Table of Contents
- Introduction
- Editor’s Choice
- History of Gesture Recognition Technology
- Body Parts Used for Gesturing
- Gesture Recognition Market Statistics
- Gesture Recognition Systems and Datasets Statistics
- Sensors Used in Gesture Recognition Statistics
- Studies Based on Data Environment Types in Hand Gesture Recognition Systems Statistics
- Studies Focusing on Hand Gesture Representations
- Innovations and Developments in Gesture Recognition Technology Statistics
- Regulations for Gesture Recognition Technology Statistics
- Recent Developments
- Conclusion
- FAQs
Introduction
Gesture Recognition Statistics: Gesture recognition is a subfield of computer vision and human-computer interaction that interprets human gestures using algorithms, with applications in gaming, virtual reality, robotics, and assistive devices.
Gestures are categorized into static and dynamic types, with recognition methods. Including vision-based techniques (2D and 3D) and sensor-based approaches utilizing wearable devices.
Challenges such as gesture variability, environmental factors, and the need for real-time processing must be addressed for effective recognition.
Further, this technology has diverse applications, from enhancing gaming experiences to facilitating smart home controls and improving human-robot interaction.
Future trends point toward improved AI algorithms, integration with other technologies, and increased use of wearable gesture recognition systems.
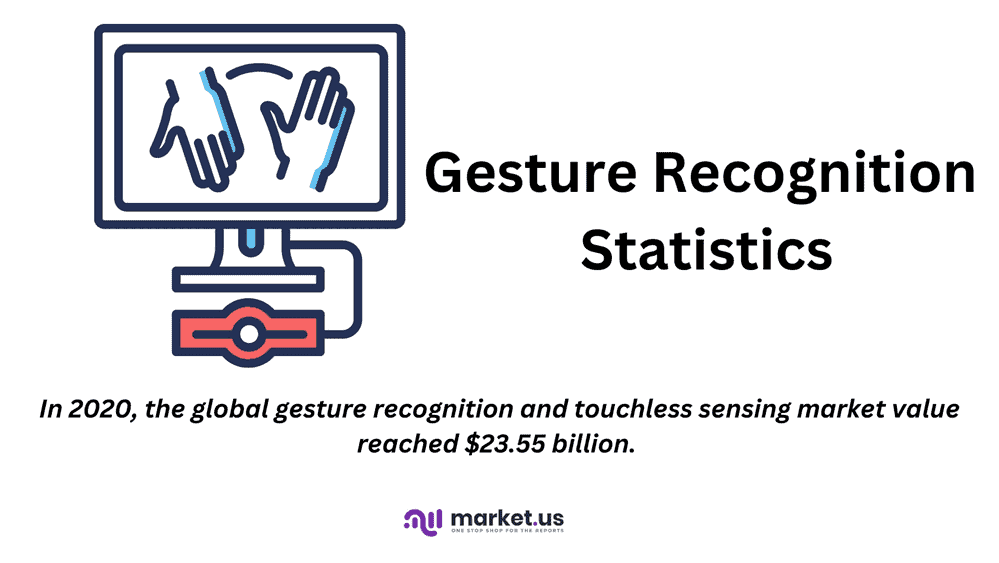
Editor’s Choice
- The 2000s brought notable breakthroughs with the introduction of devices like the Sony PlayStation EyeToy and, later. Microsoft’s Kinect popularized depth-sensing cameras for gesture-based control in gaming and beyond.
- In the realm of gesture recognition, the distribution of body parts used for gesturing varies significantly. The hand is the most commonly used body part, representing 21% of gestures.
- In 2020, the global gesture recognition and touchless sensing market value reached $23.55 billion.
- By 2031, the global gesture recognition market is projected to expand to $89.3 billion ($48.22 billion from touch-based and $41.08 billion from touchless).
- In the global gesture recognition market, the distribution of market share by technology reveals distinct segments. Sensors hold the largest portion, accounting for 42% of the market.
- From 2014 to 2020, studies focusing on hand gesture recognition systems have primarily utilized three types of data environments. Single-camera systems, are the most common. Accounted for 53% of the studies, reflecting their widespread adoption due to simplicity and cost-effectiveness.
- Moreover, a novel approach has been implemented using the MediaPipe framework along with Inception-v3 and LSTM networks to process and analyze temporal data from dynamic gestures, achieving a significant increase in accuracy up to 89.7%.
- In the United States, recent legislative proposals, such as the one introduced by Senators Kennedy and Merkley, target specific uses of facial recognition by federal agencies. Suggesting a cautious approach towards privacy issues, particularly at airports.
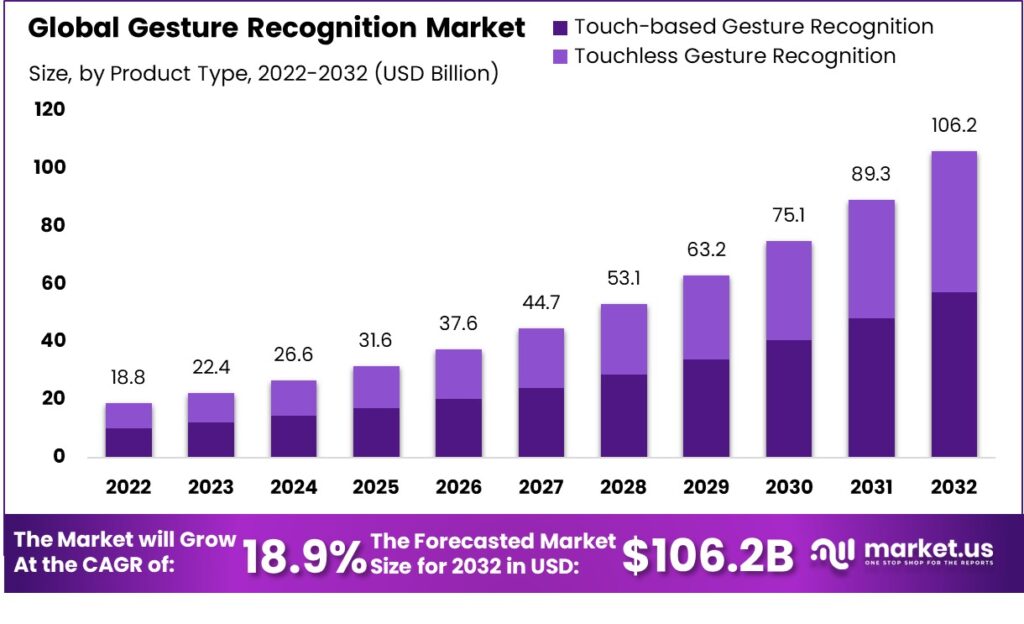
History of Gesture Recognition Technology
- Gesture recognition technology has evolved significantly over the past few decades, beginning with early research in the 1980s and 1990s. Primarily driven by advancements in human-computer interaction.
