Table of Contents
Introduction
According to Market.us, The global AI in Predictive Maintenance market is projected to grow significantly, with its value expected to reach approximately USD 2,306.2 million by 2033, up from USD 722.9 million in 2023. This growth represents a compound annual growth rate (CAGR) of 12.3% over the forecast period from 2024 to 2033.
The AI in Predictive Maintenance Market is experiencing significant growth due to the increasing adoption of IoT devices, the rise in demand for asset management solutions, and the need to reduce unplanned outages. As industries continue to digitize and embrace Industry 4.0, the market is expected to expand further, with advancements in AI technology driving innovations in predictive maintenance strategies, making it a critical component of modern industrial operations.
The growth of AI in Predictive Maintenance is driven by several key factors. One of the most significant is the increasing adoption of the Internet of Things (IoT) across various industries. IoT devices generate vast amounts of data from machinery and equipment, which AI algorithms can analyze to predict potential failures. Additionally, the growing need to reduce operational costs and improve efficiency is pushing organizations to invest in AI-powered predictive maintenance solutions. The rise in demand for asset management and the integration of AI with existing enterprise systems are also contributing to market growth, as companies seek to extend the lifespan of their assets and avoid costly unplanned downtime.
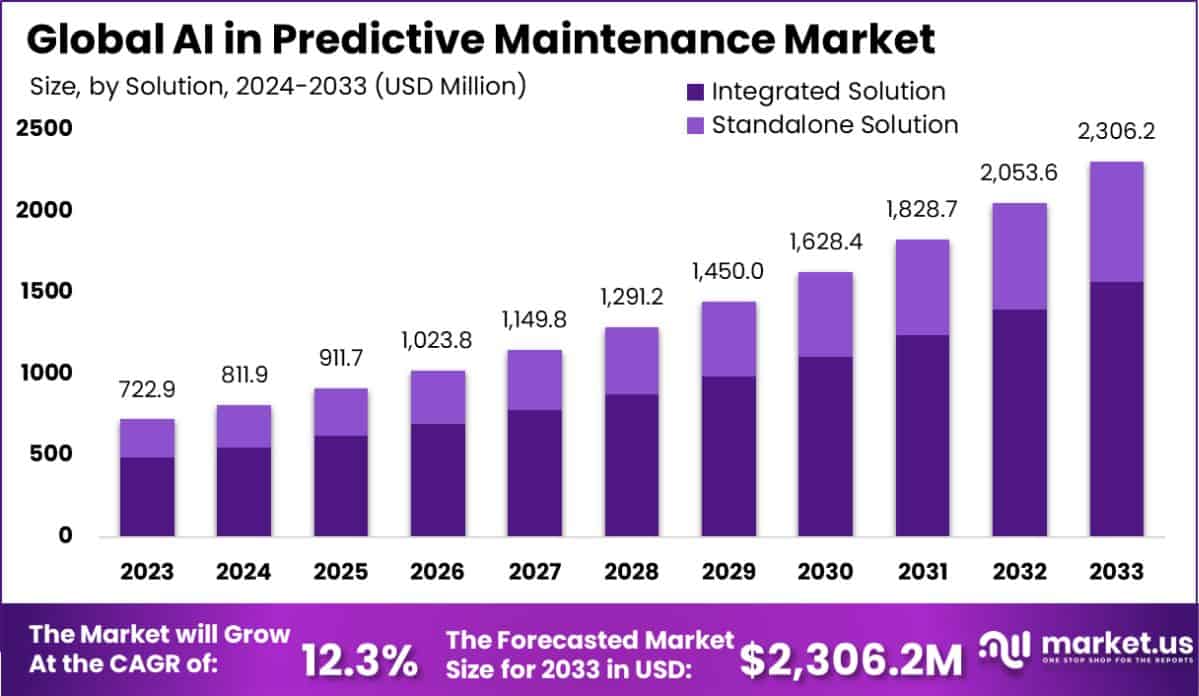
Opportunities in the AI in Predictive Maintenance market are abundant as industries continue to digitize and adopt advanced technologies. One promising opportunity lies in the expansion of AI applications beyond traditional industries such as manufacturing and energy, into sectors like healthcare, transportation, and retail, where predictive maintenance can play a crucial role in ensuring the reliability of critical systems. Moreover, the development of more sophisticated AI algorithms and the increasing use of cloud-based platforms present opportunities for vendors to offer scalable and cost-effective predictive maintenance solutions.
