Table of Contents
Introduction
The Global Explainable AI Market is poised for substantial growth, projected to escalate from USD 6.4 billion in 2023 to approximately USD 34.6 billion by 2033, advancing at a CAGR of 18.4% during the forecast period from 2024 to 2033. This market expansion is underpinned by the increasing demand for transparency and interpretability in AI systems across various sectors. The emergence of explainable AI (XAI) aims to bridge the gap between AI’s complex decision-making processes and human understanding, ensuring that AI technologies are accessible, understandable, and trustable for their users.
Recent developments highlight the market’s dynamic nature, with key players like Epic, SAP, IBM, Google, Esquire Bank, NVIDIA, and SAS Institute forging strategic partnerships and launching innovative solutions to harness the power of explainable AI in fields ranging from healthcare to banking and beyond. These initiatives not only aim to enhance AI’s decision-making transparency but also cater to the specific needs of various industries, including healthcare, BFSI, IT and telecommunication, and retail, among others.
Key Takeaways
- The Explainable AI market is expected to grow from USD 6.4 billion in 2023 to approximately USD 34.6 billion by 2033, with a robust compound annual growth rate (CAGR) of 18.4% during the forecast period.
- XAI aims to make artificial intelligence systems understandable by providing transparent explanations for their decision-making processes.
- Businesses and organizations are increasingly investing in XAI to enhance transparency, comply with regulations, and gain competitive advantages.
- Significant investment activity in the XAI sector includes notable funding rounds for key players such as H2O.ai, Anthropic, and Fiddler Labs, totaling USD 2.2 billion in 2023.
- By component, hardware dominates the market with over 60.3% share in 2023, driven by specialized hardware like GPUs and TPUs crucial for transparent AI models.
- Network security leads by application with over 53% share in 2023, addressing the escalating threats of cyber-attacks with transparent AI-driven security solutions.
- Government and defense hold a dominant position among end-users, with over 36% share in 2023, utilizing XAI for operational efficiency and national security measures.
- North America leads the market with over 31.6% share in 2023, driven by robust technological infrastructure and regulatory focus on AI transparency.
- The shortage of skilled XAI professionals poses a challenge for widespread adoption, hindering the development and deployment of XAI solutions.
- One significant opportunity XAI presents is enhanced decision support, empowering stakeholders to make more informed decisions.
- Key players in the XAI market include ID Quantique, QuintessenceLabs, MagiQ Technologies (acquired by Raytheon Technologies Corporation), Toshiba Corporation, and others.
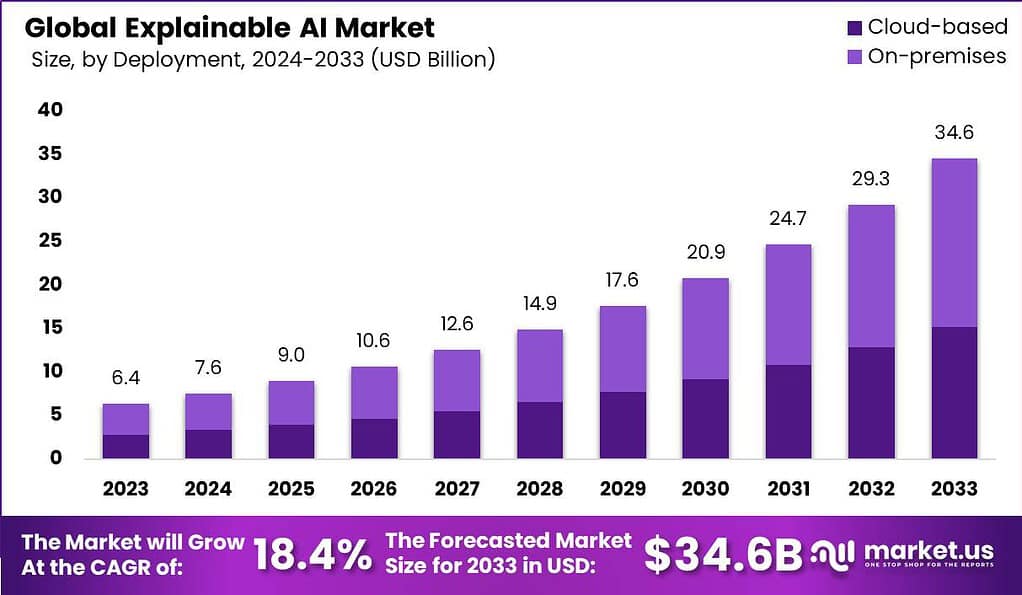
Use Cases Of Explainable AI
- Regulatory Compliance: In the EU, regulations like GDPR mandate a “right to explanation” for individuals affected by AI decisions. XAI helps organizations meet these legal requirements by making AI’s decision-making processes transparent.