- Initial work involved the use of data gloves, which measured hand positions and finger movements to recognize gestures.
- In the 1990s, vision-based systems emerged, enabling gesture recognition through image processing and video capture techniques.
- This era saw the development of algorithms capable of interpreting body movements using 2D and 3D models. Marking a significant leap in real-time gesture recognition.
- The 2000s brought notable breakthroughs with the introduction of devices like the Sony PlayStation EyeToy and, later, Microsoft’s Kinect. Which popularized depth-sensing cameras for gesture-based control in gaming and beyond.
- Today, advanced systems integrate machine learning, artificial intelligence, and deep learning algorithms, enabling more precise and natural gesture recognition.
- These systems are widely used in fields such as virtual reality, robotics, healthcare, and automotive interfaces. Continuously evolving with innovations in sensor technology and AI-driven processing methods.
- The future of gesture recognition holds promise for more intuitive, hands-free interaction across multiple sectors.
(Source: Research Gate)
Body Parts Used for Gesturing
- In the realm of gesture recognition, the distribution of body parts used for gesturing varies significantly.
- The hand is the most commonly used body part, representing 21% of gestures.
- This is followed by objects, which are used in 14% of gestures. Indicating a substantial integration of object manipulation within gesture-controlled interfaces.
- Fingers alone account for 10% of gestures, while the entire body is used in 7% of cases.
- Other miscellaneous gestures contribute to 9% of the total. Combinations of body parts are also utilized: gestures involving both the hand and head constitute 7%. While those combining the head and fingers make up 6%.
- Less common are gestures that combine the hand and fingers, making up only 2% of the total.
- Gestures involving objects with fingers are seen in 4% of cases, and foot gestures are also used, albeit minimally at 2%.
- Notably, multiple hands are employed in 13% of gestures, illustrating complex or coordinated activities.
- Lastly, gestures that incorporate both the head and objects account for 5%. Showcasing diverse and multifaceted uses in gesture recognition applications.
- This distribution highlights the versatility and complexity of human movements and how they are captured and interpreted in various technological solutions.
(Source: Jaypee University of Information Technology)
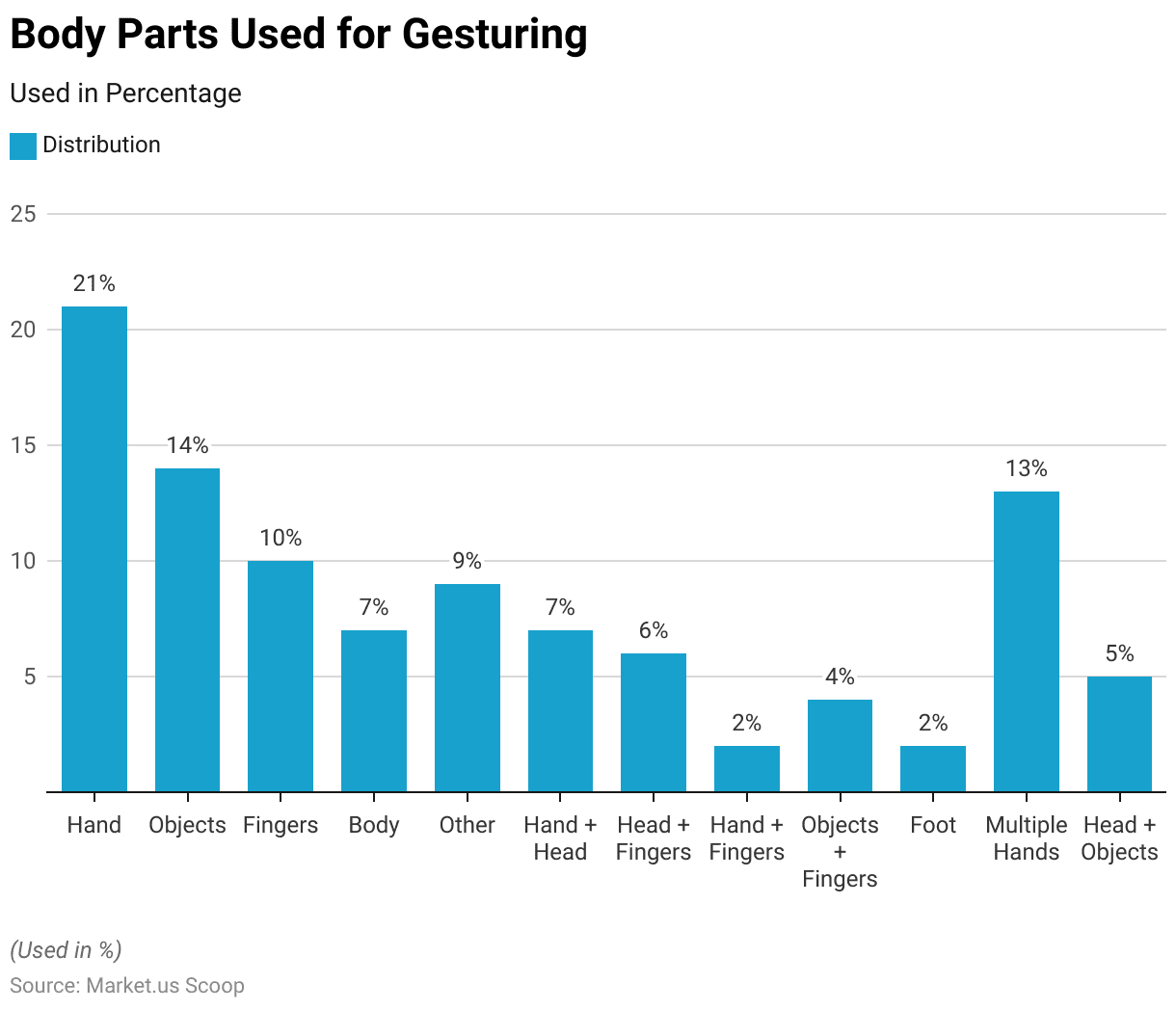
Gesture Recognition Market Statistics
Global Gesture Recognition Market Size Statistics
- The global gesture recognition market is projected to experience significant growth over the decade at a CAGR of 18.9%, starting from a revenue of $18.8 billion in 2022.
- This figure is expected to rise to $22.4 billion in 2023. Reflecting an increase driven by the advancing integration of gesture recognition technologies across various sectors.
- By 2024, the revenue is forecasted to reach $26.6 billion, followed by $31.6 billion in 2025.
- A continuous upward trend is anticipated, with market revenues reaching $37.6 billion in 2026 and $44.7 billion by 2027.
