Table of Contents
Introduction
According to Data Science Platform Statistics, A Data Science Platform (DSP) is a software ecosystem that facilitates the entire data analysis process. It integrates data from diverse sources, prepares and cleans the data, enables exploration, builds predictive models, and assesses their performance.
DSPs streamline and enhance efficiency in data-driven decision-making, making them valuable tools for professionals in various industries. Some popular DSPs include IBM Watson Studio, DataRobot, Databricks, Google Cloud AI Platform, and Microsoft Azure Machine Learning, each offering tailored features and integrations.
Editor’s Choice
- The Data Science Platform market has been experiencing robust and consistent growth over the past few years at a CAGR of 25.7%.
- In 2021, the market generated a revenue of approximately USD 64.09 billion.
- North America leads the way with the largest market share, accounting for a significant 38.0% of the total market.
- Google LLC holds a significant market share, accounting for 17% of the total market.
- Saturn Cloud serves as a data science platform that supports Python, R, and Julia for both teams and individuals.
- Among programming languages, Python stands out as the dominant choice, commanding a significant share of 27.91%.
- According to Gartner’s predictions, by 2025, approximately 75% of the data generated by enterprises will be generated and managed outside of the conventional data centers and cloud infrastructure.
Global Data Science Platform Market Overview
Global Data Science Platform Market Size
- The Data Science Platform market has been experiencing robust and consistent growth over the past few years at a CAGR of 25.7%, reflecting the growing significance of data analytics and data-driven decision-making across industries.
- In 2021, the market generated a revenue of approximately USD 64.09 billion, indicating a strong demand for data science tools and platforms to extract insights from the ever-expanding volume of data.
- This growth momentum continued into 2022, with the market reaching a revenue of USD 89.79 billion, demonstrating a substantial increase in investment in data science capabilities.
- In subsequent years, the market is forecasted to continue its expansion, with revenues reaching USD 141.19 billion in 2024 and USD 166.89 billion in 2025.
- By 2032, it is projected to achieve a substantial revenue of USD 346.79 billion.
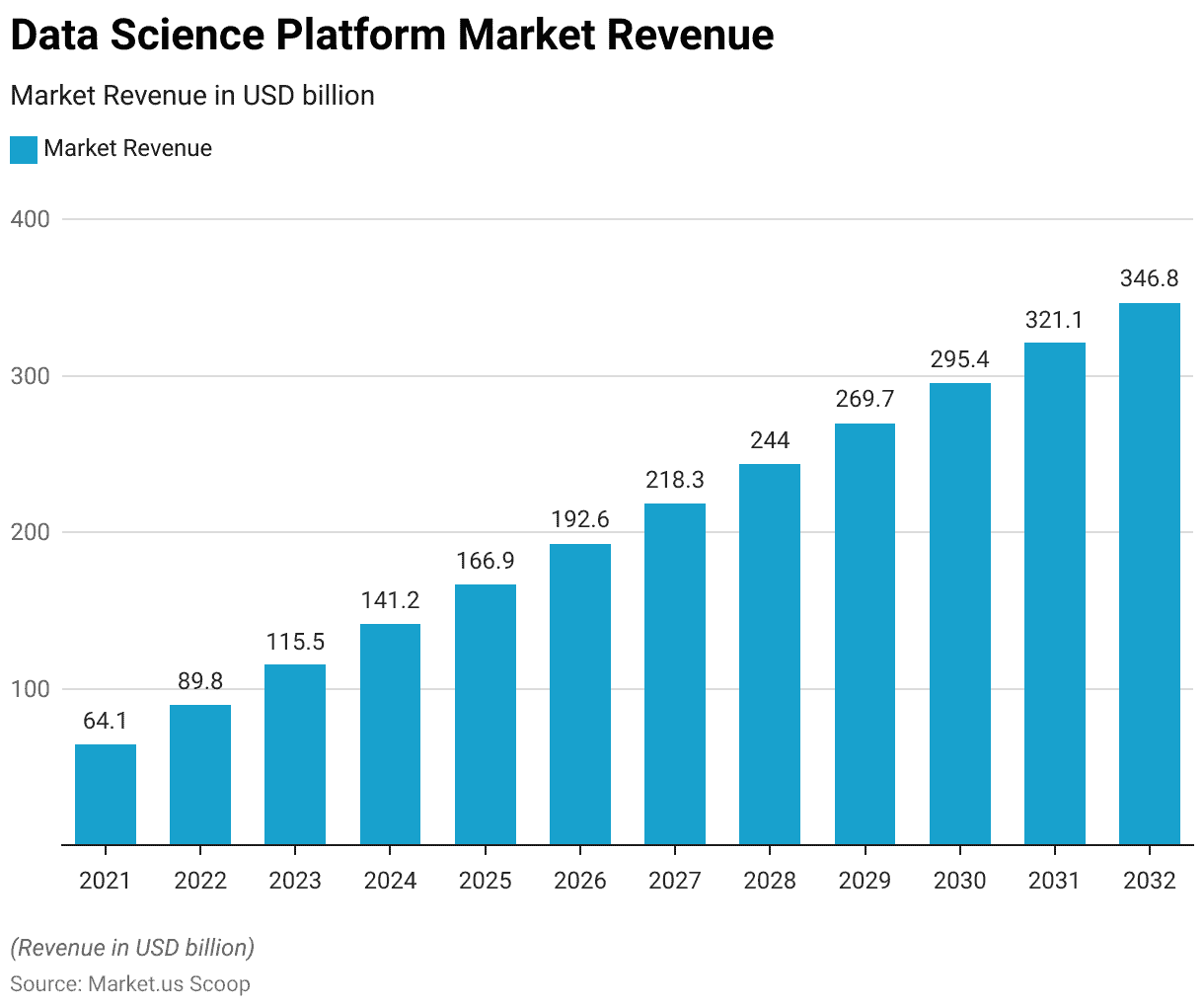
Regional Analysis of the Data Science Platform Market
- North America leads the way with the largest market share, accounting for a significant 38.0% of the total market.
- Europe follows closely behind, contributing 24.0% of the market share, underlining the continent’s growing emphasis on data-driven decision-making and innovation.
- In the Asia-Pacific (APAC) region, there is a substantial market presence, capturing 22.0% of the market share.
