI tools help in validating transactions, customer data, and financial reports, which is crucial in reducing fraud and ensuring compliance with financial regulations like GDPR and Basel III. For example, AI-powered systems in banks are able to validate transactional data in milliseconds, ensuring faster processing and reduced errors.
- Data Enrichment for Marketing Campaigns
AI-driven data quality tools are widely used in marketing to enhance and enrich customer data. By analyzing consumer behavior, preferences, and transaction history, AI can generate deeper insights, ensuring that marketing teams have the most up-to-date and accurate information. As per reports, 80% of marketing teams now use AI-driven data quality solutions to enhance their CRM systems, thereby improving targeted marketing efforts and customer segmentation.
- Improving Customer Data in CRM Systems
AI in data quality is also playing a key role in enhancing customer relationship management (CRM) systems. By using AI to clean and validate customer data, businesses can maintain a more accurate and up-to-date customer database. This, in turn, improves customer interactions, drives better service, and enables personalized communication strategies. The global market for AI-enhanced CRM systems is forecasted to exceed USD 20 billion by 2025, driven by the increasing demand for high-quality customer data.
- Data Integration and Governance in Large Enterprises
Large enterprises with vast amounts of distributed data are leveraging AI to ensure seamless data integration and governance. AI tools are helping businesses identify and reconcile data discrepancies between different systems and databases. By automating data mapping, transformation, and validation, AI ensures that integrated datasets are reliable and ready for decision-making. Companies in sectors such as healthcare and manufacturing are increasingly investing in AI to streamline data integration and governance, with the market for AI-driven data governance solutions expected to grow at a CAGR of 21.8% between 2024 and 2032.
Major Challenges
- Data Privacy and Security Concerns
One of the major challenges in AI for data quality is ensuring the privacy and security of sensitive data. As AI systems analyze and process vast amounts of data, they may inadvertently expose sensitive information. In industries like healthcare, finance, and government, where data privacy is a top concern, AI solutions must comply with strict regulations such as GDPR or HIPAA. Data breaches or mishandling of personal data can lead to significant financial losses and reputational damage. According to a report by IBM, the average cost of a data breach in 2023 was approximately USD 4.45 million, underscoring the importance of safeguarding data while using AI for quality management.
- Integration with Legacy Systems
Integrating AI-driven data quality solutions with legacy systems is another significant challenge. Many businesses continue to rely on older data management systems that were not designed for AI or machine learning integration. Transitioning from these legacy systems to AI-powered solutions often requires significant investments in infrastructure, time, and skilled personnel. A survey by McKinsey found that 70% of digital transformation initiatives fail due to poor integration with existing systems. This issue can slow down the implementation of AI in data quality management, particularly for large organizations with complex legacy systems.
- Bias in AI Algorithms
AI systems are often criticized for their potential to introduce bias into decision-making processes. In the context of data quality, if AI algorithms are trained on biased or incomplete data, they may produce inaccurate results. For example, a dataset that underrepresents certain demographic groups can lead to AI systems that overlook or misclassify important data, affecting the quality of the output. According to a 2023 study by Stanford University, over 30% of AI systems tested showed some form of bias. This challenge underscores the need for diverse, representative datasets to ensure that AI-driven data quality solutions are fair and accurate.
- Scalability Issues
As businesses grow and their data volumes increase, the scalability of AI solutions becomes a critical challenge. While AI can help manage large datasets, it may struggle to maintain high performance and accuracy as data grows in complexity and volume. For businesses with fast-growing data, the AI models used for data quality need to be continually retrained and optimized to handle larger data sets. A report from Gartner predicts that by 2025, 75% of organizations will face scalability challenges with their AI-driven data systems, potentially limiting the effectiveness of AI in data quality management.
- High Implementation Costs
While AI solutions can greatly enhance data quality management, the upfront costs for implementation can be a major barrier, especially for small and medium-sized enterprises (SMEs). Implementing AI tools requires investment in specialized software, skilled personnel, and possibly upgrades to existing IT infrastructure. According to a study by Deloitte, 53% of SMEs reported that the cost of implementing AI solutions was a significant challenge, with many opting for less advanced solutions or delaying adoption altogether due to financial constraints. The high cost of AI implementation remains a persistent challenge, especially for organizations with limited budgets.
Top Opportunities
- Rising Demand for Data-Driven Decision-Making
As businesses across various industries increasingly rely on data-driven decision-making, the demand for accurate and high-quality data is surging. AI-driven data quality solutions are positioned to address this demand by enhancing data accuracy, consistency, and timeliness. According to IDC, the global data analytics market is expected to reach USD 274.3 billion by 2026, with a significant portion of this growth coming from sectors adopting AI for better data management. This trend presents a strong growth opportunity for AI in data quality, as more organizations seek to leverage AI to enhance the reliability of their data for strategic decisions.