- The expansion is set to accelerate further, with the market expected to generate $53.1 billion in 2028, $63.2 billion in 2029, and $75.1 billion by 2030.
- The growth trajectory remains robust into the early 2030s, with projected revenues of $89.3 billion in 2031 and $106.2 billion by 2032.
- This sustained increase underscores the rising adoption and technological enhancements in gesture recognition systems.
(Source: market.us)
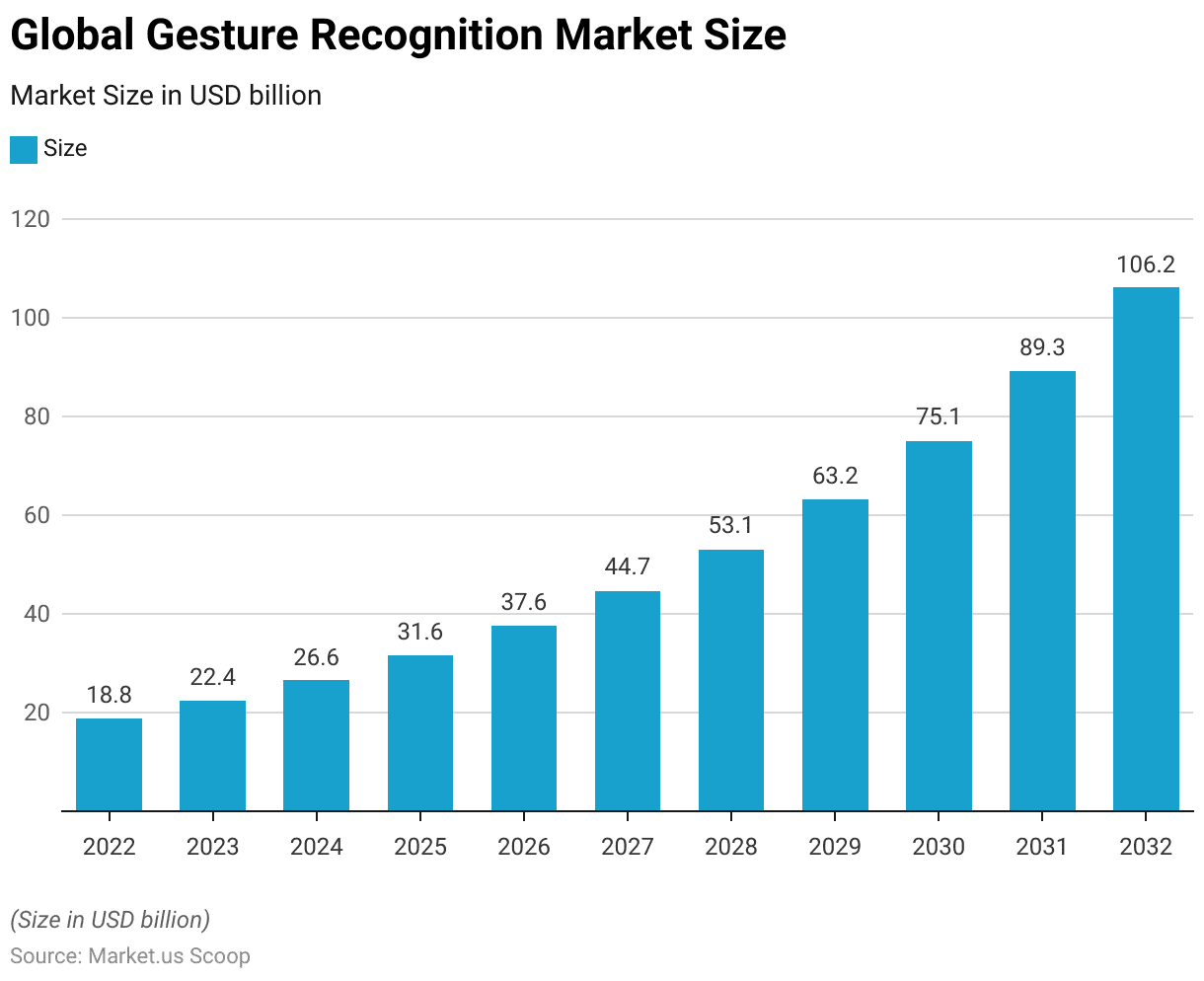
Gesture Recognition and Touchless Sensing Market Size Statistics
- The projected size of the global gesture recognition and touchless sensing market experienced substantial growth between 2014 and 2020.
- In 2014, the market was valued at approximately $5.15 billion.
- By 2020, this value had risen significantly to $23.55 billion.
- This dramatic increase highlights the rapid advancement and adoption of gesture recognition and touchless technologies over the period.
- Such growth is indicative of the expanding applications and integration of these technologies across various sectors. Driven by the rising demand for more intuitive and contact-free interaction solutions in consumer electronics, automotive, healthcare, and other industries.