- Healthcare: XAI plays a crucial role in diagnosing diseases, predicting patient outcomes, and recommending treatments. By explaining the rationale behind its predictions, XAI enables healthcare providers to make informed decisions, thus potentially saving lives.
- Manufacturing: XAI helps improve product quality and optimize production processes by analyzing production data to identify factors affecting product quality and explaining the reasons behind these factors.
- Autonomous Vehicles: For autonomous vehicles, safety and trust are paramount. XAI can explain driving decisions made by the vehicle, such as when to brake or change lanes, which is crucial for understanding the vehicle’s actions, especially in the event of accidents.
- Fraud Detection: In the financial sector, XAI aids in identifying fraudulent transactions by explaining why a transaction is considered fraudulent. This not only helps in detecting fraud more accurately but also assists in regulatory compliance and dispute resolution.
- Insurance: XAI finds applications in customer retention, claims management, and insurance pricing by providing explanations for its predictions, thereby improving customer satisfaction and trust.
- Banking: From fraud detection to customer engagement and tailored pricing, XAI enhances banking operations by ensuring that AI models’ predictions are understandable, thus increasing confidence in these automated systems.
Why Do Businesses Need Explainable AI?
- Maximizing Benefits: Companies attributing at least 20% of EBIT to AI usage tend to follow best practices for explainability.
- Revenue Growth: Organizations fostering digital trust through practices like AI explainability witness annual revenue and EBIT growth rates exceeding 10%.
- Understanding Decisions: Users and stakeholders must comprehend how AI models arrive at conclusions to trust the results.
- Building Trust: Explainability fosters trust among customers, regulators, and employees by ensuring accuracy and fairness in decision-making.
- Enhancing Productivity: Techniques enabling explainability help identify errors swiftly, enhancing efficiency in managing AI systems.
- Encouraging Adoption: When users understand the rationale behind AI recommendations, they are more likely to adopt and trust them.
- Revealing Hidden Interventions: Understanding AI models can uncover valuable business interventions that might otherwise remain unnoticed.
- Validating Business Value: Explainability allows businesses to confirm that AI applications align with intended objectives and deliver expected value.
- Mitigating Risks: Explainability assists in identifying and mitigating ethical and regulatory risks associated with AI usage.
- Compliance with Regulations: As regulations concerning AI explainability evolve, businesses must ensure compliance to avoid scrutiny and penalties.
- Establishing Governance: Organizations need to establish governance frameworks to ensure transparency and accountability in AI development and usage.
- Cross-functional Collaboration: A diverse team comprising business leaders, technical experts, and legal professionals is crucial for effective AI governance.
- Setting Standards: AI governance committees set standards for explainability, including risk taxonomy and guidelines for use-case-specific review processes.
- Assessing Risks: Model development teams must assess the explainability needs of each AI use case to manage associated risks effectively.
- Addressing Trade-offs: Sometimes, a trade-off exists between explainability and accuracy, requiring careful consideration and possibly escalation to leadership.
- Investing in Talent: Companies must hire and retain talent with expertise in AI governance, legal compliance, and technology ethics.
- Utilizing Technology: Investing in advanced explainability tools helps meet the diverse needs of AI development teams and ensures accuracy in explanations.
- Continuous Research: AI governance committees must engage in ongoing research to stay updated on legal and regulatory requirements and industry norms.
- Training Programs: Establishing training programs ensures employees across the organization understand and apply the latest developments in AI explainability.
- User Confidence and Bottom Line Impact: Businesses that prioritize explainable AI gain trust from users, regulators, and consumers, ultimately impacting their bottom lines positively.
Discuss your needs with our analyst
Please share your requirements with more details so our analyst can check if they can solve your problem(s)
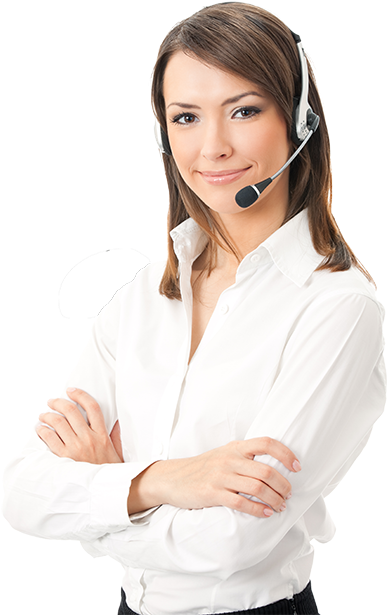