Table of Contents
Introduction
Data Science Platform Statistics: A Data Science Platform (DSP) is a software ecosystem that facilitates the entire data analysis process.
It integrates data from diverse sources, prepares and cleans the data, enables exploration, builds predictive models, and assesses their performance.
DSPs streamline and enhance efficiency in data-driven decision-making, making them valuable tools for professionals in various industries.
Some popular DSPs include IBM Watson Studio, DataRobot, Databricks, Google Cloud AI Platform, and Microsoft Azure Machine Learning, each offering tailored features and integrations.
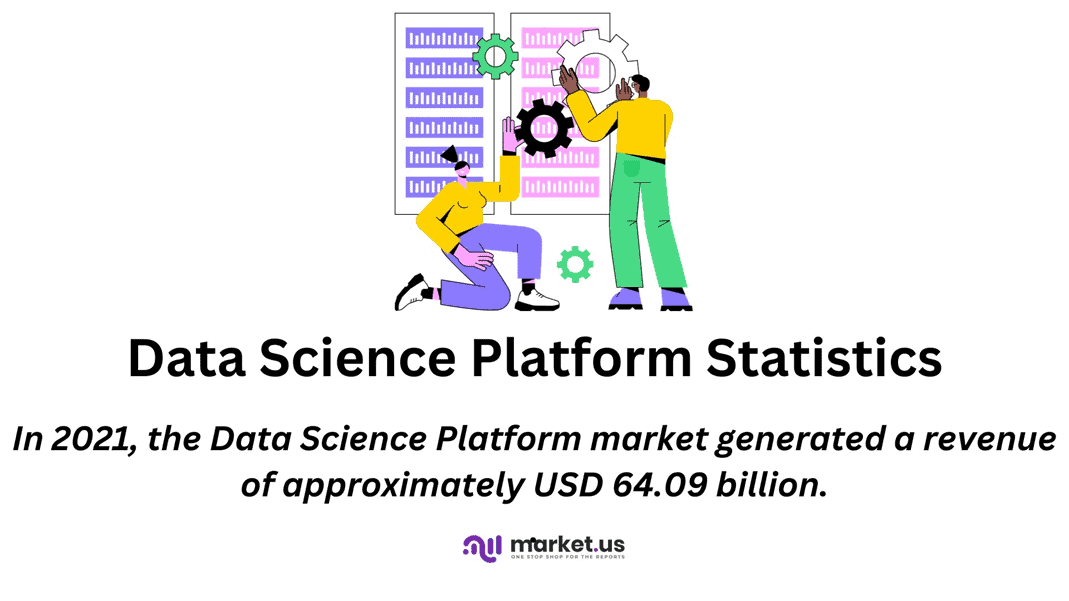
Editor’s Choice
- The Data Science Platform market has been experiencing robust and consistent growth over the past few years at a CAGR of 25.7%.
- In 2021, the market generated a revenue of approximately USD 64.09 billion.
- North America leads the way with the largest market share, accounting for a significant 38.0% of the total market.
- Google LLC holds a significant market share, accounting for 17% of the total market.
- Saturn Cloud serves as a data science platform that supports Python, R, and Julia for both teams and individuals.
- Among programming languages, Python stands out as the dominant choice, commanding a significant share of 27.91%.
- According to Gartner’s predictions, by 2025, approximately 75% of the data generated by enterprises will be generated and managed outside of the conventional data centers and cloud infrastructure.