(Source: Statista)
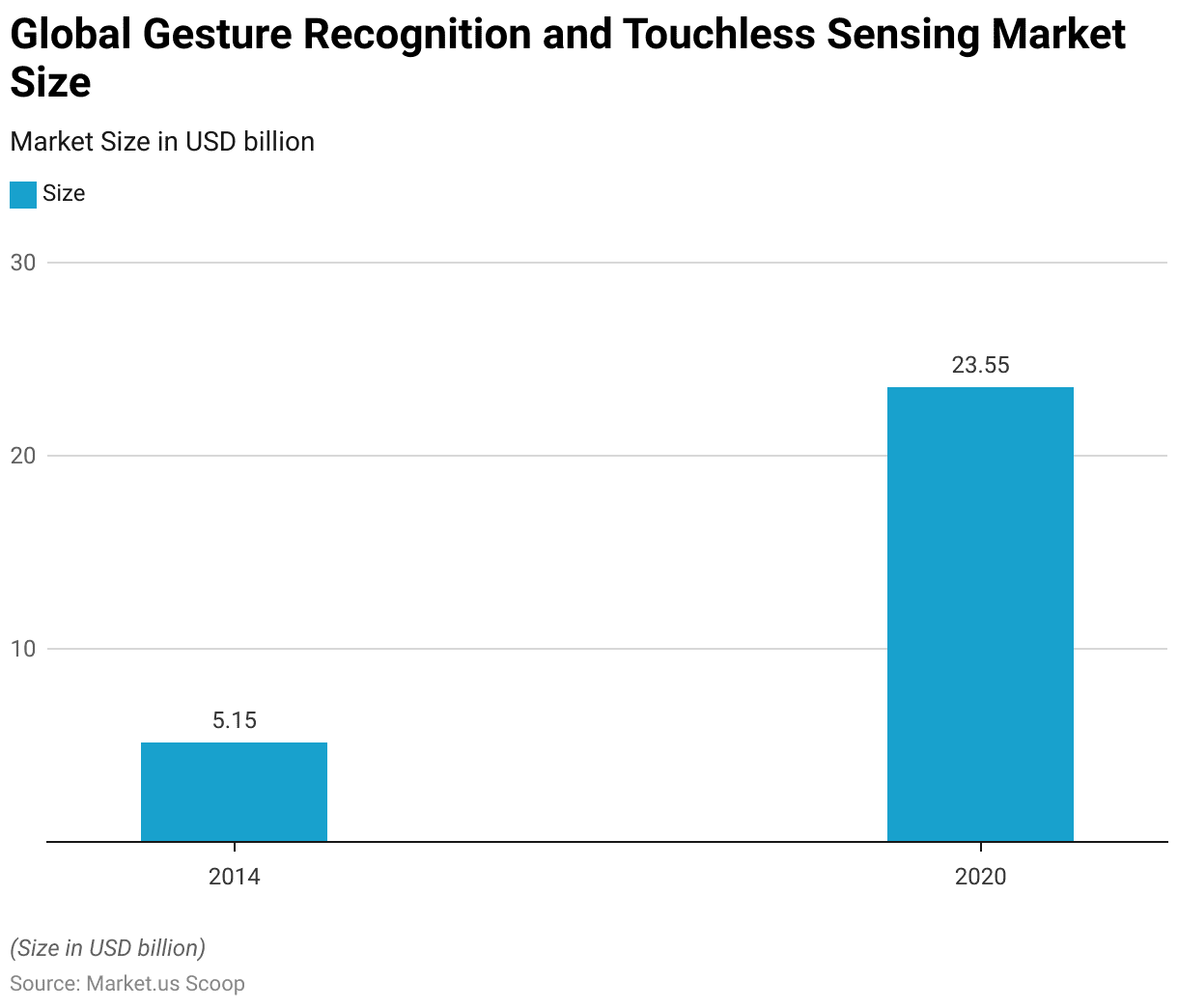
Global Gesture Recognition Market Size – By Product Type Statistics
2022-2026
- The revenue in the global gesture recognition market is segmented into touch-based and touchless products.
- In 2022, the total market revenue was $18.8 billion. Divided between touch-based systems generating $10.15 billion and touchless systems contributing $8.65 billion.
- By 2023, the total market size increased to $22.4 billion, with touch-based systems accounting for $12.10 billion and touchless systems at $10.30 billion.
- This trend of growth continues, with 2024 seeing total revenues of $26.6 billion. Split into $14.36 billion for touch-based and $12.24 billion for touchless.
- In the subsequent years, the growth trajectory remains positive; by 2025. Revenues reached $31.6 billion ($17.06 billion from touch-based and $14.54 billion from touchless).
- The market size expanded to $37.6 billion in 2026, with touch-based systems at $20.30 billion and touchless at $17.30 billion.
2027-2032
- By 2027, the revenue hit $44.7 billion ($24.14 billion from touch-based and $20.56 billion from touchless), and by 2028. It rose further to $53.1 billion ($28.67 billion from touch-based and $24.43 billion from touchless).
- This upward trend continued through the end of the decade and into the early 2030s; in 2029. The market size was $63.2 billion ($34.13 billion from touch-based and $29.07 billion from touchless).
- By 2030, it reached $75.1 billion, with touch-based systems making up $40.55 billion and touchless systems $34.55 billion.
- In 2031, the market expanded to $89.3 billion ($48.22 billion from touch-based and $41.08 billion from touchless).
- By 2032, it surged to $106.2 billion, with revenues of $57.35 billion from touch-based systems and $48.85 billion from touchless.
- This data underscores the robust growth and evolving landscape of the gesture recognition market. Reflecting increasing technological advancements and adoption rates.
(Source: market.us)
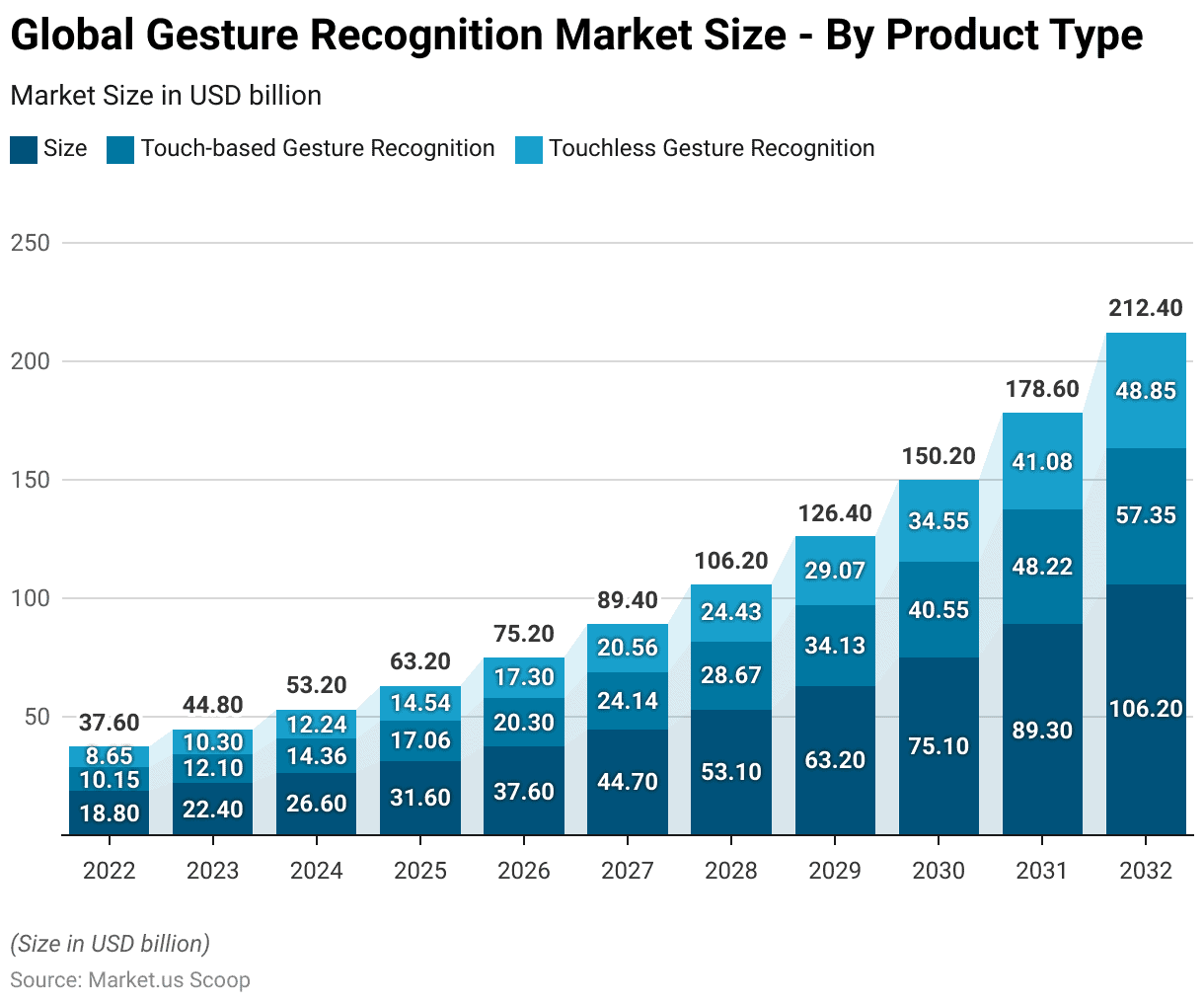
Global Gesture Recognition Market Share – By Technology Statistics
- In the global gesture recognition market, the distribution of market share by technology reveals distinct segments.