- South America accounts for 9.0% of the market share, demonstrating a notable but smaller presence, while the Middle East and Africa (MEA) contribute 7.0% to the overall market share.
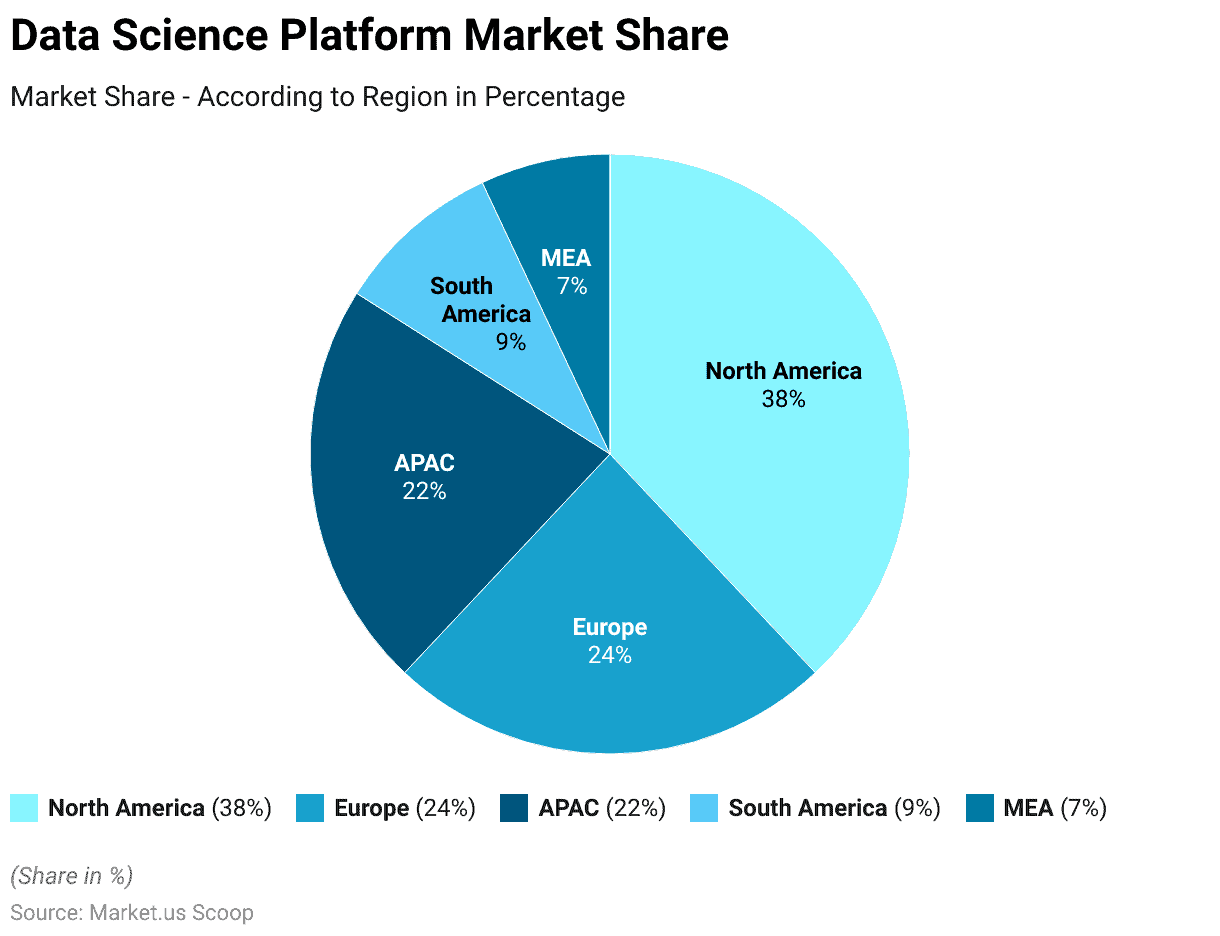
Key Players in the Data Science Platform Market
- Notably, Google LLC holds a significant market share, accounting for 17% of the total market.
- Close behind, Microsoft Corporation commands an 18% market share, showcasing its substantial presence in the data science platform sector.
- IBM Corporation follows closely with a 15% market share, underlining its long-standing expertise in data analytics and data-driven solutions.
- Further diversifying the market, H2O.ai and Oracle each contribute 8% of the market share, reflecting their prominence in the industry.
- Alteryx, Inc. holds a notable 14% market share, indicating its strong market position. TIBCO Software Inc. and SAP share 6% of the market each, emphasizing their significance in the data science platform arena.
- Collectively, these key players play a pivotal role in driving innovation, shaping the future of data science, and meeting the diverse needs of businesses and organizations.
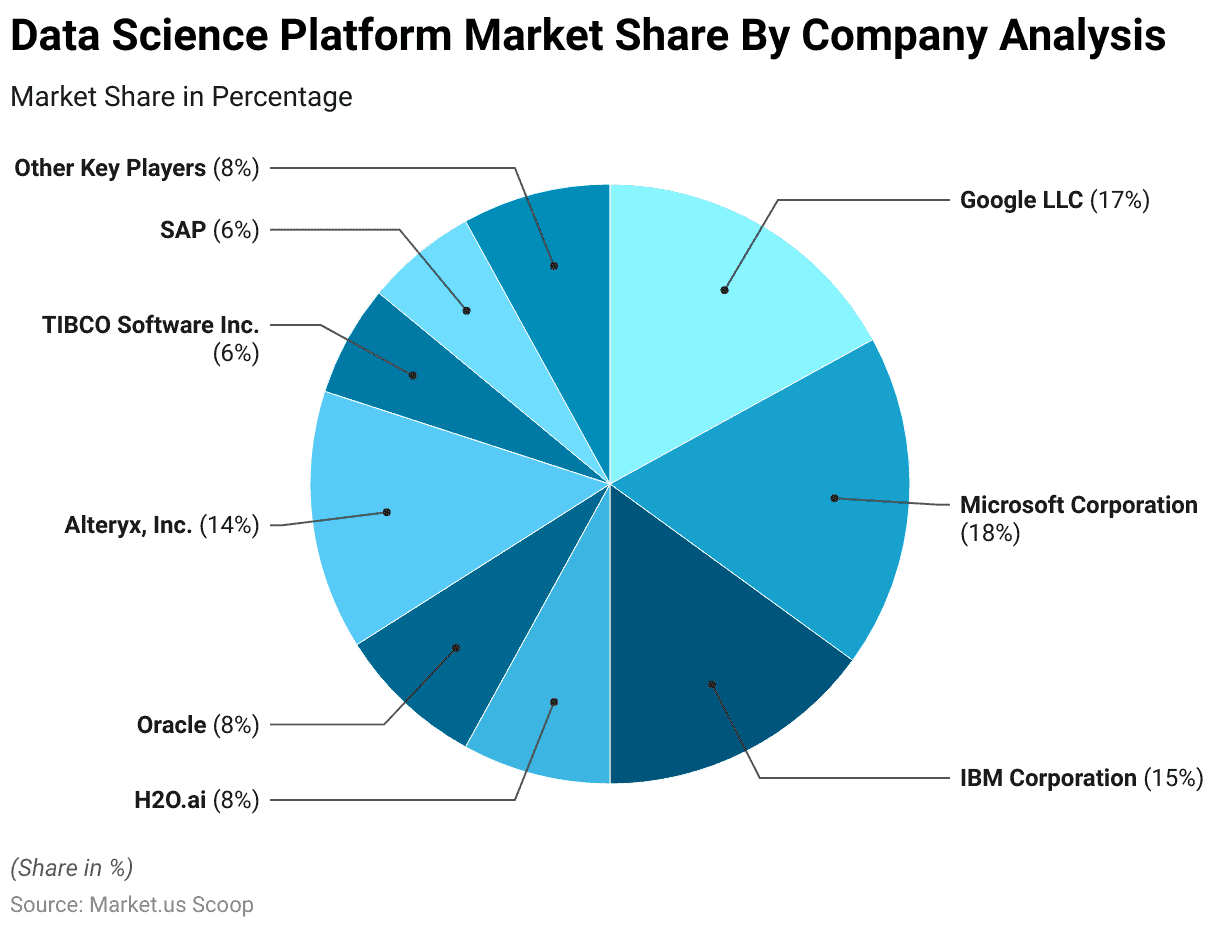
Popular Data Science Platforms
KNIME Analytics Platform
- KNIME excels in managing complete workflows for machine learning and predictive analytics.
- It efficiently gathers extensive data, including from notable sources like Google and Twitter, making it a preferred choice for enterprise applications.