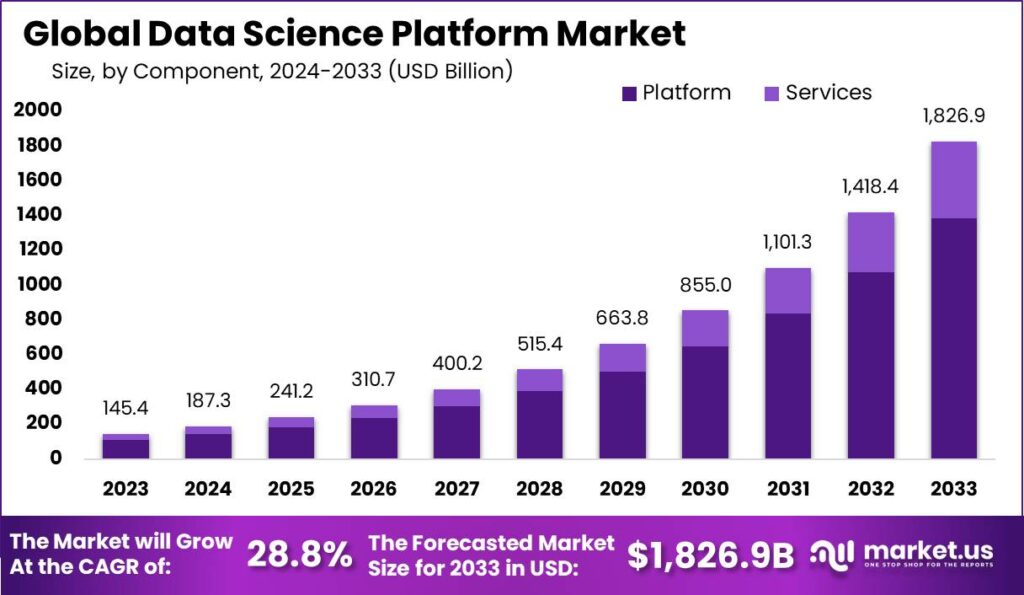
Data Science Platform Market Overview
Global Data Science Platform Market Size Statistics
- The Data Science Platform market has been experiencing robust and consistent growth over the past few years at a CAGR of 25.7%. Reflecting the growing significance of data analytics and data-driven decision-making across industries.
- In 2021, the market generated a revenue of approximately USD 64.09 billion, indicating a strong demand for data science tools and platforms to extract insights from the ever-expanding volume of data.
- This growth momentum continued into 2022, with the market reaching a revenue of USD 89.79 billion. Demonstrating a substantial increase in investment in data science capabilities.
- Looking ahead, the market is expected to maintain its upward trajectory. Projections suggest that in 2023, the Data Science Platform market will achieve revenues of approximately USD 115.49 billion. Showcasing the increasing adoption of these platforms for data analysis, machine learning, and predictive modeling.
- As organizations recognize the value of data-driven insights, they are willing to invest more in advanced analytics solutions.
- In subsequent years, the market is forecasted to continue its expansion. With revenues reaching USD 141.19 billion in 2024 and USD 166.89 billion in 2025.
- This steady growth highlights the essential role that data science platforms play in helping businesses gain a competitive edge through data-driven decision-making, predictive analytics, and improving overall operational efficiency.