- Sensors hold the largest portion, accounting for 42% of the market. This indicates a strong preference and widespread adoption of sensor-based technologies within the industry.
- Meanwhile, 3D gesture technology captures a significant share as well, representing 33% of the market. This reflects the growing interest and investment in more sophisticated, depth-aware gesture recognition systems.
- On the other hand, 2D gesture technology, which tracks movements on a planar surface without depth, constitutes 25% of the market.
- This segmentation highlights the varied applications and technological preferences within the gesture recognition market. Showcasing a dynamic landscape with multiple technological approaches being utilized.
(Source: market.us)
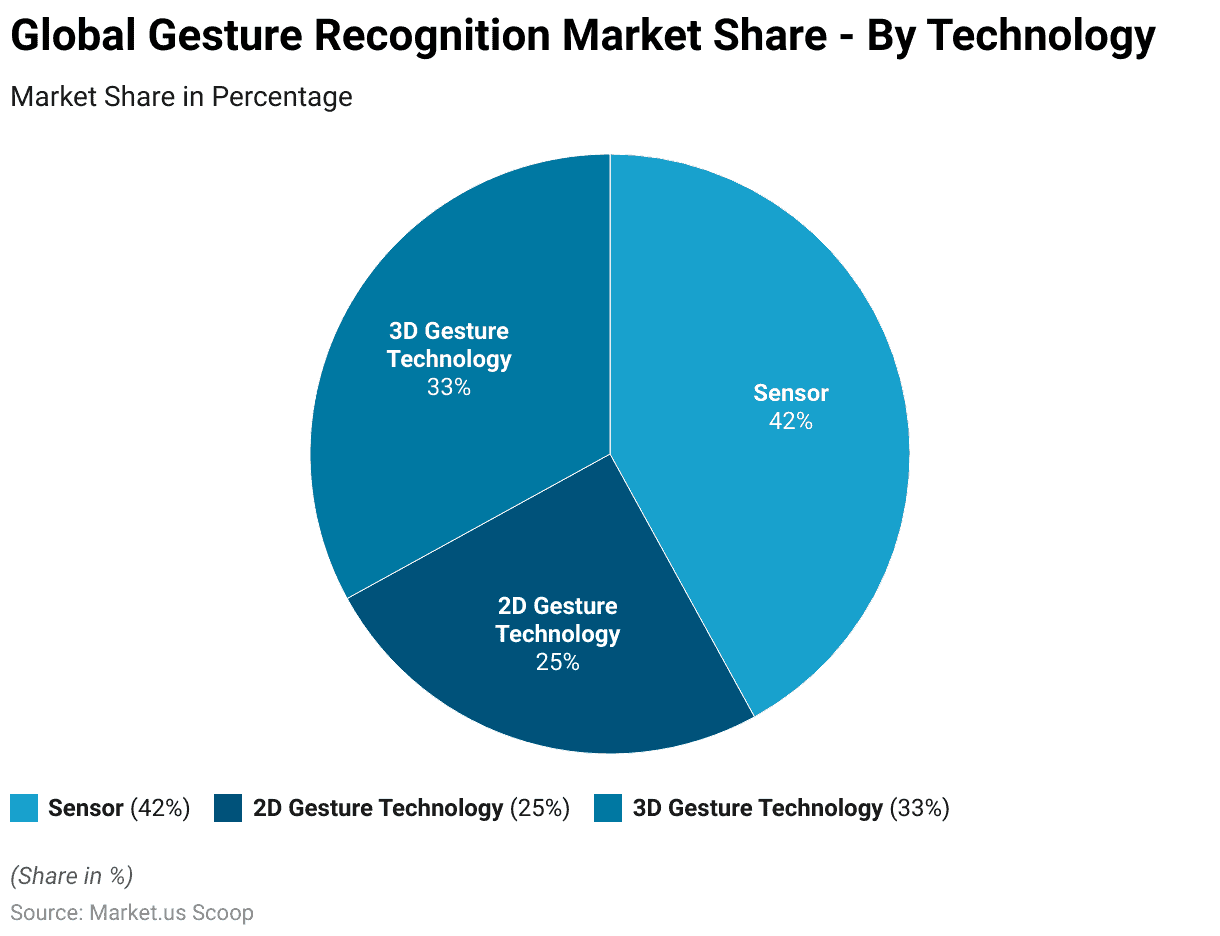
Gesture Recognition Systems and Datasets Statistics
- The landscape of existing sign language datasets is diverse and global. Encompassing a variety of countries, classes, subjects, and language levels.
- The SIGNUM dataset from Germany includes 450 classes, 25 subjects, and over 33,210 samples at a sentence level.
- In contrast, GSL 20 from Greece features only 20 classes with six subjects and 840 samples, focusing on individual words.
- The Boston ASL LVD in the USA is more extensive, with over 3,300 classes and 9,800 samples, and it is also at the word level.
- Poland’s PSL Kinect 30 is smaller, comprising 30 classes, one subject, and 300 samples.
- Argentina’s LSA64 offers 64 classes with ten subjects and 3,200 samples.
- The MSR Gesture 3D, another dataset from the USA, includes just 12 classes but has 10 subjects contributing to 336 samples.
- From China, the DEVISIGN-L dataset is substantial, with 2,000 classes, 8 subjects, and 24,000 samples. Belgium presents two datasets: LSFB-CONT and LSFB-ISOL.
- LSFB-CONT is particularly large, featuring 6,883 classes and over 85,000 samples from 100 subjects, straddling both word and sentence levels. LSFB-ISOL offers 400 classes and over 50,000 samples from 85 subjects.
- Lastly, the WLASL dataset from the USA (listed under the abbreviation EEUU) includes 2,000 classes, 119 subjects, and 21,083 samples, focusing on word-level recognition.