- KNIME offers seamless transitions to cloud environments with integrations for Microsoft Azure and AWS.
- Its versatility and comprehensive approach stand out, and its long-term strategy and plans surpass those of many rivals.
Saturn Cloud
- Saturn Cloud serves as a data science platform that supports Python, R, and Julia for both teams and individuals.
- It leverages GPU computing to significantly accelerate data science tasks, sometimes by as much as 2000 times.
- Saturn offers a versatile setting where data scientists can readily deploy robust notebooks such as Jupyter, RStudio, VS Code, and others in the cloud.
- They can also swiftly utilize Dask clusters and GPUs, extend their data science capabilities by deploying cloud resources, and engage in collaborative work throughout the entire project journey, among other features.
H2O.ai
- H2O provides extensive deep-learning capabilities that enhance your access to artificial intelligence. It stands as a top-tier platform that unifies machine learning processes.
- Additionally, it is open-source and includes a section dedicated to predictive analytics.
- Notably, it has attracted the attention of several large enterprises, such as PayPal and Dun & Bradstreet.
- Its open-source machine learning component has become a widely accepted industry norm.
Cloudera
- Cloudera stands as a highly favored platform tailored for both cloud and enterprise data needs.
- It comes equipped with automated data pipelines and robust support for complete Hadoop security and data encryption.
