Table of Contents
Introduction
The Global AI Training Dataset Market has shown remarkable growth and potential in recent years. It was valued at USD 2.3 billion in 2023 and is expected to grow at a compound annual growth rate (CAGR) of 20.5% from 2023 to 2032, reaching USD 11.7 billion by 2032. This growth is due to the increasing demand for AI across various sectors, necessitating high-quality datasets for training machine learning algorithms.
The surge in AI and machine learning applications across different industry verticals, which has led to an elevated demand for training datasets, is one of the key drivers of this market’s growth. Technological advancements and innovations, such as ChatGPT, have revolutionized dataset creation, significantly reducing the time and resources required for constructing extensive datasets for NLP models.
However, the market faces some challenges such as the high cost of dataset integration and infrastructural limitations in underdeveloped countries, which impede market growth. Moreover, the market needs to develop more accurate and unbiased datasets to ensure AI’s broader applicability and acceptance.
The AI Training Dataset sector has seen a flurry of mergers, acquisitions, and funding activities, highlighting the industry’s vibrant dynamism and growth potential. Notable transactions include Nextiva’s acquisition of Simplify360, an Indian AI customer experience platform that brings advanced AI and automation capabilities to Nextiva, serving over 5,000 businesses globally, including prestigious clients like Amazon and Honda. McKinsey’s acquisition of Iguazio enhances McKinsey’s QuantumBlack with industry-specific AI solutions, promising more productivity, speed, and reliability. Amazon has expanded its AI portfolio by acquiring Snackable.
AI, aiming to enrich Amazon Music’s podcast features through advanced audio-focused AI. Snowflake’s purchase of Myst AI, specializing in AI-based time series forecasting, marks a strategic extension into machine learning for Snowflake. Deloitte’s acquisition of SFL Scientific further strengthens its standing as an AI industry leader, blending deep science and analytics knowledge with Deloitte’s vast AI capabilities. VideoVerse’s acquisition of Reely.ai, BioNTech’s acquisition of InstaDeep, and Pinterest’s purchase of THE YES are other noteworthy developments that reflect the sector’s ongoing evolution and the strategic importance of AI technologies across various applications.
The AI startup ecosystem, especially in India, has faced a complex funding landscape influenced by global economic conditions and investment trends. There has been a noticeable shift towards more mature funding rounds, indicating investors’ preference for ventures with proven business models amid economic uncertainties. Uniphore’s recent $400 million series E funding highlights the sector’s potential for innovative conversational AI solutions. Despite challenges like rising interest rates and recession fears, the AI startup sector continues to attract significant investments, driven by the transformative power of AI technologies across various industries.
Key Takeaways
- The Global AI Training Dataset Market was valued at USD 2.3 billion in 2023.
- It’s estimated to achieve a remarkable CAGR of 20.5% between 2023 and 2032.
- By 2032, the market is anticipated to reach a staggering USD 11.7 billion.
- North America commands a significant revenue share of 35.8% in the AI training dataset market.
- Google LLC, Microsoft Corporation, and Amazon Web Services Inc. are some key players in the AI training dataset market.