- This collection illustrates the robust efforts globally to capture and codify sign language in varied formats, serving as a critical resource for developing advanced gesture recognition systems.
(Source: arXiv)
Comparative Analysis of Gesture Recognition Systems by Recognition Rate and Methodology
- Recent analysis presents a comparative analysis of various gesture recognition systems, detailing different methods, input devices, segmentation types, features, feature vector representations, classification algorithms, and recognition rates.
- Tin H. employed a digital camera with threshold segmentation and non-geometric features using orientation histograms. Achieving a recognition rate of 90% through supervised neural networks.
- Manar M. utilized both a colored glove and digital camera, with an HSI color model segmentation. Featuring neural network systems such as the Elman recurrent network with a recognition rate of 89.66% and a fully recurrent network achieving 95.11%.
- Kouichi M. used a data glove and threshold segmentation with non-geometric features based on data items related to bending and coordinate angles. Employing a two-neural network system including a backpropagation network and Elman recurrent network, with recognition rates of 71.4% and 96%, respectively.
- Stergiopoulou E. leverages a digital camera with YCbCr color space segmentation and geometric features based on hand shape angles and palm distance. Analyzed via Gaussian distribution, reaching a 90.45% recognition rate.
Moreover
- Shweta employed a webcam with non-geometric features and a supervised neural network, though the recognition rate is not available.
- William F. Michal R. and Hanning Z. also used digital cameras, with the latter implementing threshold segmentation and an Euclidean distance metric, achieving a 92.3% recognition rate.
- Wysoski utilized skin color detection filters with geometric features in a digital camera setup. Achieving a high recognition rate of 98.7% through MLP and DP matching.
- Xingyan also employed a digital camera with threshold segmentation and non-geometric features. Reaching an 85.83% recognition rate through the Fuzzy C-Means algorithm.
- Keskiin C. used two colored cameras and a marker with a connected components algorithm. Delivering a 98.75% recognition rate through sequences of quantized velocity vectors analyzed by HMM.
- This summary underscores the diversity in gesture recognition technologies and approaches. Highlighting significant variations in system design and effectiveness.
(Source: International Journal of Computer Applications)
Overview of Publicly Available Benchmark Datasets for Deep Learning Evaluation in Gesture Recognition
- The landscape of publicly available benchmark datasets commonly used for evaluating deep learning methods in gesture recognition is diverse, spanning various years, devices, modalities, and metrics.
- The 20BN-jester dataset from 2019 utilizes laptop cameras or webcams, focusing on RGB modality, comprising 27 classes, 1,376 subjects, and a massive 148,092 samples, evaluated on accuracy.
- The Montalbano dataset (V2) from 2014, captured with Kinect v1 and incorporating RGB and depth (D) modalities, includes 20 classes, 27 subjects, and 13,858 samples, using the Jaccard index for evaluation.
- The ChaLearn LAP IsoGD dataset from 2016, also using Kinect v1 and RGB, D modalities, features 249 classes, 21 subjects, and 47,933 samples, assessed for accuracy.
- Introduced in 2017, the DVS128 Gesture dataset utilizes both DVS128 and webcam, with modality spanning spiking (S) and ultrasound (UM), including 11 classes, 29 subjects, and 1,342 samples, evaluated in a single scene for accuracy.
- The SKIG dataset from 2013, recorded with Kinect v1 in RGB modality, contains ten classes, six subjects, and 2,160 samples across three scenes. It is also measured on accuracy.
- Lastly, the EgoGesture dataset from 2018, using Intel RealSense and RGB, D modalities, encompasses 83 classes, 50 subjects, and 24,161 samples, evaluated across six scenes for accuracy.
- This array of datasets underscores the evolving technological and methodological approaches in the field of gesture recognition, highlighting their critical role in advancing and benchmarking deep learning solutions.
(Source: Science Direct)
Performance of Gesture Recognition Models Across Multiple Datasets Statistics
- The performance of various gesture recognition models across different datasets is showcased through a range of strategies and their corresponding accuracy or Jaccard index percentages.
- The CNN model applied to the Montalbano dataset achieved 78.90% accuracy, while DNN+DCNN on the same dataset scored slightly higher at 81.62%.
- The two-stream+RNN strategy also performed well on Montalbano, reaching 91.70% accuracy.
- In contrast, the Two-stream method tested on the 20BN-Jester dataset V1 notably achieved 96.28% accuracy.
- The C3D model on the ChaLearn LAP IsoGD dataset had a lower performance, with only 57.40% accuracy, and similar models with modifications such as C3D+Pyramid and ResC3D recorded even lower scores of 49.20% and 50.93%, respectively.
- RNN models demonstrated varied effectiveness; for example, a standard RNN reached 67.71% on the ChaLearn LAP IsoGD, while the ResC3D+Attention model significantly improved performance on Montalbano to 90.60% and even higher to 97.40% in another instance.
- Remarkably, the R3DCNN+RNN strategy achieved a perfect accuracy of 100% on the SKIG dataset.
- Models incorporating LSTM, such as C3D+LSTM, showed diverse outcomes ranging from 51.02% to 98.89% across different tests on the ChaLearn LAP IsoGD and SKIG datasets.
- These results underline the significant impact that model choice and dataset characteristics have on the performance of gesture recognition systems, highlighting the need for tailored approaches based on specific dataset attributes and recognition goals.
(Source: Science Direct)

Comparative Analysis of Accuracy Across Different Fusion Methods in Gesture Recognition
- The performance of various fusion methods in gesture recognition across multiple datasets highlights significant differences in accuracy and Jaccard index percentages.
- The Motion fused strategy applied to the 20BN Jester dataset V1 achieves a high accuracy of 96.28%.
- In contrast, the AvgFusion method used on the ChaLearn LAP IsoGD dataset shows a lower performance with 57.40% accuracy.
- The Concatenation strategy yields impressive results on the SKIG dataset, achieving an accuracy of 99.5%.
- The CCA (Canonical Correlation Analysis) method, also applied to the ChaLearn LAP IsoGD, scores slightly higher than AvgFusion at 58.65% accuracy.
- Further applications of Concatenation on the same dataset show varying results with 64.40% and 68.14% accuracies, demonstrating the variability of this method depending on specific conditions or configurations.
- Sparse fusion, tested on the ChaLearn LAP ConGD dataset, registers a notably lower accuracy of 26.55%, indicating potential limitations or mismatches with the dataset characteristics.
- ModDrop fusion used on the Montalbano dataset significantly improves performance to 88.90% accuracy.
- Additional uses of Concatenation on the ChaLearn LAP IsoGD and Montalbano datasets yield accuracies of 80.96% and 85.00%, respectively.
- These statistics elucidate the diverse outcomes that different fusion methods can achieve in gesture recognition, depending on the dataset and specific methodological implementations.