- Looking further into the future, the Data Science Platform market is expected to reach remarkable milestones. By 2032, it is projected to achieve a substantial revenue of USD 346.79 billion.
(Source: Market.us)
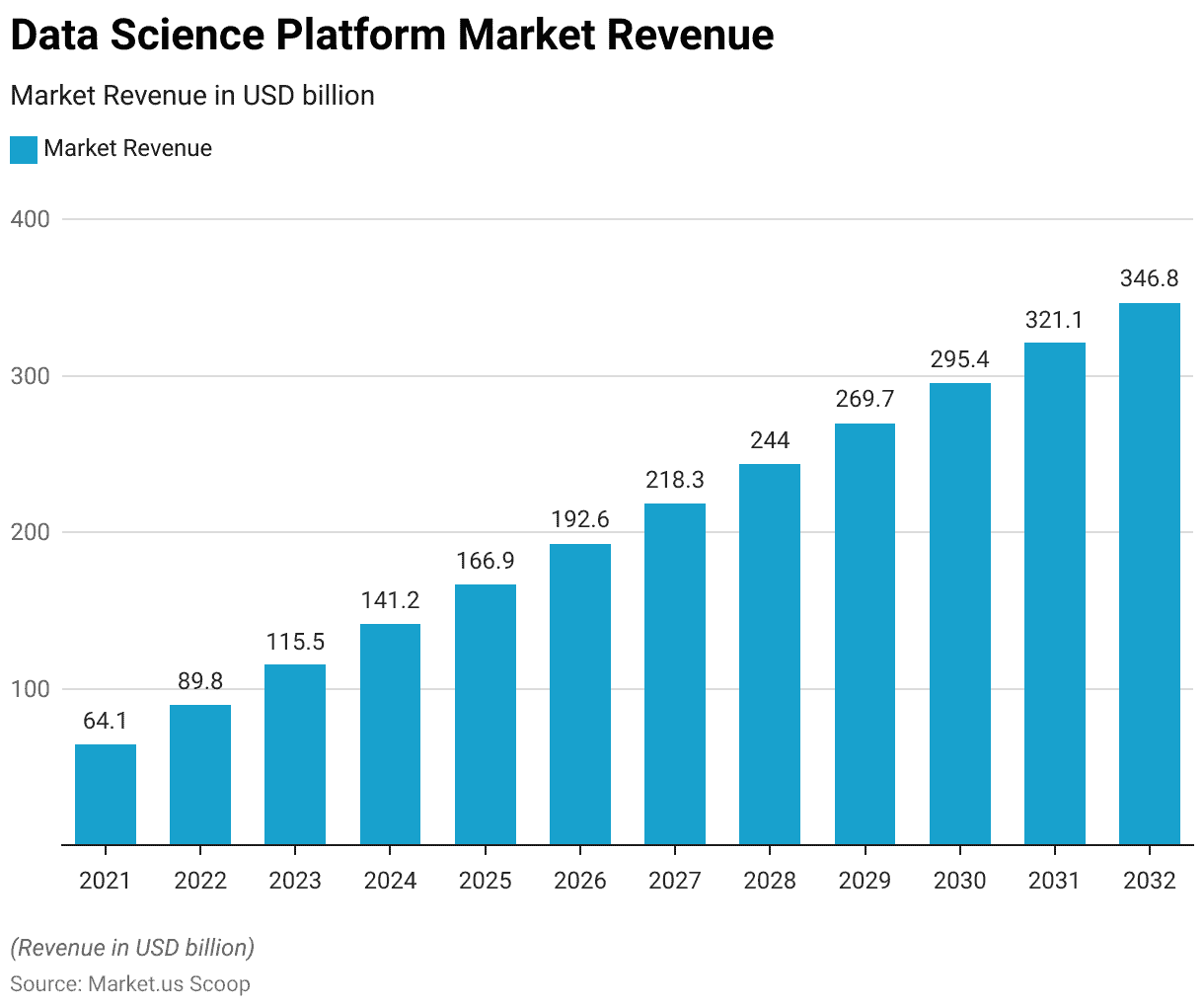
Regional Analysis of the Data Science Platform Market Statistics
- The global Data Science Platform market exhibits a distribution of market share across different regions. Reflecting varying degrees of adoption and investment in data science technologies.
- North America leads the way with the largest market share, accounting for a significant 38.0% of the total market.
- This dominance is indicative of the region’s advanced data science infrastructure and the widespread integration of data analytics into businesses and industries.
- Europe follows closely behind, contributing 24.0% of the market share, underlining the continent’s growing emphasis on data-driven decision-making and innovation.
- In the Asia-Pacific (APAC) region, there is a substantial market presence, capturing 22.0% of the market share. APAC’s inclusion highlights its emergence as a key player in the global data science landscape. Driven by the adoption of digital technologies and a burgeoning interest in data analytics.
- South America accounts for 9.0% of the market share, demonstrating a notable but smaller presence, while the Middle East and Africa (MEA) contribute 7.0% to the overall market share.
