Table of Contents
Introduction
According to Market.us, The Global Federated Learning Market size is expected to be worth around USD 311.4 Million by 2033, from USD 133.1 Million in 2023, growing at a CAGR of 10.2% during the forecast period from 2024 to 2033.
Federated learning is an innovative approach to machine learning that enables collaboration and model training across multiple devices while keeping data decentralized and secure. Unlike traditional methods where data is transferred to a central server, federated learning allows training to occur locally on individual devices, preserving data privacy and ownership. This is particularly useful in situations where data is sensitive or cannot be easily shared due to privacy regulations or security concerns.
The market for federated learning is experiencing significant growth as organizations recognize its potential in leveraging distributed data for machine learning applications. With the increasing number of connected devices and the vast amount of data generated at the edge, federated learning offers a solution to harness this distributed data while ensuring privacy compliance. Industries such as healthcare, finance, telecommunications, and manufacturing can benefit from federated learning by extracting insights and building models while maintaining data security.
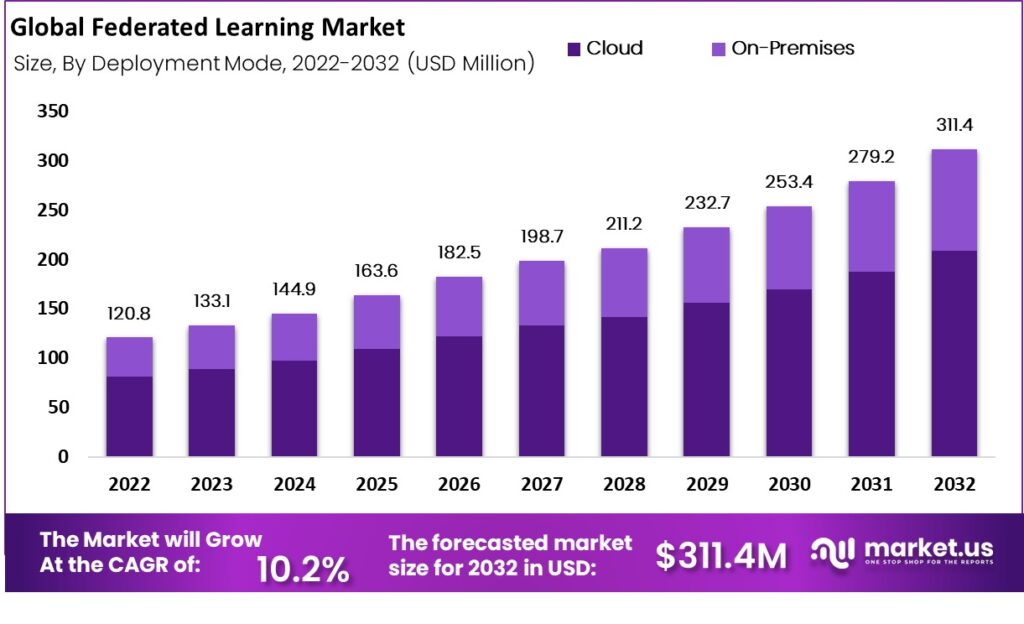
Facts and Latest Statistics
- The Federated Learning Market was valued at USD 133.1 million in 2023 and is projected to reach USD 311.4 million by 2032, exhibiting a compound annual growth rate (CAGR) of 10.2%.
- The finance and banking sector is set to embrace federated learning significantly, with an expected growth rate of approximately 49% from 2022 to 2024. This growth is fueled by its potential in enhancing fraud detection, credit risk analysis, and anti-money laundering efforts.
- Around 30% of organizations are anticipated to adopt federated learning strategies to tackle data privacy and security concerns, while still benefiting from distributed data for AI model training.
- In 2023, the healthcare and life sciences sector is projected to dominate the federated learning market, holding a 36% share. This dominance is primarily due to its applications in medical imaging analysis and drug discovery, showcasing the sector’s rapid adoption of federated learning solutions.
- Approximately 40% of organizations are expected to use federated learning to foster collaborative AI model development and ensure secure data sharing across various entities. This approach highlights federated learning’s role in promoting collaboration while safeguarding data integrity.
- About 25% of organizations are likely to implement federated learning to enhance data sharing and collaboration across different locations and organizations, all while upholding data privacy and meeting regulatory compliance requirements.
- By the end of the period, 20% of organizations are projected to have incorporated federated learning into their AI and analytics strategies. This marks a significant increase from less than 5% in 2022, indicating the growing recognition of federated learning’s benefits in leveraging AI technologies while prioritizing data privacy.
- Cloud deployment is expected to dominate the market due to its cost-efficiency, scalability, and adaptability, contributing to substantial revenue growth.
- Industrial IoT holds the largest share in applications, driven by increasing adoption of big data analytics and technological advancements, particularly in emerging economies.
- Healthcare & Life Science dominates the market, with Manufacturing experiencing rapid growth due to a focus on the Industrial IoT and increased competition.
- Growing adoption in various applications, especially in Healthcare and Life Sciences, is propelling market growth.
- Scarcity of skilled IT professionals hampers market growth potential, posing challenges for companies trying to integrate federated learning into their workflows.
- Federated learning enables collaborative learning among users, presenting opportunities in finance and other sectors.
- Europe is expected to hold the largest market share, particularly in healthcare applications.
- Acuratio, apheresis AI GmbH, Cloudera, Google LLC, Enveil, Edge Delta, FedML, IBM, Sherpa.AI, Nvidia, Intel, Lifebit, and Secure AI Labs are key players in the market, implementing various growth strategies to enhance market presence.