Key Takeaways
- The global AI in Predictive Maintenance market is projected to reach approximately USD 2,306.2 million by 2033, up from USD 722.9 million in 2023, with a compound annual growth rate (CAGR) of 12.3% during the forecast period from 2024 to 2033.
- In 2023, the Integrated Solution segment dominated the AI in Predictive Maintenance market by solution type, accounting for over 68% of the market share.
- The Manufacturing sector led the industry segment in 2023, capturing more than 25% of the AI in Predictive Maintenance market share.
- North America is expected to lead the market, holding a 36% share in 2023, with revenue of USD 260.2 million from the AI in Predictive Maintenance market.
AI in Predictive Maintenance Statistics
- The Global Artificial Intelligence Market is forecasted to reach an estimated USD 2,745 billion by 2032, up from USD 177 billion in 2023, marking a significant compound annual growth rate (CAGR) of 36.8% from 2024 to 2033.
- The Global Predictive Maintenance Market is projected to expand to around USD 107.3 billion by 2033, starting from USD 8.7 billion in 2023, with a growth rate of 28.5% during the period from 2024 to 2033.
- The Global Industry 4.0 market is anticipated to grow to USD 482 billion by 2032, from USD 93 billion in 2023, achieving a CAGR of 20.7% over the forecast period from 2023 to 2032.
- The Global Industry 5.0 Market is expected to increase to USD 964 billion by 2033, from USD 135 billion in 2023, with a CAGR of 21.7% from 2024 to 2033.
- AI-driven predictive maintenance has demonstrated effectiveness in reducing equipment downtime by 30% to 50% and extending the lifespan of machinery by 20% to 40%. Utilizing AI for predictive maintenance significantly reduces breakdowns by 70%, boosts productivity by 25%, lowers maintenance costs by 25%, decreases unplanned downtime by 30%, accelerates service resolutions by 83%, and cuts time on site by 75%.
- According to Deloitte, AI-driven anomaly detection and fault prediction in predictive maintenance provide considerable advantages, such as increasing runtime by 10% to 20%, slashing maintenance costs by up to 10%, and reducing the time required for maintenance scheduling by up to 50%.
- For establishments focusing on preventive and predictive maintenance, this approach is linked with 15% less downtime, an 87% lower defect rate, and 66% fewer inventory increases due to maintenance issues. Additionally, AI-enhanced predictive maintenance results in a 30% reduction in unplanned downtime, 83% faster service resolutions, and 75% less time needed on site.
Why Manufacturers Should Invest in Predictive Maintenance?
According to survey, machine failures account for approximately 15% of all downtime in pharmaceutical manufacturing. However, our customer data indicates that machine failures can represent up to 25% of downtime within the pharmaceutical industry (as illustrated in the image below). It’s important to highlight that in the pharmaceutical sector, the primary cause of downtime is often changeovers, driven by stringent regulatory requirements for thorough line cleaning. In comparison, equipment failures in other industries may contribute to more than 25% of total downtime.
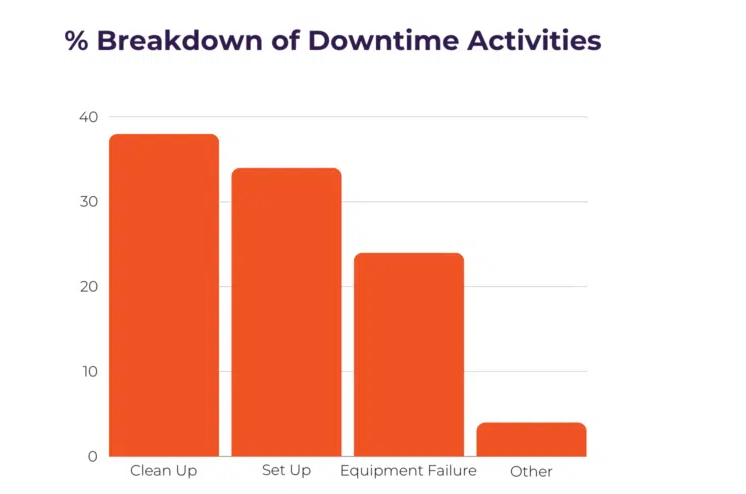
Source: scw.ai
Emerging Trends
- Increased Use of IoT and Edge Computing: In 2024, the integration of AI with IoT and the expansion of edge computing are significant trends. This combination allows for real-time data processing and quicker decision-making directly at the data source, enhancing the efficiency of predictive maintenance operations.