- These figures reflect a growing global recognition of the pivotal role data science platforms play in shaping business strategies. Enhancing competitiveness, and driving innovation across diverse geographical regions.
(Source: Market.us)
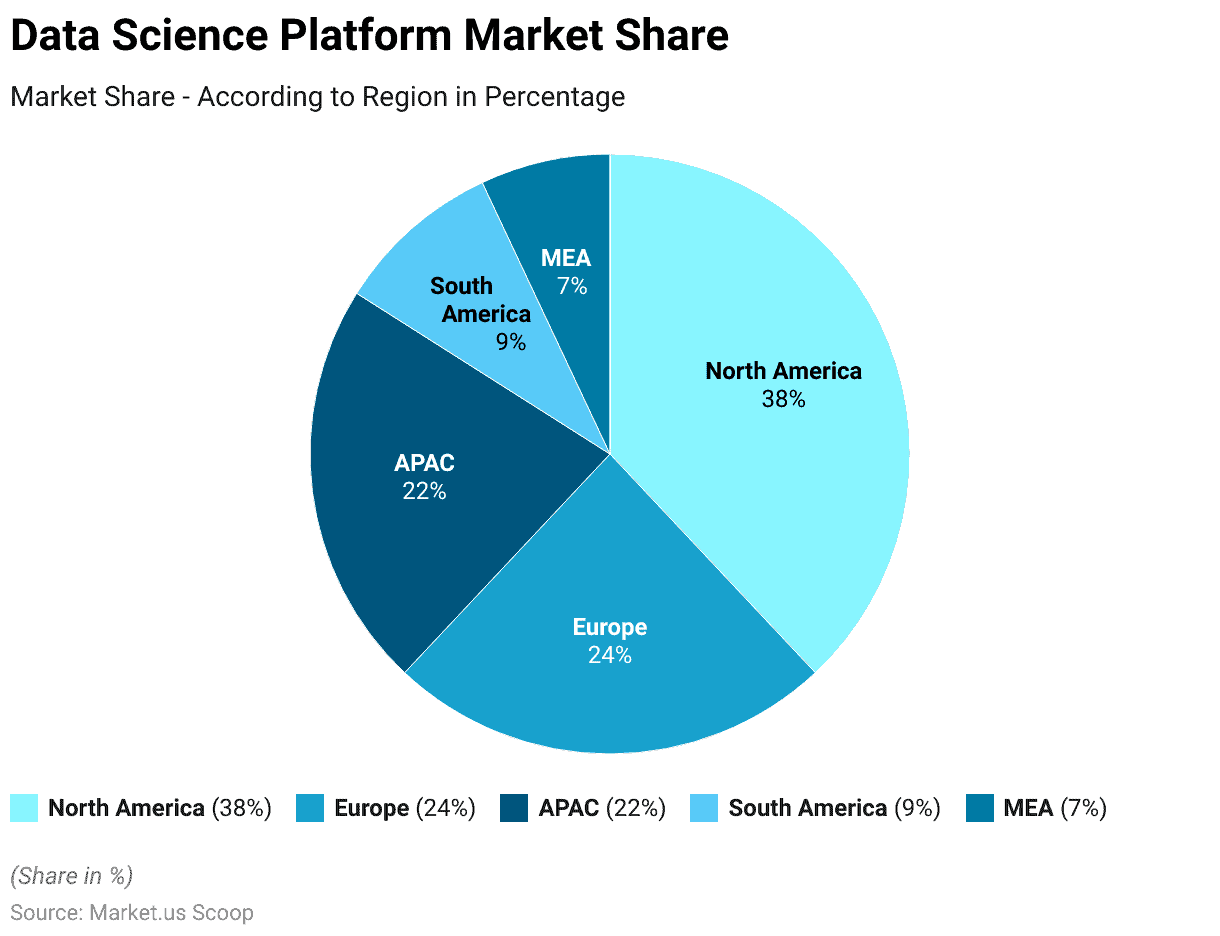
Key Players in the Data Science Platform Market Statistics
- In the highly competitive landscape of the Data Science Platform market. Several key players have emerged, each contributing to the industry’s growth and evolution.
- Notably, Google LLC holds a significant market share, accounting for 17% of the total market.
- Close behind, Microsoft Corporation commands an 18% market share, showcasing its substantial presence in the data science platform sector.
- IBM Corporation follows closely with a 15% market share, underlining its long-standing expertise in data analytics and data-driven solutions.
- Further diversifying the market, H2O.ai and Oracle each contribute 8% of the market share, reflecting their prominence in the industry.
- Alteryx, Inc. holds a notable 14% market share, indicating its strong market position. TIBCO Software Inc. and SAP share 6% of the market each, emphasizing their significance in the data science platform arena.
- Collectively, these key players play a pivotal role in driving innovation, shaping the future of data science, and meeting the diverse needs of businesses and organizations.
- Additionally, other key players collectively make up 8% of the market share. Highlighting the dynamic nature of the data science platform industry and the continuous emergence of new entrants and technologies.