Common Use Cases and Benefits
- Healthcare:
- Collaborative Learning Across Hospitals: With federated learning, hospitals can work together to improve AI models that predict patient outcomes or diagnose conditions, all without sharing the actual patient data. This means a patient’s privacy is protected while still benefiting from insights derived from a broad pool of medical data.
- Personalized Medical Predictions: Doctors can use AI to get tailored health predictions for their patients. Federated learning ensures these predictions are based on vast amounts of data without ever compromising the confidentiality of individual health records.
- Financial Services:
- Enhanced Fraud Detection: Banks can share insights from transaction data to bolster fraud detection without actually sharing the data itself. This collaborative approach means your bank is better equipped to spot and stop fraud, keeping your money safer.
- Customer Privacy: Even as banks get smarter about detecting fraud, they can do so without risking the privacy of customer data. Each branch contributes to the learning model without sending customer data out of the branch.
- IoT and Edge Devices:
- Smart Learning on Devices: Devices like smart thermostats, fitness wearables, and even smart fridges can learn from how you use them to offer a better, more personalized service. Because the learning happens right on the device, your data stays with you.
- Adapting to Contexts: These devices aren’t just learning in isolation; they’re adapting based on data from similar devices, making them smarter in ways that are specifically useful to you.
- Natural Language Processing (NLP):
- Decentralized Data Training: Federated learning allows NLP models to learn from text data that’s spread out across different sources. This could mean better chatbots or smarter AI assistants that understand you better without compromising the privacy of the conversations they learn from.
- Localized Privacy-sensitive Information: The sensitive information contained in text data, like personal messages or reviews, remains secure and decentralized, ensuring that privacy is maintained while still benefiting from collective insights.
Importance of Federated Learning
Federated learning is a big step forward in how machines learn from data, especially when keeping that data safe and private is crucial. Here’s why it’s so important:
- Data Privacy: This is a huge deal. With federated learning, companies can use AI to make smart decisions without risking the privacy of their customers’ or their own sensitive information. Imagine a doctor using a health app to predict patient health risks without ever having to send personal health records out of the hospital’s system. Federated learning makes this possible by training AI models right where the data is, without needing to move it around.
- Learning on the Go: Traditional ways of teaching computers require all the learning to happen in one big central place. This doesn’t work well for gadgets that are out and about, like your smartphone or a self-driving car, which need to keep getting smarter even while they’re on the edge of the network. Federated learning changes the game by allowing these devices to learn from new information as they encounter it, making them more intelligent and responsive in real-time.
- Keeping Data in Its Place: Normally, when AI needs to learn from a lot of data, this data has to be collected and sent to one central spot. This is risky because it could lead to leaks or misuse of very private information. Federated learning turns this approach on its head by saying “Let’s keep the data right where it is and do the learning there.” This way, each device or server works with its own data, learns from it, and then shares its new knowledge in a secure way without ever exposing the actual data.
Recent Developments
- January 2023: Google and Nvidia unveil a partnership to create “Federated Learning on Kubernetes (FL on K8s),” an open-source platform aimed at advancing federated learning technologies.
- March 2023: IBM introduces “IBM Maximo for Federated Learning,” a new collection of federated learning tools designed to optimize asset management within industrial environments.
- June 2023: Intel launches an updated version of its Open Federated Learning (OpenFHE) toolkit, featuring enhanced security and performance capabilities.
- August 2023: Lifebit, a startup dedicated to privacy-preserving AI, secures $20 million in Series A financing. This funding is intended to fast-track the development of its federated learning platform.
- October 2023: Secure AI Labs announces a collaboration with a leading healthcare provider. This partnership focuses on employing its secure federated learning solutions for collaborative medical research purposes.
Conclusion
Federated Learning represents a transformative shift in the landscape of data analysis and machine learning, primarily driven by the increasing need for privacy-preserving techniques in data utilization. The growth of Federated Learning can be attributed to several critical factors. Foremost among these is the escalating concern for data privacy, necessitating the development of methods that allow for the improvement of machine learning models without direct access to the underlying data. Additionally, the proliferation of edge computing devices, such as smartphones and IoT devices, which generate vast amounts of data, underscores the need for decentralized data processing methodologies that Federated Learning offers.
The opportunities presented by Federated Learning are vast and multifaceted. It enables organizations to leverage the collective intelligence from distributed data sources while adhering to privacy regulations, thereby expanding the scope for innovation in data-driven industries. This approach not only enhances model accuracy by learning from a diverse dataset but also opens new avenues for collaboration across sectors without compromising sensitive information. Moreover, Federated Learning is poised to significantly benefit sectors where data sensitivity is paramount, such as healthcare, finance, and public services, by facilitating the development of personalized, yet privacy-compliant, solutions.
Discuss your needs with our analyst
Please share your requirements with more details so our analyst can check if they can solve your problem(s)
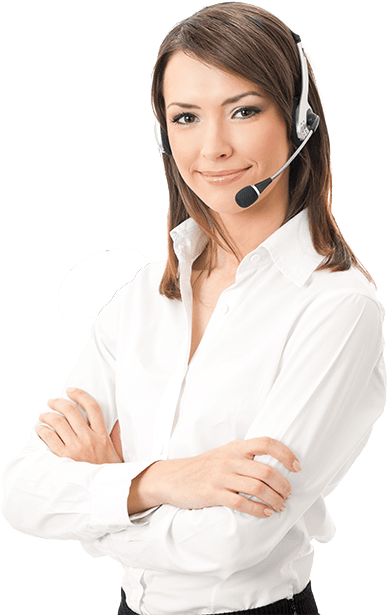