- Cloudera excels in handling the kind of sensitive data frequently found in large corporate settings, ensuring the safety of Spark queries.
- Additionally, it offers the capability to share models as REST APIs seamlessly, eliminating the need for extensive rewrites.
Databricks
- This platform originates from the creators of Apache Spark and combines elements of data science, data engineering, and business analytics.
- It excels in seamlessly integrating with various ecosystem tools, making it a preferred option for businesses with a range of favored tools in place.
- It offers features like shared revision history and GitHub integration.
Programming Languages in Data Science Platforms
- Programming languages are a fundamental aspect of data science platforms, influencing the tools and capabilities available to data scientists and analysts.
- Among these languages, Python stands out as the dominant choice, commanding a significant share of 27.91%.
- Python’s popularity in the field can be attributed to its extensive library support for data manipulation, machine learning, and visualization, making it the go-to language for many data professionals.
- Java, with a 16.58% share, is another prominent language, offering versatility and compatibility with various data-related applications.
- JavaScript, at 9.67%, plays a critical role in web-based data visualization and interactive data analysis, contributing to its significance in data science projects.
- C/C++ (6.93%) and C# (6.88%) provide essential options for data-intensive applications where performance and efficiency are paramount.
- PHP (5.19%) finds its place in web development and data processing tasks, diversifying the range of available tools.
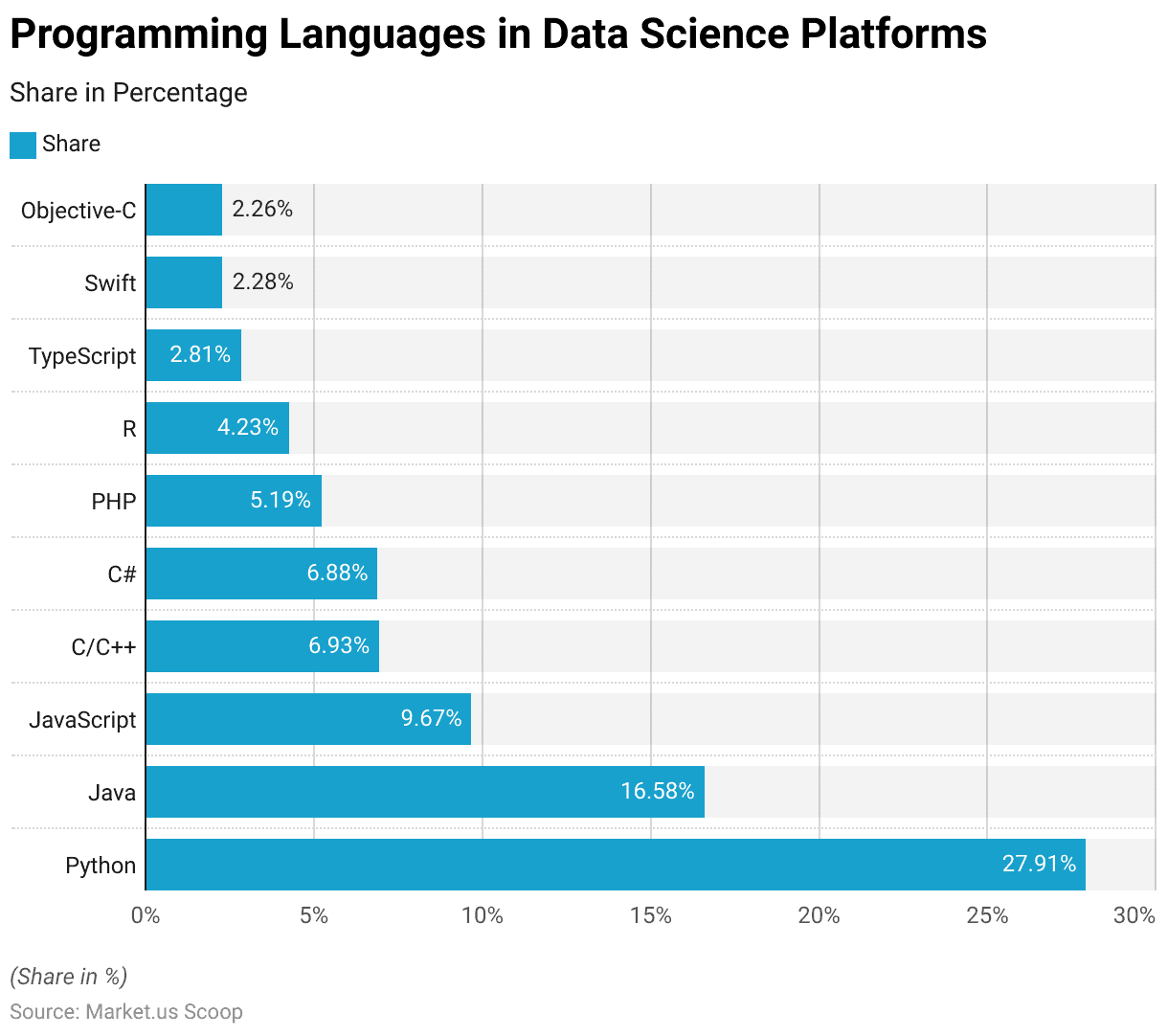
Upcoming Trends for Data Science Platforms
Edge Computing
- Edge computing refers to the practice of processing data close to where it is collected. This approach enables immediate decision-making based on information gathered from internet-connected sensors located in places such as factories, transportation networks, retail outlets, and remote areas.
- According to Gartner’s predictions, by 2025, approximately 75% of the data generated by enterprises will be generated and managed outside of the conventional data centers and cloud infrastructure.