(Source: Science Direct)
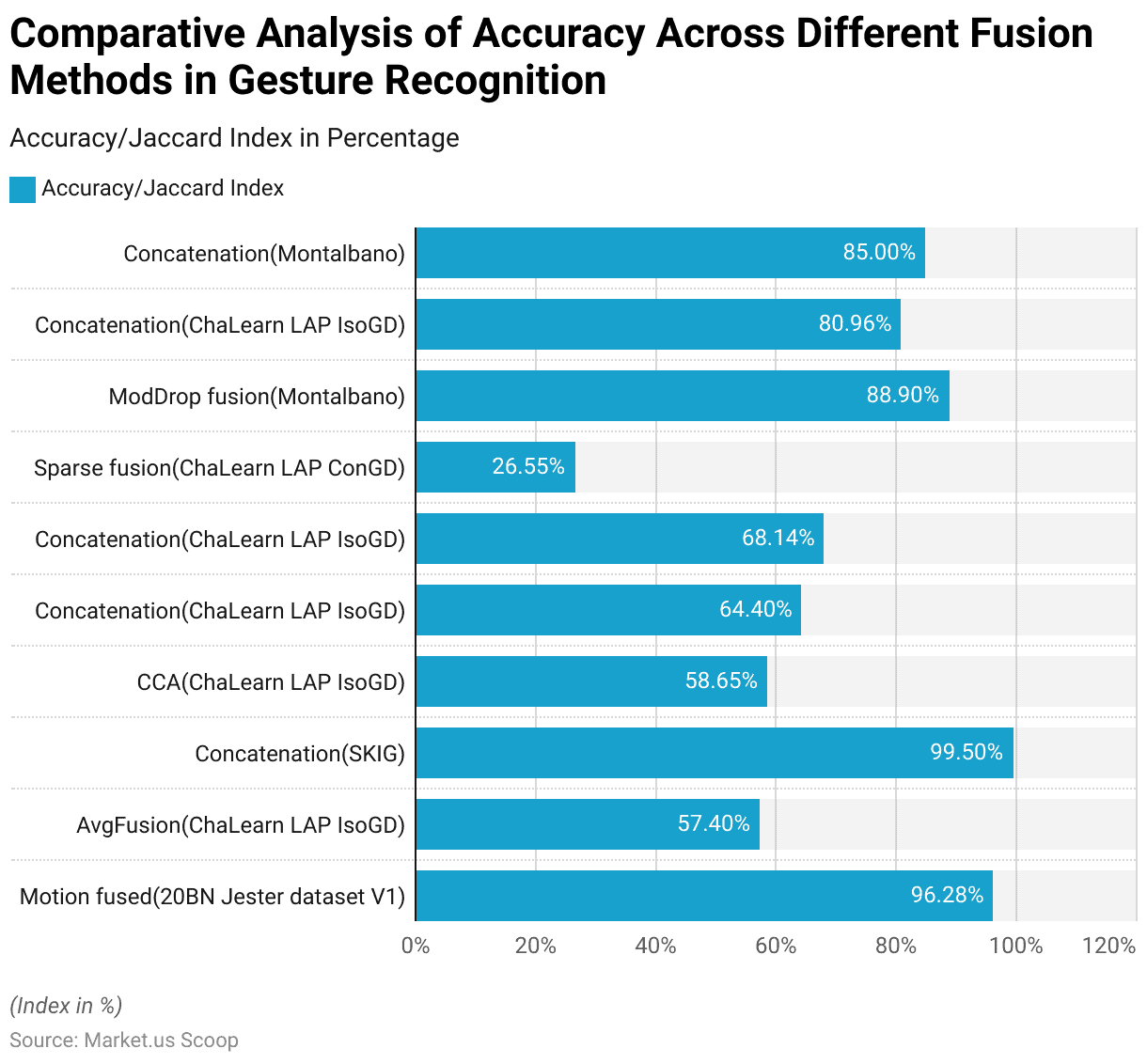
Sensors Used in Gesture Recognition Statistics
Global IoT Forecast: Sensors Market Breakdown – By Segment
- The projected global market for Internet of Things (IoT) enabled sensors in 2022 reveals a diverse distribution across different sensor types.
- Pressure sensors are anticipated to dominate the market with a substantial 36% share, reflecting their widespread applications in various industries.
- Temperature sensors also hold a significant portion, accounting for 26.2% of the market, indicative of their essential role in environmental monitoring and process control.
- Light sensors follow with a 17.27% market share, utilized broadly in applications such as automatic lighting control and safety systems.
- Chemical sensors, which are crucial for detecting and measuring chemical components in various environments, represent 10.21% of the market.
- Motion sensors, often used in security systems and smart devices, comprise 8.35% of the market.
- Lastly, other sensors, which include a variety of less common sensor types, make up approximately 1.97% of the market.
- This breakdown underscores the varied and critical applications of sensors in enhancing IoT solutions across multiple sectors.
(Source: Statista)
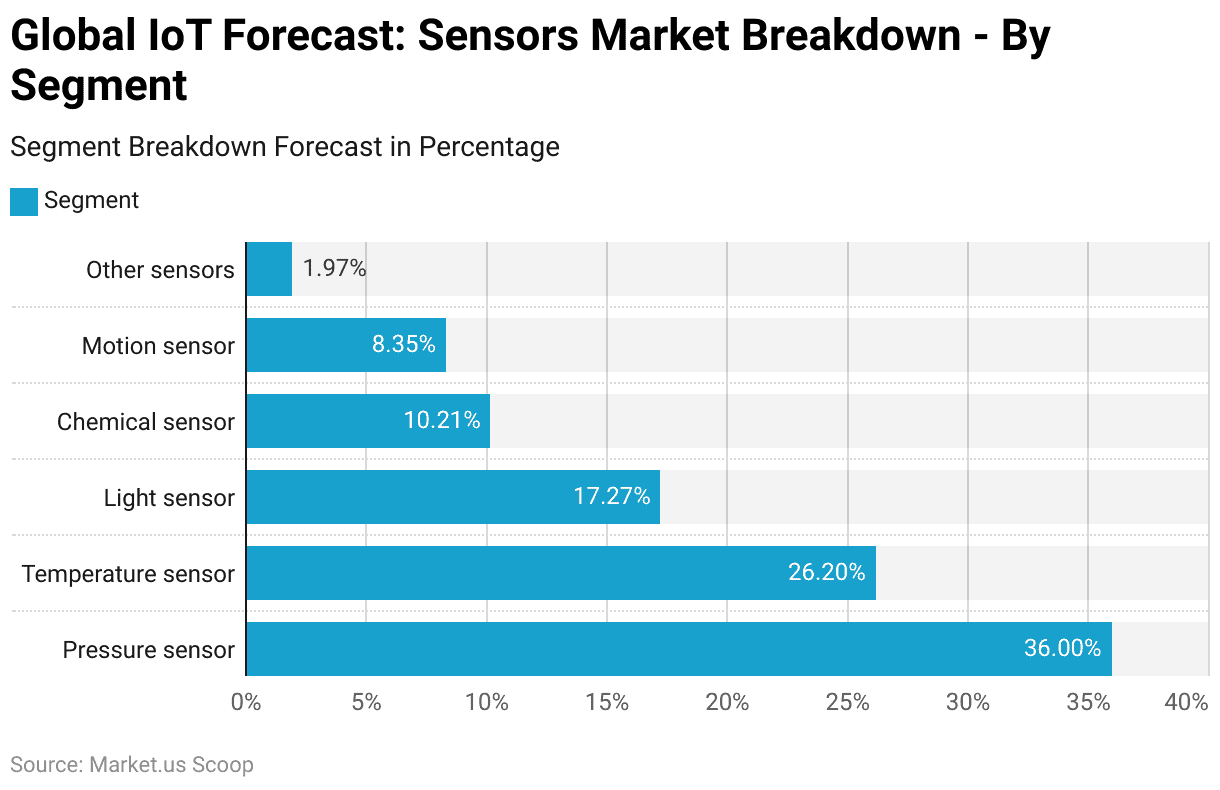
Connected Biosensors
- The global connected biosensors market has shown a significant upward trend in revenue from 2017 to 2029 at a CAGR of 5.70%.