- Expansion in the Healthcare Sector
The healthcare sector represents a significant opportunity for AI in data quality. Healthcare organizations manage vast amounts of sensitive data that need to be accurate and up-to-date to improve patient outcomes and comply with regulations. AI technologies are being used to clean, validate, and integrate data from various sources, such as electronic health records (EHR), lab results, and medical imaging systems. The global healthcare data analytics market is projected to reach USD 76.2 billion by 2026, and AI-powered data quality solutions will play a crucial role in enabling this growth by ensuring data integrity and reducing human error in medical data management.
- Increased Adoption of Cloud-Based Solutions
The shift towards cloud-based solutions has created a new wave of growth opportunities for AI in data quality. As more businesses migrate their data to the cloud, ensuring the quality of this data becomes a top priority. AI tools can seamlessly integrate with cloud-based platforms to automate data validation, cleaning, and transformation processes. With the global cloud computing market expected to grow at a CAGR of 15.7%, reaching USD 1,556.5 billion by 2030, AI-driven data quality solutions are well-positioned to capture significant market share as organizations increasingly adopt cloud technologies.
- Growing Regulatory Pressure for Data Accuracy
With the rise of data privacy regulations such as GDPR in Europe and CCPA in California, businesses are under increasing pressure to maintain accurate, secure, and compliant data. AI-powered data quality tools can assist organizations in ensuring they meet regulatory standards by automating data audits, detecting errors, and flagging potential compliance issues. The market for compliance automation tools is expected to grow from USD 5.2 billion in 2023 to USD 15.1 billion by 2030, creating a significant opportunity for AI in data quality solutions.
- AI-Powered Data Governance Initiatives
As organizations prioritize robust data governance frameworks, AI-driven data quality solutions are becoming an essential component of these initiatives. AI can automate data profiling, metadata management, and lineage tracking, ensuring that data remains trustworthy and accessible. According to Gartner, the global data governance market will grow at a CAGR of 24.1% from 2023 to 2028, providing a substantial opportunity for AI in data quality as businesses look to strengthen their data management practices.
Recent Developments
- Launch of DataRobot’s AI-Powered Data Quality Platform (2024)
In 2024, DataRobot, a leading AI-driven data automation company, launched a new AI-powered data quality platform aimed at enhancing data governance and improving the accuracy of machine learning models. The platform leverages machine learning and deep learning algorithms to automatically detect and correct data quality issues, allowing organizations to ensure the integrity of their datasets before using them for predictive analytics. DataRobot’s new offering is expected to drive market growth, helping businesses enhance their decision-making capabilities with clean and accurate data.
- Trifacta Acquired by Alteryx to Boost Data Quality Capabilities (2023)
In 2023, data analytics company Alteryx acquired Trifacta, a leader in data preparation and quality management solutions. This acquisition is aimed at enhancing Alteryx’s AI capabilities to better support businesses in improving their data quality through automated data wrangling and cleaning processes. The acquisition expands Alteryx’s offerings to help companies improve data consistency and reliability for machine learning applications. This move reflects the increasing importance of data quality solutions in the AI landscape and opens up new opportunities for market growth.
- BigID’s Series D Funding to Enhance AI-Based Data Quality Solutions (2024)
BigID, a leader in data intelligence and privacy solutions, secured USD 100 million in Series D funding in 2024 to expand its AI-based data quality solutions. The funding will enable BigID to enhance its platform’s ability to help businesses improve data quality, ensure compliance, and manage data privacy risks effectively. This move signifies growing investor confidence in AI-driven data quality solutions, providing the company with the capital to scale its operations and extend its reach in the global market.
- Informatica Introduces AI-Powered Data Quality Suite (2023)
Informatica, a global leader in cloud data management, introduced a new AI-powered data quality suite in 2023. This suite integrates machine learning algorithms to automate data cleansing, matching, and enrichment processes, improving the overall accuracy of data across enterprises. The suite is designed to help organizations enhance their data governance frameworks while ensuring compliance with data quality standards. Informatica’s AI-powered offering is expected to gain widespread adoption, addressing the increasing need for high-quality data in business intelligence and analytics.
- Dataiku Partners with Microsoft Azure for Enhanced Data Quality (2023)
In 2023, Dataiku, a prominent AI and machine learning platform provider, partnered with Microsoft Azure to integrate its advanced data quality tools with the Azure cloud platform. This collaboration enables businesses to utilize Dataiku’s AI-based data quality solutions directly within the Azure ecosystem, streamlining the process of cleaning and preparing data for analytics. The partnership strengthens the ability of organizations to leverage cloud services while ensuring the integrity of their data, contributing to the overall growth of the AI in data quality market.