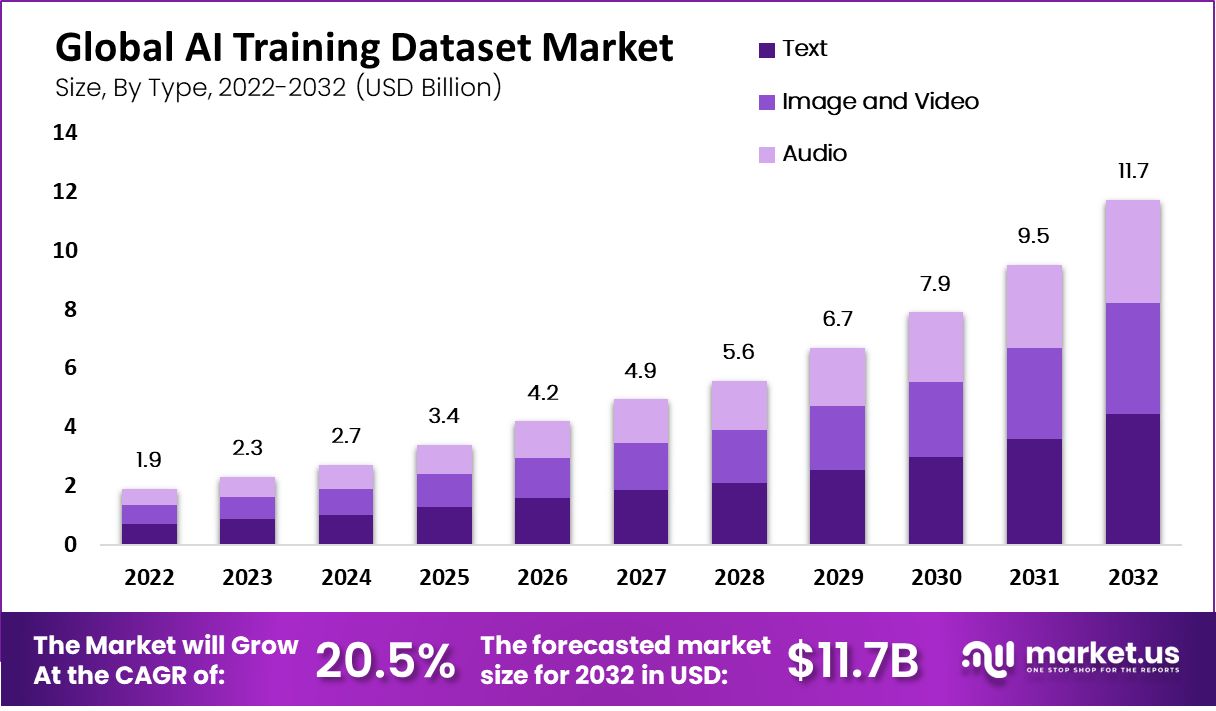
AI Training Dataset Statistics
- AI training data acts as the “teacher” for algorithms, with datasets serving as collections of data points.
- There are two main types of AI training data: structured and unstructured.
- Structured data is organized and easy to sift through, like spreadsheets.
- Unstructured data includes text, images, and audio, which are more challenging to process.
- The quality of AI training data directly influences the performance and reliability of machine learning models.
- Data annotation is crucial for ensuring the accuracy and usability of training data.
- Relevant AI training data must align with the specific goals of a project.
- A focus on quality over volume is essential, as a well-curated dataset is more valuable than a vast, unfiltered one.
- Diverse datasets help in creating unbiased AI models.
- Legal and ethical considerations, such as GDPR, play a significant role in data handling and privacy.
- The average estimated revenue size of companies acquired in the AI sector in 2022 was $6 million across various sectors.
- The aggregate estimated revenue of these acquired companies in 2022 was $202 million.
- The vision datasets’ scale ranged from 2e3 to 3e9, with a yearly growth rate of approximately 0.09.
- Speech datasets showed a vast scale range from 9e2 to 3e12, with a higher yearly growth rate of approximately 0.21.
- Game-related datasets varied between 7e5 to 4e11, sharing a similar growth rate to vision datasets.
- Recommendation datasets varied from 1e8 to 1e10, showcasing the smallest growth rate among the categories mentioned.
- Drawing datasets showed a significant scale variance from 6e4 to 4e9, indicating substantial growth potential.
- Vision and language datasets have undergone significant growth, particularly noted after 2014-2015, marking a transition to much larger datasets than were previously common.
- The largest language dataset observed was the FLAN dataset at 1.87e12 words.
- Training datasets for language models have experienced a growth of 0.23 orders of magnitude per year since 1990, amounting to a total growth of 7 orders of magnitude by 2022.
- Vision, speech, and language domains have shown trends of increasing dataset sizes over time, with a notable transition to larger datasets after the mid-2010s.
Use Cases Of AI Training Dataset
- Computer Vision for Automotive Applications: The Apollo Open Platform provides a rich autonomous driving dataset, including HD maps with geometric & semantic metadata, crucial for developing more accurate perception algorithms for self-driving vehicles.
- Medical Imaging: Training datasets are indispensable for medical imaging applications, including X-Ray, CT, MRI, etc., supporting computer vision models in accurately predicting medical conditions from imaging data.
- Real Estate and Urban Planning: AI models trained on datasets containing property data, mortgage information, urban planning data, and more can significantly enhance market analysis, valuation models, and investment strategies in the real estate sector.
- E-commerce and Retail: Transaction data, including B2B transactions, sales data, and consumer behavior data, are used to train AI models to predict market trends, optimize inventory, and personalize shopping experiences.