- Advancement in Machine Learning for Anomaly Detection: Machine learning technologies are increasingly refined to better detect anomalies and predict equipment failures, thus reducing downtime and maintenance costs.
- Digital Twins and Immersive Technologies: The use of digital twins is becoming more prevalent. These virtual replicas of physical systems allow for continuous monitoring and simulation, providing insights that lead to more proactive maintenance strategies. Additionally, immersive technologies like augmented and virtual reality are being incorporated to facilitate complex maintenance tasks.
- Predictive Analytics and AI Models: Predictive analytics continue to evolve, leveraging advanced algorithms and machine learning to analyze vast amounts of data and predict equipment failure before it happens.
- Service Models and Blockchain Integration: New business models such as Predictive Maintenance as a Service (PdMaaS) are emerging, offering predictive maintenance capabilities as cloud-based solutions. Moreover, blockchain technology is being explored for its potential to enhance data security and integrity in maintenance operations.
Top Use Cases
- Manufacturing and Industrial Applications: AI-driven predictive maintenance is particularly valuable in manufacturing, where it can significantly reduce downtime and maintenance costs while improving equipment effectiveness and safety.
- Transportation and Fleet Management: In transportation, predictive maintenance helps in monitoring vehicle health and predicting failures, which is crucial for reducing operational delays and maintenance costs.
- Energy and Utilities: AI is used to predict equipment failures in power plants and grid systems, enhancing the reliability of energy supply and optimizing maintenance schedules for critical infrastructure.
- Healthcare Equipment Maintenance: Predictive maintenance is critical in healthcare, where equipment reliability can directly impact patient care. AI helps in ensuring that medical equipment operates efficiently without unexpected failures.
- Smart Buildings and Infrastructure: AI enables predictive maintenance for HVAC systems and other critical building infrastructure, leading to energy savings, improved building management, and reduced operational costs.
Major Challenges
- Data Quality and Integration: Effective predictive maintenance relies heavily on high-quality, integrated data from various sources. Ensuring data accuracy, reliability, and accessibility is crucial for AI algorithms to provide meaningful insights.
- High Initial Investment: The implementation of AI-driven predictive maintenance systems often requires significant upfront investments in technology, sensors, and software. Organizations need to balance these costs with the potential long-term benefits.
- Skill Gaps: There is a critical need for skilled personnel who can manage and interpret the complex data produced by AI systems. Training employees to effectively use these tools is essential for the successful deployment of predictive maintenance strategies.
- Change Management: Transitioning from traditional to AI-driven maintenance methods involves significant cultural and operational shifts within organizations. Effective change management is necessary to ensure that all stakeholders are aligned and the new systems are adopted smoothly.
- Scalability and Adaptability Challenges: As predictive maintenance systems scale, they must remain adaptable to changing conditions and new types of equipment. This requires continuous updates and refinements to the AI models and systems in place.
Top Opportunities
- Reduction in Operational Costs: AI-enabled predictive maintenance can significantly reduce maintenance costs by allowing businesses to perform maintenance only when needed, rather than on a scheduled or reactive basis. This can decrease downtime and increase operational efficiency.
- Extended Equipment Lifespan: By predicting when maintenance is needed, AI helps extend the lifespan of equipment, ensuring that machinery operates efficiently for longer periods. This not only saves money on replacements but also improves overall asset management.
- Improved Safety and Compliance: AI-driven predictive maintenance can enhance safety by predicting potential equipment failures before they occur, thereby reducing the risk of accidents. It also helps ensure compliance with various regulatory requirements by maintaining equipment in optimal condition.
