Table of Contents
Introduction
Based on the research findings of Market.us, The Global AI Hardware Market is projected to experience remarkable growth, with its value expected to surge from USD 53.9 billion in 2023 to a substantial USD 833.4 billion by 2033. This impressive expansion corresponds to a CAGR of 31.5% during the forecast period from 2024 to 2033. In 2023, North America took a commanding lead in the market, holding over 35.2% of the market share, equivalent to USD 18.9 billion in revenue.
AI in hardware refers to specialized computer components designed to efficiently run artificial intelligence applications. These components are engineered to accelerate AI processes, enhance efficiency, and minimize energy consumption. The goal is to speed up AI tasks significantly, making it possible for AI systems to operate faster and more effectively. Key components of AI hardware include GPUs (Graphics Processing Units), TPUs (Tensor Processing Units), NPUs (Neural Processing Units), FPGAs (Field-Programmable Gate Arrays), and VPUs (Vision Processing Units), each serving unique roles in various AI tasks like deep learning, neural network processing, and computer vision.
The AI in hardware market is witnessing significant growth, driven by the expanding demand for powerful computing in AI applications across various sectors. The market is projected to reach substantial revenues, highlighting the critical role of AI hardware in technological advancements. This growth is fueled by the increasing integration of AI into devices and systems, pushing the boundaries of innovation and necessitating robust hardware solutions to support complex AI operations.
The major driving factors for the AI in hardware market include the growing complexity of AI algorithms which require specialized processing power, the widespread adoption of AI technologies across different industries, and the increasing need for energy-efficient AI operations. The surge in data-intensive applications like autonomous vehicles, smart robotics, and IoT devices also propels the demand for advanced AI hardware.
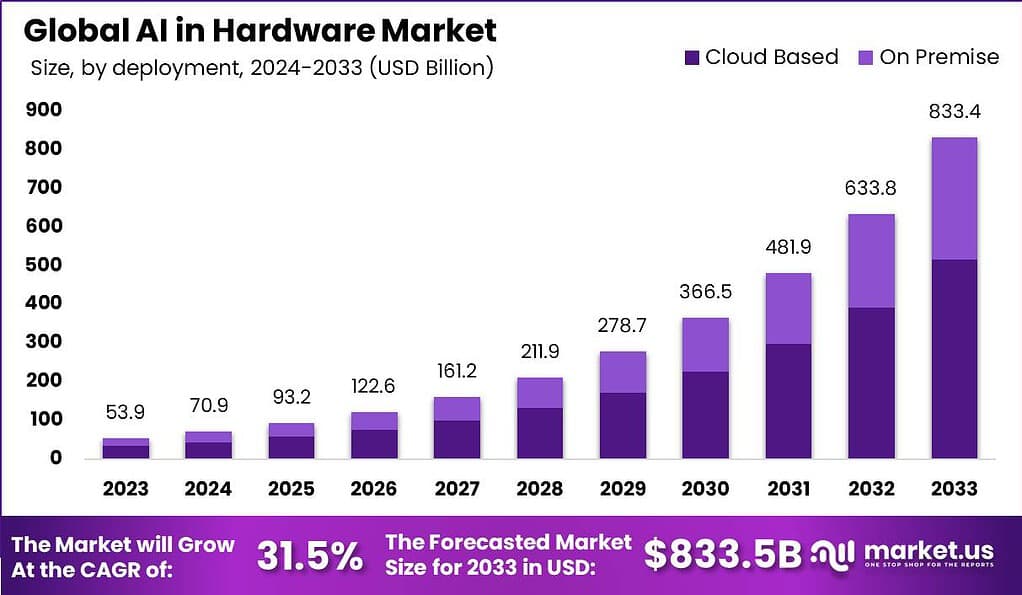
Market demand for AI in hardware is robust, underlined by the critical need for hardware that can keep pace with the rapidly evolving AI software landscape. Industries such as automotive, healthcare, and consumer electronics are increasingly reliant on AI technologies, which in turn drives the demand for specialized AI hardware components that can deliver the necessary computational power and speed.
The AI in hardware market is ripe with opportunities, especially in the development of hardware that can further reduce power consumption while increasing processing speeds. Innovations in chip design, like those seen in TPUs and NPUs, present significant opportunities for hardware manufacturers to contribute to the next wave of AI advancements. Additionally, the push towards more sustainable technology solutions offers a fertile ground for developing new, energy-efficient AI hardware technologies.