- Content Moderation in Social Media: Training datasets containing web data, including IP addresses, web browsing data, and sentiment data, help AI models identify and moderate inappropriate content across digital platforms, enhancing user experience and safety.
- Healthcare Analytics: AI training datasets in healthcare, including electronic health records (EHR), patient data, and medical claims data, play a critical role in developing predictive models for patient care, treatment outcomes, and operational efficiency.
- Financial Services: In finance, AI models trained on datasets such as bank transaction data, electronic payment data, and credit scoring data are used for fraud detection, risk assessment, and customer service automation.
- Entertainment and Media: AI models use datasets from streaming data, sports data, and event data to personalize content recommendations, optimize streaming quality, and analyze audience engagement trends.
Recent Developments
- Nextiva acquired Simplify360, an AI customer experience platform from India, aiming to level the playing field for businesses by making customer support delivery easier through AI and automation.
- McKinsey acquired Iguazio, a leader in artificial intelligence and machine learning, to enhance its QuantumBlack platform with industry-specific AI solutions, marking a significant enhancement in McKinsey’s AI offerings.
- Amazon acquired Snackable.AI, specializing in audio-focused AI, to enrich user features for its podcast offerings on Amazon Music, highlighting the e-commerce giant’s ongoing efforts to integrate AI-powered features across its business.
- Snowflake planned to acquire Myst AI, specializing in AI-based time series forecasting, as part of its strategy to integrate machine learning into its data cloud services, indicating a growing interest in advanced forecasting technology.
- Deloitte announced the acquisition of SFL Scientific, a leading AI strategy and data science consulting firm, aiming to bolster its position as an industry leader in AI and drive AI-fueled transformations across various industries.
- VideoVerse announced its acquisition of Reely.ai, a US-based Esports company with AI-powered technology for content creation and distribution, indicating a strategic move to enhance offerings in the gaming and esports sectors.
- BioNTech acquired InstaDeep, a prominent technology company in AI and ML, to integrate validated AI- and ML-based models into its discovery platforms, aiming to accelerate the development of next-generation immunotherapies and vaccines.
- Pinterest announced it would acquire THE YES, an innovative AI-based shopping platform for fashion, expected to bolster Pinterest’s position as a leading platform for taste-driven shopping.
- Google’s significant AI and Data Science acquisitions in recent years include Kaggle, Halli Labs, AIMatter, Onward, Alooma, and Looker, each contributing to Google’s aim to attain technology supremacy and propel success boundaries in AI and data science.
- The AMD acquisition of Xilinx for $28.3 billion in an all-stock deal aimed to give AMD access to a portfolio of high-leadership computing, graphics, and adaptive SoC products, although AMD’s stock price saw a significant decline post-announcement.
- The Prologis merger with Duke Realty valued at $26 billion brought together two of the world’s major logistics real estate companies, significantly expanding Prologis’ portfolio and establishing it as the world’s largest logistics real estate operator.
- The merger between Orange and Grupo MásMóvil created a new leader in Spain’s cellular telephone market, aiming for scale and combined financial muscle for investments in 5G and fiber.
Conclusion
The AI Training Dataset Market holds a promising future, with its growth fueled by the widespread adoption of AI technologies, ongoing technological innovations, and the strategic expansion of market players into emerging markets. Despite facing challenges, the market’s potential for creating more refined and unbiased training datasets presents lucrative opportunities for growth and innovation.
Discuss your needs with our analyst
Please share your requirements with more details so our analyst can check if they can solve your problem(s)
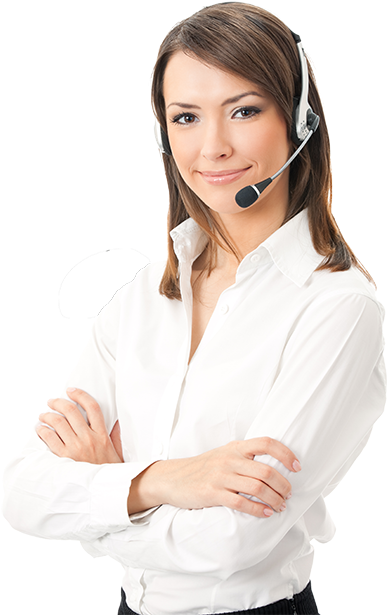