Key Player Analysis
- Microsoft Corporation in AI in Data Quality
Microsoft Corporation is making significant strides in the AI-driven data quality market with its Azure cloud platform. In 2023, Microsoft launched new AI-powered data management tools aimed at improving data governance, accuracy, and accessibility. The Azure Purview platform, for instance, includes built-in AI capabilities that automatically detect data quality issues, providing businesses with insights to improve data accuracy and consistency. By integrating these AI features, Microsoft allows organizations to ensure that their data complies with regulatory standards, which is critical for sectors like healthcare, finance, and government. This enhances the overall quality and reliability of data used in analytics, decision-making, and machine-learning models.
- Informatica Inc. in AI in Data Quality
Informatica Inc., a leading data integration and management firm, has been actively advancing its AI-driven data quality solutions. In 2024, the company launched its new “Cloud Data Quality” suite, which integrates AI and machine learning technologies to automate various aspects of data governance, including data profiling, validation, and cleansing. This suite helps businesses quickly detect and resolve data quality issues, ensuring that data used for analytics and decision-making is reliable and accurate. Informatica’s tools are particularly beneficial for industries dealing with large volumes of complex data, such as finance, healthcare, and retail. With its focus on automation and AI, Informatica is positioning itself as a leader in the data quality market, offering scalable solutions to enterprises globally.
- SAP SE in AI in Data Quality
SAP SE is leveraging artificial intelligence to enhance its data quality management capabilities. Through its SAP Data Intelligence platform, the company integrates AI algorithms to provide businesses with intelligent insights into their data, helping to identify and resolve quality issues. This allows organizations to automate tasks like data cleansing, profiling, and enrichment, significantly reducing the time and effort required to maintain high-quality data. SAP’s AI-powered solutions help enterprises across various industries, including manufacturing and logistics, ensure the integrity and accuracy of their data, which is critical for supporting decision-making processes and achieving operational efficiency.
- SAS Institute Inc. in AI in Data Quality
SAS Institute Inc. is making strides in improving data quality with AI-driven tools that provide advanced analytics and automation capabilities. In 2023, SAS enhanced its data management solutions by integrating machine learning algorithms to help organizations better identify and rectify data quality issues. These AI features enable automated data profiling, cleansing, and standardization, which ensures that data is ready for analytics and machine learning projects. SAS has been focusing on industries such as finance, healthcare, and retail, where data accuracy is paramount. With a strong emphasis on automation, SAS is positioning its platform as a valuable asset for businesses looking to improve the efficiency and reliability of their data quality processes.
- Qlik in AI in Data Quality
Qlik, known for its data analytics and business intelligence solutions, has been incorporating AI into its data quality management tools to provide real-time, actionable insights. The company launched an AI-enhanced version of its Qlik Data Catalyst platform, which uses machine learning to automate data quality tasks such as cleansing, cataloging, and profiling. This integration allows businesses to quickly identify anomalies and inconsistencies within their data, helping improve its accuracy and reliability. Qlik’s AI-driven solutions are particularly useful for enterprises looking to scale their data management efforts, providing a seamless approach to handling large volumes of data while ensuring it meets high-quality standards. As data-driven decision-making becomes more critical, Qlik’s AI tools help businesses ensure their data remains trustworthy and actionable.
Conclusion
The AI in Data Quality market is poised for rapid growth as organizations continue to recognize the importance of clean, accurate, and trustworthy data for decision-making, analytics, and machine learning. With the growing reliance on data-driven strategies across industries, companies are increasingly adopting AI-powered solutions to automate and enhance data quality processes.
Major players like Microsoft, Informatica, SAP, and SAS Institute are leading the way in developing advanced tools that leverage AI to identify and resolve data inconsistencies, errors, and redundancies. The market is expected to grow significantly, from USD 0.9 billion in 2023 to USD 6.6 billion by 2033, driven by the growing need for data integrity and governance in sectors like healthcare, finance, retail, and manufacturing.
With a compound annual growth rate (CAGR) of 22.10%, AI in Data Quality is becoming an essential element for organizations seeking to optimize their data assets and achieve competitive advantage in an increasingly data-dependent world.
Discuss your needs with our analyst
Please share your requirements with more details so our analyst can check if they can solve your problem(s)
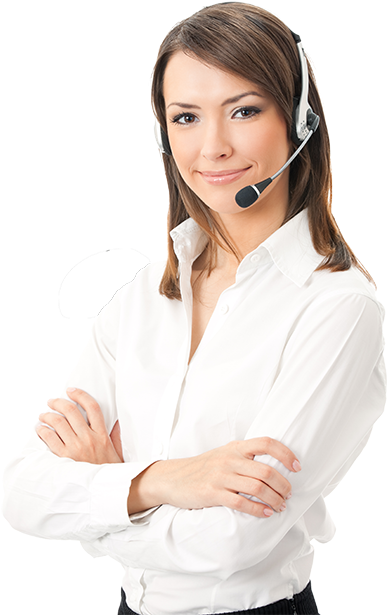