Recent technological advancements in AI hardware focus on optimizing the performance and efficiency of AI systems. Innovations in processor design and architecture, such as improvements in TPUs and NPUs, are tailored specifically for handling complex neural networks and deep learning tasks. These advancements not only boost the capabilities of AI systems but also improve their energy efficiency, making them suitable for more widespread and diverse applications.
Key Takeaways
- The AI Hardware Market is projected to soar, reaching USD 833.4 billion by 2033, growing at a solid CAGR of 31.5%. In 2023, the market value stood at USD 53.9 billion.
- When looking at types, processors led the market in 2023, claiming a 37% share due to their central role in powering AI applications and handling complex computations efficiently.
- In terms of deployment, cloud-based solutions are currently in the lead, with 62% of the market share as of 2023. This popularity is driven by businesses prioritizing scalability, storage, and ease of integration that the cloud provides.
- Machine Learning (ML) technology is the dominant force in the market based on technology, capturing 40% share in 2023. ML’s versatility and adaptability have made it a cornerstone for AI-based advancements across various industries.
- For applications, Image and Diagnosis is the top segment, holding 22% of the market share in 2023. This growth reflects the rising use of AI for accurate medical imaging, diagnostic precision, and improved patient outcomes.
- Lastly, by end-user sector, Telecommunication and IT claims the largest market slice at 20% in 2023. The demand here is fueled by the sector’s need to enhance data processing, network management, and AI-powered analytics.
AI in Hardware Statistics
- Global Artificial Intelligence Market is projected to reach a substantial USD 3,527.8 billion by 2033, up from USD 250.1 billion in 2023, demonstrating a robust CAGR of 30.3%. This impressive growth aligns with AI’s increasing integration across sectors, from consumer tech to enterprise solutions, reshaping global economic landscapes.
- AI’s impact on U.S. GDP is expected to surge by 21% by 2030, underscoring its potential to boost economic productivity. Supporting this growth, 64% of businesses anticipate AI-driven productivity increases, with over 60% of business owners confident in AI’s ability to enhance customer relationships.
- Voice search has become a common feature, with half of U.S. mobile users engaging daily. This high adoption rate highlights the significance of natural language processing (NLP) in AI, particularly for applications enhancing user experience and convenience.
- Field-Programmable Gate Array (FPGA) Market is expected to reach USD 39.0 billion by 2033 from USD 10.9 billion in 2023, growing at a CAGR of 13.6%. FPGAs are instrumental in supporting advanced AI models by providing flexible and high-performance processing power, essential for emerging AI workloads.
- Nvidia has established itself as a leader in the AI hardware sector, achieving a valuation of over $1 trillion in early 2023. Key technologies, including the A100 chip and Volta GPU, have proven vital for resource-intensive AI models, particularly in data centers, where large-scale computations are required.
- Intel is aggressively expanding within the AI hardware domain. Its CPUs currently handle 70% of global data center inferencing, indicating a strategic approach to leverage its CPU legacy across AI applications, catering to high-demand data-driven environments.
- Multislice technology stands out as a revolutionary performance-scaling solution, optimizing model efficiency with nearly 60% utilization of floating-point operations per second on TPU v4, pushing the boundaries of AI hardware performance.
- Apple’s M1 chip in MacBooks showcases remarkable advancements with 3.5 times faster general performance and fivefold graphic performance improvement, setting a new standard for consumer AI-capable devices.
- By 2027, 60% of PCs shipped are expected to feature AI capabilities, according to Canalys, reflecting the swift incorporation of AI functionalities in personal computing.
- Global AI Chip Market is projected to soar to USD 341 billion by 2033, up from USD 23.0 billion in 2023, with an accelerated CAGR of 31.2%.
Emerging Trends
- Hybrid AI and On-device Intelligence: There’s a significant shift towards integrating AI capabilities directly into devices such as PCs and mobile phones, facilitated by specialized chipsets like neural processing units (NPUs). This trend enhances devices’ ability to perform AI tasks locally, improving performance, power efficiency, and privacy.
- Open Source and Custom AI Models: The trend towards open-source AI models allows organizations to customize AI solutions tailored to their specific needs without heavy investments in infrastructure. This democratizes AI by making powerful tools accessible to a broader range of users and industries.
- Sustainability in AI: While improving operational efficiency and cost reduction still dominate, there’s a growing emphasis on sustainability. Organizations are increasingly considering AI’s role in energy efficiency and emissions reduction as part of their AI adoption strategy.