(Source: Market.us)
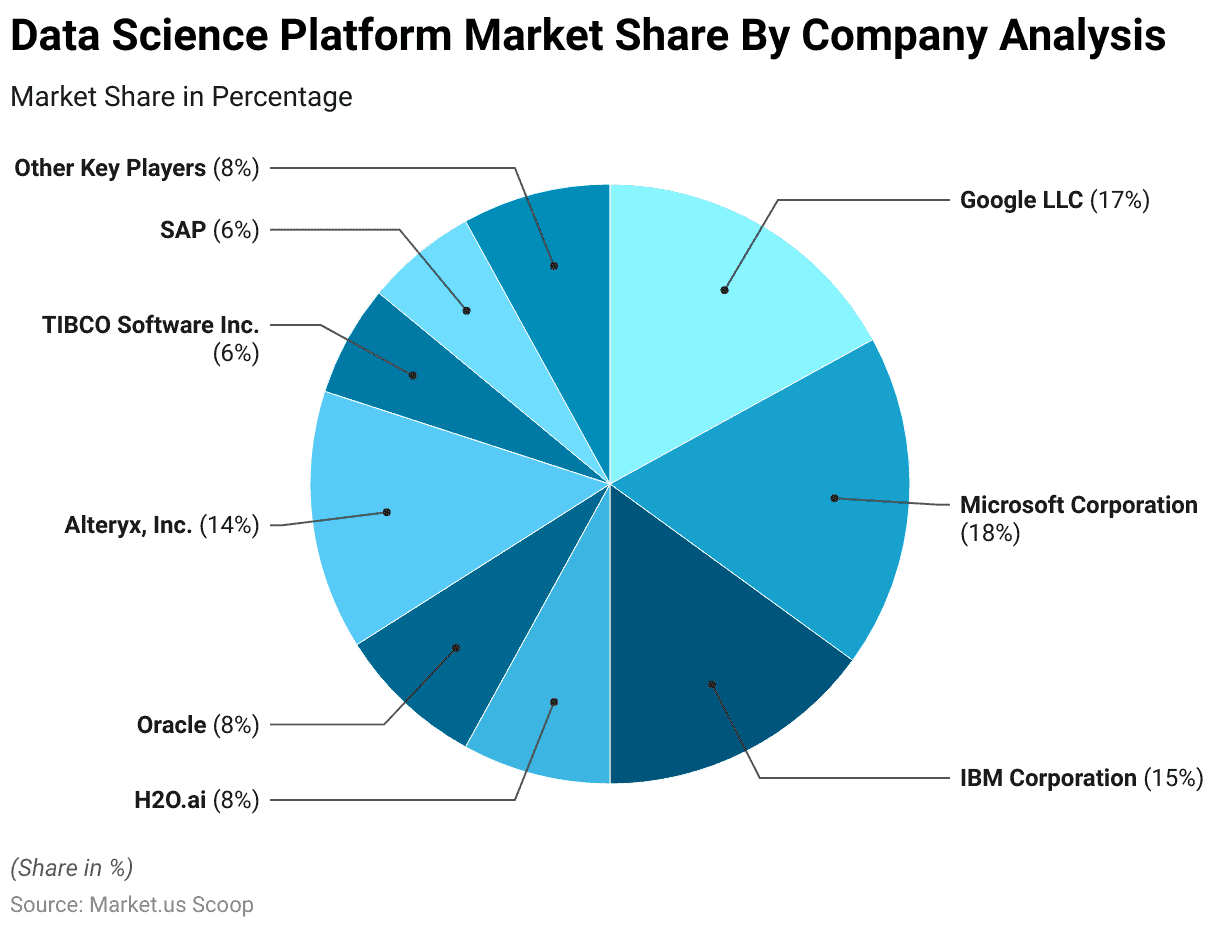
Popular Data Science Platform Statistics
KNIME Analytics Platform
- KNIME excels in managing complete workflows for machine learning and predictive analytics.
- It efficiently gathers extensive data, including from notable sources like Google and Twitter, making it a preferred choice for enterprise applications.
- KNIME offers seamless transitions to cloud environments with integrations for Microsoft Azure and AWS.
- Its versatility and comprehensive approach stand out, and its long-term strategy and plans surpass those of many rivals.
(Source: KNIME)
Saturn Cloud
- Saturn Cloud serves as a data science platform that supports Python, R, and Julia for both teams and individuals.
- It leverages GPU computing to significantly accelerate data science tasks, sometimes by as much as 2000 times.
- Saturn offers a versatile setting where data scientists can readily deploy robust notebooks such as Jupyter, RStudio, VS Code, and others in the cloud.
- They can also swiftly utilize Dask clusters and GPUs, extend their data science capabilities by deploying cloud resources, and engage in collaborative work throughout the entire project journey, among other features.
(Source: Saturn Cloud)
H2O.ai
- H2O provides extensive deep-learning capabilities that enhance your access to artificial intelligence. It stands as a top-tier platform that unifies machine learning processes.
- Additionally, it is open-source and includes a section dedicated to predictive analytics.
- Notably, it has attracted the attention of several large enterprises, such as PayPal and Dun & Bradstreet.
- Its open-source machine learning component has become a widely accepted industry norm.
(Source: H2O.ai)
Cloudera
- Cloudera stands as a highly favored platform tailored for both cloud and enterprise data needs.
- It comes equipped with automated data pipelines and robust support for complete Hadoop security and data encryption.
- Cloudera excels in handling the kind of sensitive data frequently found in large corporate settings, ensuring the safety of Spark queries.