- The trend towards adopting edge analytics is on the rise, particularly in situations where quick responses to real-time data are essential.
Data-as-a-Service (DaaS)
- Data as a Service (DaaS) is a data management approach that harnesses the value of data as a valuable corporate asset to improve business flexibility.
- It falls within the broader category of “as a service” models, which gained prominence in the 1990s with the advent of the internet and the introduction of Software as a Service (SaaS).
- Similar to other “as a service” models, DaaS empowers organizations to efficiently handle large volumes of daily data. It simplifies the dissemination of critical data across the entire organization, enabling well-informed decision-making.
Federated Learning
- Federated Learning enables mobile devices to collaborate in constructing a collective prediction model without the necessity of centralizing training data on a distant cloud server.
- On-device model training, which is an advancement beyond local models directly making predictions on mobile devices, similar to Mobile Vision API and On-Device Smart Reply, is introduced.
- Here’s the process: Your device acquires the existing model, enhances it by learning from the data on your device, and summarizes the changes into a concise update.
Data Governance and Regulation
- In 2023, data governance will take center stage as governments worldwide introduce new regulations to oversee the use of personal and other data categories.
- Following the examples set by regulations such as the European GDPR, Canadian PIPEDA, and Chinese PIPL, many other countries are expected to follow suit by enacting laws aimed at safeguarding their citizens’ data.
- According to Gartner analysts, it’s anticipated that by 2023, roughly 65% of the global population will be subject to GDPR-like regulations.
- Consequently, businesses across the globe will face the crucial responsibility of ensuring that their internal procedures for handling and processing data are thoroughly documented and well-understood.
Discuss your needs with our analyst
Please share your requirements with more details so our analyst can check if they can solve your problem(s)
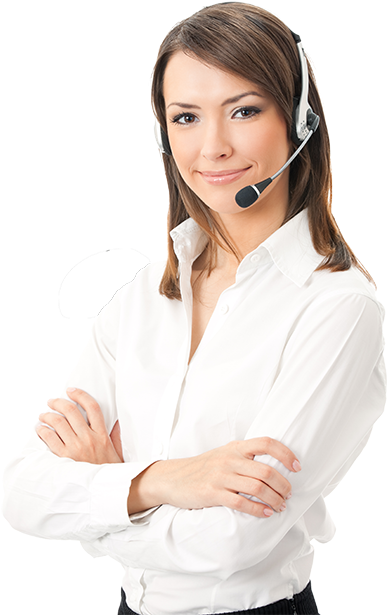