- AI-Enhanced Security: As AI technologies become more embedded in devices and systems, ensuring the security and privacy of data handled by these AI systems is becoming paramount. This includes deploying safe and secure AI solutions to protect against potential data breaches and privacy issues.
- Smaller, More Efficient AI Models: There’s a move towards smaller, more efficient AI models due to the increasing costs of cloud computing and hardware. These models are not only necessary to manage costs but also help in addressing privacy and security concerns by running on local devices instead of centralized servers.
Top Use Cases
- Personal Computing: AI capabilities are being integrated into consumer PCs to enable more efficient data processing and enhanced user experiences. This includes features like real-time AI image generation, advanced search functionalities, and language translation, supported by the hardware advancements like those seen in Microsoft’s Copilot+ PCs and Apple’s devices with specialized neural engines.
- Mobile Devices: AI is increasingly used in mobile phones to enhance features such as camera functionality, user interface personalization, and on-device voice processing. These improvements are driven by AI-powered chipsets that enable more powerful processing directly on the device.
- Edge Computing: Small, efficient AI models are suited for edge computing scenarios where data processing needs to happen close to the data source. This minimizes latency and bandwidth use, crucial for real-time applications such as industrial automation and smart city infrastructure.
- Healthcare: AI in hardware is critical for healthcare applications, where it helps in patient monitoring systems and diagnostic tools that require real-time data analysis. This facilitates quicker decision-making directly at the point of care.
- Automotive: AI hardware is transforming the automotive industry by enhancing vehicle automation and safety features. AI capabilities embedded into vehicles enable advanced driver-assistance systems (ADAS) and pave the way for future autonomous driving technologies.
Major Challenges
- Energy Efficiency and System Integration: One of the principal challenges facing AI hardware development is creating energy-efficient systems that can integrate multiple chips or approaches into cohesive, multi-chip heterogeneous systems. This is critical for balancing high throughput with power efficiency in datacenter environments.
- Resource Constraints at the Edge: Devices on the edge of networks, such as IoT devices, face severe resource constraints including power, performance, and bandwidth limitations. This necessitates innovations in on-chip sensing and computing to optimize energy and bandwidth use.
- Memory and Bandwidth Limitations: AI applications are heavily reliant on high-bandwidth memory to process and store data. The industry faces challenges in optimizing memory architecture to meet the demanding requirements of AI computations without escalating costs.
- Thermal Management: As AI hardware pushes the limits of processing power, managing heat generation becomes a critical challenge. Effective thermal management is essential to maintain system stability and performance, especially in compact devices and data centers.
- Complexity in AI Model Deployment: Deploying AI models on hardware involves complex trade-offs between accuracy, latency, and power consumption, which can be particularly challenging when dealing with real-time processing requirements such as in autonomous vehicles or healthcare monitoring systems.
Attractive Opportunities
- Growth in Edge AI: The increasing deployment of AI applications in edge devices presents significant opportunities for the development of specialized AI hardware. These devices require processors capable of handling AI tasks locally, minimizing latency and dependence on cloud services.
- AI Accelerators for Cloud Data Centers: There is a burgeoning demand for AI accelerators like ASICs and FPGAs in cloud data centers. These accelerators are designed to handle specific tasks more efficiently than general-purpose CPUs or GPUs, offering both performance and energy efficiency advantages.
- Advancements in AI for Gaming and Entertainment: The gaming and entertainment sectors are rapidly adopting AI to create more immersive experiences through advanced computer vision, motion tracking, and interactive content. This drives demand for robust AI hardware capable of supporting these sophisticated applications.
- AI-Enabled Autonomous Systems: There is increasing interest in AI-enabled autonomous systems for applications such as autonomous vehicles, drones, and robotics. These systems rely heavily on AI hardware for sensory data processing and decision-making in real-time environments.
- Innovations in Memory Solutions: The AI sector’s growing need for high-speed, high-capacity memory presents opportunities for innovations in memory solutions tailored for AI applications. This includes developments in in-memory computing and advanced memory architectures that can accelerate AI performance while reducing power consumption.
Top 10 Companies
Here’s a summary of the top ten companies in the AI hardware market, each bringing unique innovations and advancements to the field:
- Nvidia: A leader in the AI chip market, Nvidia dominates with its high-performance GPUs widely used across gaming, data science, and AI applications. Its latest innovation, the Blackwell B200 chip, is setting new standards in AI hardware performance.
- Intel: With a longstanding reputation in the semiconductor industry, Intel is making significant strides in the AI hardware space with its new AI chip, Gaudi 3, which promises enhanced training performance and efficiency over its competitors.