- Additionally, it offers the capability to share models as REST APIs seamlessly, eliminating the need for extensive rewrites.
(Source: Cloudera)
Databricks
- This platform originates from the creators of Apache Spark and combines elements of data science, data engineering, and business analytics.
- It excels in seamlessly integrating with various ecosystem tools. Making it a preferred option for businesses with a range of favored tools in place.
- It offers features like shared revision history and GitHub integration.
- It effectively manages the production aspects of analytics, including pipelines and monitoring, and consistently updates and improves machine learning models.
- It provides the advantages of scalability while maintaining agility, and it also offers the option to rely on its managed service for security concerns.
(Source: Databricks)
Programming Languages in Data Science Platform Statistics
- Programming languages are a fundamental aspect of data science platforms. Influencing the tools and capabilities available to data scientists and analysts.
- Among these languages, Python stands out as the dominant choice, commanding a significant share of 27.91%.
- Python’s popularity in the field can be attributed to its extensive library support for data manipulation, machine learning, and visualization, making it the go-to language for many data professionals.
- Java, with a 16.58% share, is another prominent language, offering versatility and compatibility with various data-related applications.
- JavaScript, at 9.67%, plays a critical role in web-based data visualization and interactive data analysis, contributing to its significance in data science projects.
- C/C++ (6.93%) and C# (6.88%) provide essential options for data-intensive applications where performance and efficiency are paramount.
- PHP (5.19%) finds its place in web development and data processing tasks, diversifying the range of available tools.
- R, TypeScript, Swift, and Objective-C, with shares ranging from 2.26% to 4.23%, represent specialized languages used in specific data science niches.
- R is particularly well-suited for statistical analysis and data visualization. While TypeScript, Swift, and Objective-C are used in applications where their unique strengths come into play.
(Source: PYPL)
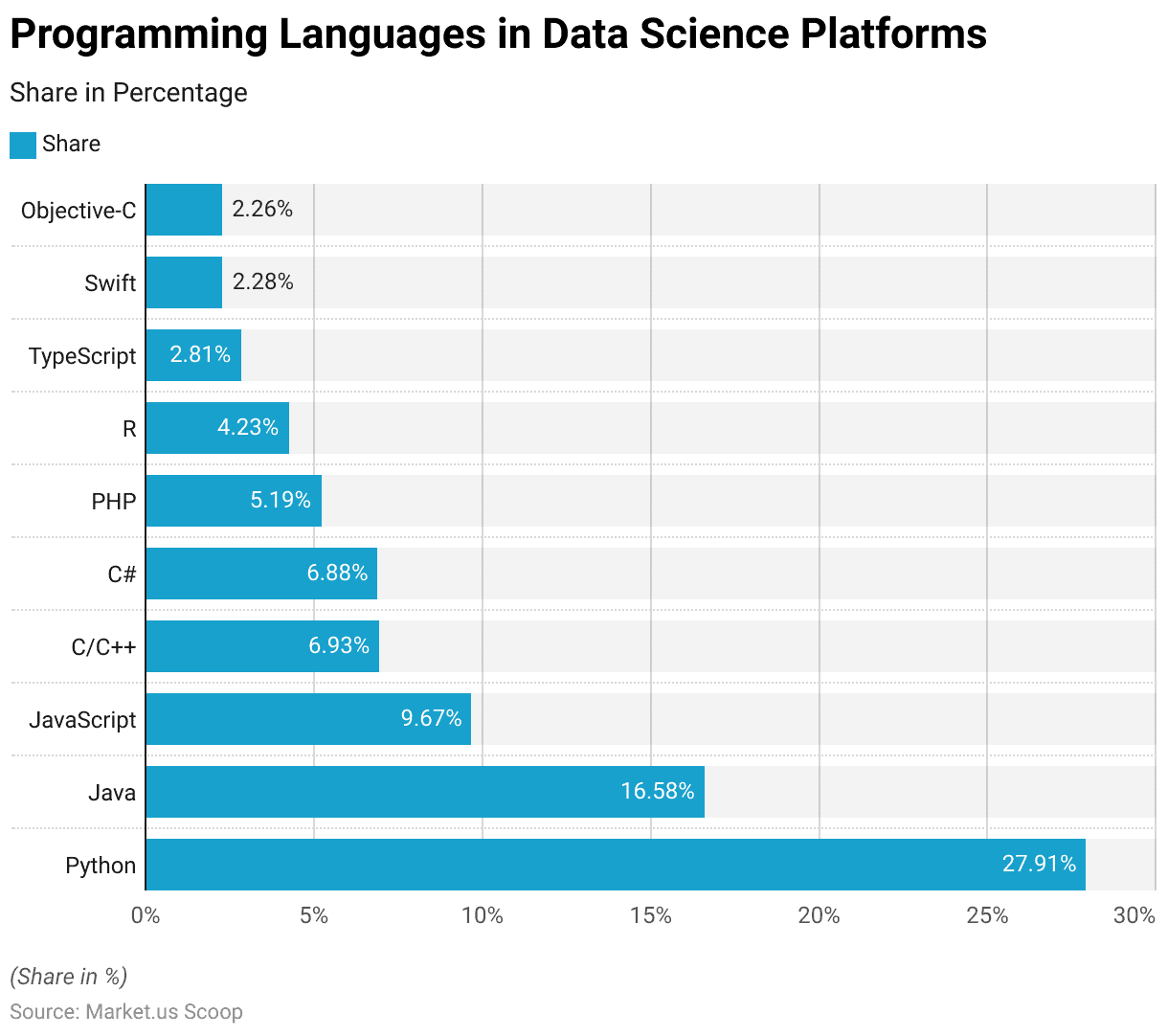
Upcoming Trends for Data Science Platforms Statistics
Edge Computing
- Edge computing refers to the practice of processing data close to where it is collected. This approach enables immediate decision-making based on information gathered from internet-connected sensors located in places such as factories, transportation networks, retail outlets, and remote areas.
- According to Gartner’s predictions, by 2025, approximately 75% of the data generated by enterprises will be generated and managed outside of the conventional data centers and cloud infrastructure.
- The trend towards adopting edge analytics is on the rise, particularly in situations where quick responses to real-time data are essential.
- To support this trend, the concept of “edge” is evolving into a more comprehensive concept known as “fog computing.”