- Enhanced Decision Making: With AI, companies can make more informed decisions about maintenance activities, which can lead to better resource allocation and prioritization of maintenance tasks based on actual equipment condition rather than fixed schedules.
- New Business Models: There is an opportunity for the development of new business models such as Predictive Maintenance as a Service (PdMaaS), where companies offer predictive maintenance capabilities as cloud-based solutions. This can open up predictive maintenance to a wider range of businesses, including small and medium-sized enterprises that might not have been able to afford the initial investment.
Recent Developments
- Radix Engineering and Software – In 2023, Radix expanded its AI-driven predictive maintenance solutions across new industrial sectors, including oil and gas. This expansion aligns with the growing demand for real-time monitoring and AI analytics to predict equipment failures before they occur.
- KCF Technologies, Inc. – In November 2023, KCF Technologies launched an upgraded version of its predictive maintenance platform, SMARTdiagnostics. The update includes enhanced vibration monitoring and condition monitoring capabilities, which are crucial for detecting early signs of equipment failure.
- ONYX Insight – In early 2024, ONYX Insight announced a strategic partnership with several wind energy companies to integrate its predictive maintenance software into their operations. This partnership aims to reduce maintenance costs and improve the reliability of wind turbines through AI-driven insights.
- PROGNOST Systems GmbH – In 2023, PROGNOST Systems released a new version of its predictive maintenance software specifically designed for rotating machinery. The update includes advanced machine learning algorithms that improve fault detection accuracy, reducing downtime and maintenance costs for users.
- OCP Maintenance Solutions: In January 2024, OCP Maintenance Solutions announced a significant partnership with Nexans, a leader in cabling and connection solutions. This collaboration aims to integrate mechanical and electrical expertise, paving the way for innovative predictive maintenance solutions that enhance operational efficiency and reliability across industries.
- Gastops: In September 2023, Gastops secured a deal to supply its ChipCHECK systems to Bell Textron Canada for the Royal Canadian Air Force’s CH146 Griffon helicopters. This move enhances maintenance capabilities and reduces operational costs by improving equipment readiness and streamlining maintenance processes.
- KCF Technologies: KCF Technologies continues to advance its predictive maintenance solutions by expanding its SMARTdiagnostics platform. This platform now includes enhanced vibration monitoring and motor current signature analysis, providing comprehensive machine health insights to prevent equipment failures.
- Infinite Uptime: In August 2024, Infinite Uptime launched Nity, an AI-driven conversational tool designed for large-scale asset condition monitoring. Nity enhances decision-making by swiftly providing performance data and predictive insights, helping companies optimize their maintenance strategies.
Conclusion
In Conclusion, The predictive maintenance market, while facing hurdles like high initial investment and the need for skilled professionals, holds a crucial position in modern industrial strategies. This market is marked by its significant benefits such as substantial cost savings and enhanced operational efficiency, which help businesses mitigate unexpected failures and maintain continuous production flows. As technological advancements in IoT and AI continue to evolve, they drive the predictive maintenance sector forward, making it indispensable for industries aiming to remain competitive and future-ready. Thus, the sector’s growth and ongoing innovation not only underscore its importance but also highlight its potential to revolutionize industrial practices globally.
Discuss Your Needs With Our Analyst
Please share your requirements with more details so our analyst can check if they can solve your problem(s)
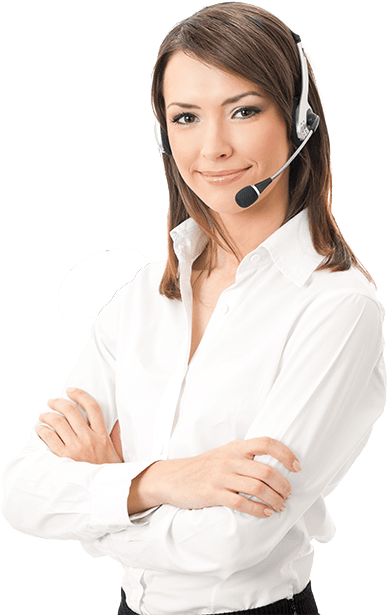