- AMD: Known for its powerful CPUs and GPUs, AMD continues to impress in the AI sector with its MI300 AI chip, which competes closely with Nvidia in terms of memory capacity and performance.
- Apple: Apple integrates AI hardware into its consumer devices through the Neural Engine present in the M1 chip, greatly enhancing processing power and efficiency in MacBooks. The forthcoming M3 chip is expected to push these boundaries even further.
- Amazon Web Services (AWS): AWS is expanding its footprint in the AI hardware market with its Trainium and Inferentia chips, designed for training deep learning models and performing high-speed inferencing, respectively.
- Google (Alphabet): Google’s TPU (Tensor Processing Unit) has been a game-changer in the AI space, offering exceptional speed and efficiency for training and inferencing AI models. The latest iterations, including the Trillium TPU, continue to advance Google’s edge in AI hardware.
- IBM: IBM has made significant contributions with its Telum AI chip, which is designed to improve the efficiency of AI computations over traditional CPUs, reflecting IBM’s deep commitment to advancing AI technology.
- Cerebras Systems: Known for creating the largest AI chips, Cerebras’ Wafer Scale Engine chips are designed to handle extremely large and complex AI models, making significant impacts in areas like genomics and deep learning.
- Qualcomm: As a major player in mobile technologies, Qualcomm’s venture into AI hardware with its Cloud AI 100 chip is designed for efficient AI inferencing, highlighting its strategic move into high-performance AI applications.
- Tenstorrent: Although less detailed information was found about Tenstorrent compared to the others, it’s known for developing highly scalable and efficient processors that support the growing demands of AI workloads, positioning it as a notable contender in the AI hardware space.
Recent Developments
- In January 2024, Sidus Space made strategic advancements in hardware contracts and AI applications, setting the stage for a significant milestone with the upcoming launch of LizzieSat-1. Slated for March 2024 on SpaceX’s Transporter-10 mission, LizzieSat-1 will be the first satellite in Sidus’ planned constellation, launching from Vandenberg Space Force Base in California. This launch marks a critical step in Sidus Space’s strategy to expand its data-as-a-service offerings.
- At Intel’s AI Everywhere event in December 2023 in New York, Intel introduced its latest AI hardware, including the 5th Gen Intel Xeon and Intel Core Ultra. These additions to Intel’s AI product line demonstrate its commitment to staying competitive in the AI hardware market, solidifying its position among top industry players with a more robust AI hardware portfolio.
- In December 2023, rabbit inc., an emerging AI-focused start-up, secured $10 million in a Series A funding round. This funding will drive the development of rabbit inc.’s standalone AI-capable hardware, as the company aims to reshape the human-machine interface and broaden its reach in the AI technology landscape.
Conclusion
In conclusion, the AI in hardware market is poised for significant growth, driven by the escalating demand for AI capabilities across various sectors. The specialized hardware designed for AI tasks is not just enhancing the efficiency and speed of AI applications but is also playing a crucial role in advancing technological innovations. With the increasing complexity of AI algorithms and the expansion of AI into new domains such as autonomous vehicles and smart devices, the need for robust AI hardware is more critical than ever.
Opportunities abound for developments in energy efficiency and processing power, promising to push the boundaries of what AI can achieve. As industries continue to integrate AI more deeply into their operations, the AI in hardware market is expected to remain a vital component of the technological landscape, fostering significant advancements and offering substantial economic potential.
Explore More Reports
- Customer Experience Management Market to hit USD 57.9 Bn By 2033
- Device as a Service Market to Reach USD 3,075.6 Bn by 2033
Discuss your needs with our analyst
Please share your requirements with more details so our analyst can check if they can solve your problem(s)
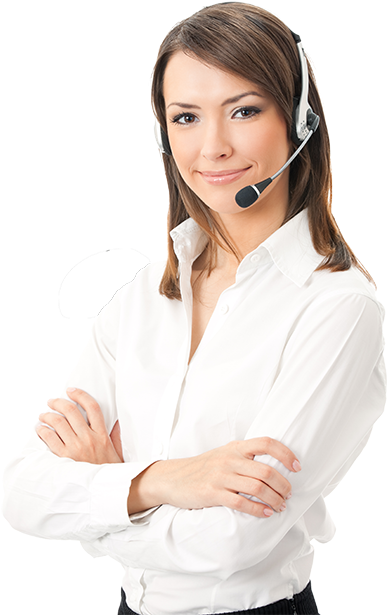