- This evolution reflects the increasing importance of processing data closer to its source to meet the demands of a rapidly changing technological landscape.
(Source: Gartner)
Data-as-a-Service (DaaS)
- Data as a Service (DaaS) is a data management approach that harnesses the value of data as a valuable corporate asset to improve business flexibility.
- It falls within the broader category of “as a service” models, which gained prominence in the 1990s with the advent of the internet and the introduction of Software as a Service (SaaS).
- Similar to other “as a service” models, DaaS empowers organizations to efficiently handle large volumes of daily data. It simplifies the dissemination of critical data across the entire organization, enabling well-informed decision-making.
- DaaS solutions liberate data from the constraints of traditional data centers. However, unlike Software as a Service (SaaS), DaaS does not offer business users application functionalities without local installation.
- Additionally, it lacks the application development environment provided by Platform as a Service (PaaS).
(Source: Great Learning Education Services Private Limited)
Federated Learning
- Federated Learning enables mobile devices to collaborate in constructing a collective prediction model without the necessity of centralizing training data on a distant cloud server.
- On-device model training, which is an advancement beyond local models directly making predictions on mobile devices. Similar to Mobile Vision API and On-Device Smart Reply, is introduced.
- Here’s the process: Your device acquires the existing model. Enhances it by learning from the data on your device, and summarizes the changes into a concise update.
- This update is the sole part that’s securely transmitted to the cloud through encryption. In the cloud, it’s amalgamated with updates from other users to improve the shared model.
- Significantly, your data remains on your device, and no personal information is stored in the cloud.
(Source: Great Learning Education Services Private Limited)
Data Governance and Regulation
- In 2023, data governance will take center stage as governments worldwide introduce new regulations to oversee the use of personal and other data categories.
- Following the examples set by regulations such as the European GDPR, Canadian PIPEDA, and Chinese PIPL. Many other countries are expected to follow suit by enacting laws aimed at safeguarding their citizens’ data.
- According to Gartner analysts, it’s anticipated that by 2023, roughly 65% of the global population will be subject to GDPR-like regulations.
- Consequently, businesses across the globe will face the crucial responsibility of ensuring that their internal procedures for handling and processing data are thoroughly documented and well-understood.
- This entails conducting audits to assess the types of data they possess, how it’s acquired, where it’s stored, and how it’s utilized. While this might seem like additional effort, the ultimate objective is to foster consumer trust in organizations’ data management practices.
- When consumers have confidence in the security of their data, organizations can leverage it to develop products and services that better align with customer needs and affordability.
(Source: Gartner)
Recent Developments
Acquisitions and Mergers:
- Snowflake acquires Streamlit: In 2023, Snowflake, a cloud data platform, acquired Streamlit, a popular data science platform for building data apps, for $800 million. This acquisition enhances Snowflake’s data science and machine learning capabilities. Allowing data teams to collaborate and build interactive data applications more efficiently.
- Alteryx acquires Hyper Anna: In 2023, Alteryx, a leading data analytics platform, completed the acquisition of Hyper Anna, an AI-powered analytics startup, for $180 million. The merger aims to strengthen Alteryx’s data science platform by integrating AI-driven insights and automation features, enhancing the user experience.
New Product Launches:
- Microsoft Azure launches Synapse Analytics integration: In 2023, Microsoft introduced a new integration between Azure Synapse Analytics and its data science platform. This integration provides a unified environment for big data processing, machine learning, and business analytics, making it easier for data teams to collaborate and build models.
- Google Cloud introduces Vertex AI Workbench: In early 2024, Google Cloud launched Vertex AI Workbench, an enhanced data science platform that integrates machine learning development with Google’s cloud infrastructure. The platform simplifies model building and operationalization, allowing data scientists to work more efficiently.
Funding:
- Databricks raises $1.6 billion for platform expansion: In 2023, Databricks, a cloud-based data science and analytics platform, raised $1.6 billion in a Series H funding round, bringing its valuation to $38 billion. The funding will be used to expand Databricks’ machine learning and AI capabilities, with a focus on growing its customer base in new markets.
- DataRobot secures $300 million in funding: In 2024, DataRobot, a leader in AI and machine learning automation, raised $300 million in Series G funding. The investment will fuel innovation in its data science platform, specifically targeting advancements in AI-driven automation and end-to-end machine learning model deployment.
Technological Advancements:
- AI and automation in data science: The integration of AI and automation is transforming data science platforms. By 2025, over 50% of data science platforms will feature AI-driven automation, reducing manual efforts in data preparation, model training, and deployment, significantly improving efficiency.
- Low-code and no-code data science platforms: Low-code and no-code platforms are gaining traction, allowing non-experts to engage in data science tasks. By 2026, 40% of businesses are expected to adopt low-code/no-code data science tools to democratize data insights across teams and departments.
Conclusion
Data Science Platform Statistics – Data science platforms are essential for organizations aiming to harness data’s power for informed decisions and innovation.
They offer comprehensive solutions for data collection, analysis, and visualization, enabling businesses to gain valuable insights.
The market is growing, driven by the increasing importance of data-driven strategies and technological advancements.
While established players like Google, Microsoft, and IBM dominate, new entrants contribute significantly, making this field dynamic.
As data becomes more central to business success, data science platforms remain crucial for adapting and thriving in today’s data-centric landscape.
FAQs
A data science platform is a software solution or ecosystem that provides tools and capabilities for data collection, cleaning, analysis, modeling, and visualization. It enables data scientists and analysts to work efficiently with data to extract valuable insights and make data-driven decisions.
A data science platform typically includes components like data integration and preparation tools, machine learning and statistical modeling libraries, data visualization tools, and collaboration features. It may also have cloud integration and support for various programming languages.
Data science helps businesses analyze large datasets to gain insights, make predictions, and optimize processes. It can lead to improved decision-making, cost savings, and the development of innovative products and services.
Data science platforms are more comprehensive and geared towards advanced analytics, including machine learning and predictive modeling. They offer a broader range of tools and capabilities compared to traditional data analytics software.
Python and R are two of the most commonly used programming languages in data science platforms. They offer extensive libraries and frameworks for data analysis and machine learning.
Discuss your needs with our analyst
Please share your requirements with more details so our analyst can check if they can solve your problem(s)
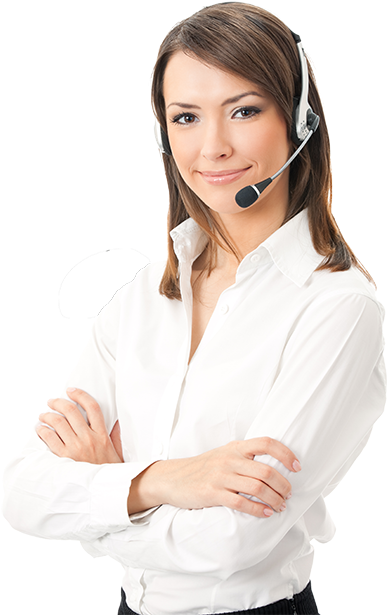