- Starting at $4.37 billion in 2017, the market revenue increased to $6.19 billion in 2018 and further to $7.93 billion by 2019.
- A notable jump occurred in 2020, where revenue surged to $11.08 billion, followed by a more modest increase to $12.22 billion in 2021 and a slight rise to $12.27 billion in 2022.
- The growth continued steadily thereafter, reaching $13.5 billion in 2023, $14.63 billion in 2024, and $15.68 billion in 2025.
- The market is expected to keep growing, with projections showing an increase to $16.66 billion in 2026, $17.62 billion in 2027, $18.46 billion in 2028, and reaching $19.3 billion by 2029.
- This consistent growth trajectory highlights the increasing adoption and integration of connected biosensors in various healthcare applications, driven by technological advancements and the rising demand for real-time health monitoring.
(Source: Statista)
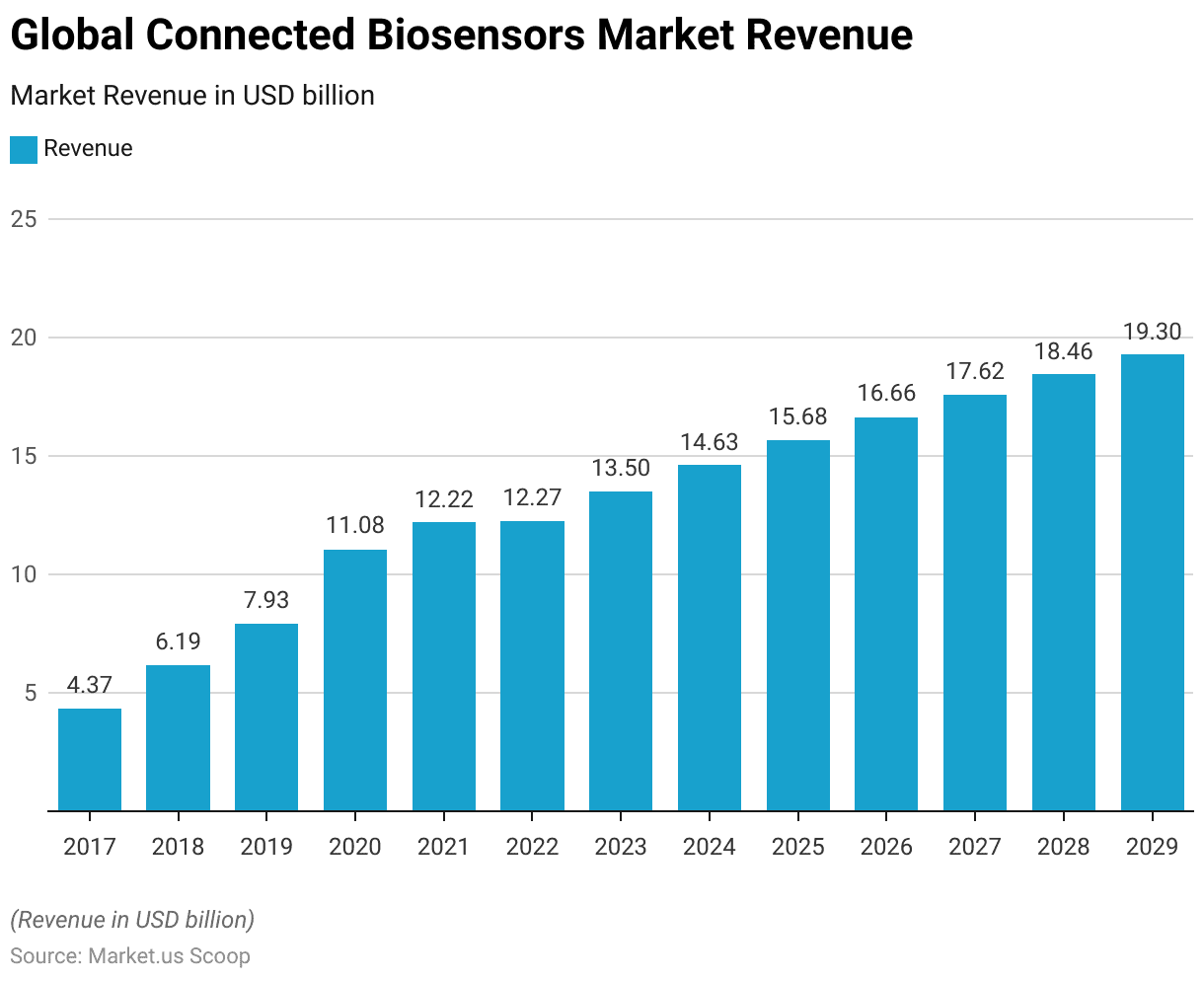
Automotive Sensors
- The global market for automotive sensors was valued at $30 billion in 2020, and it is projected to experience significant growth, reaching an estimated $55 billion by 2025.
- This substantial increase reflects the growing integration of advanced sensor technologies in vehicles., Driven by trends such as automation, connectivity, and enhanced safety features.
- The market’s expansion is indicative of the increasing reliance on sensors to support the complex functionalities and performance standards demanded in modern vehicles. Highlighting their critical role in the evolving automotive industry.
(Source: Statista)
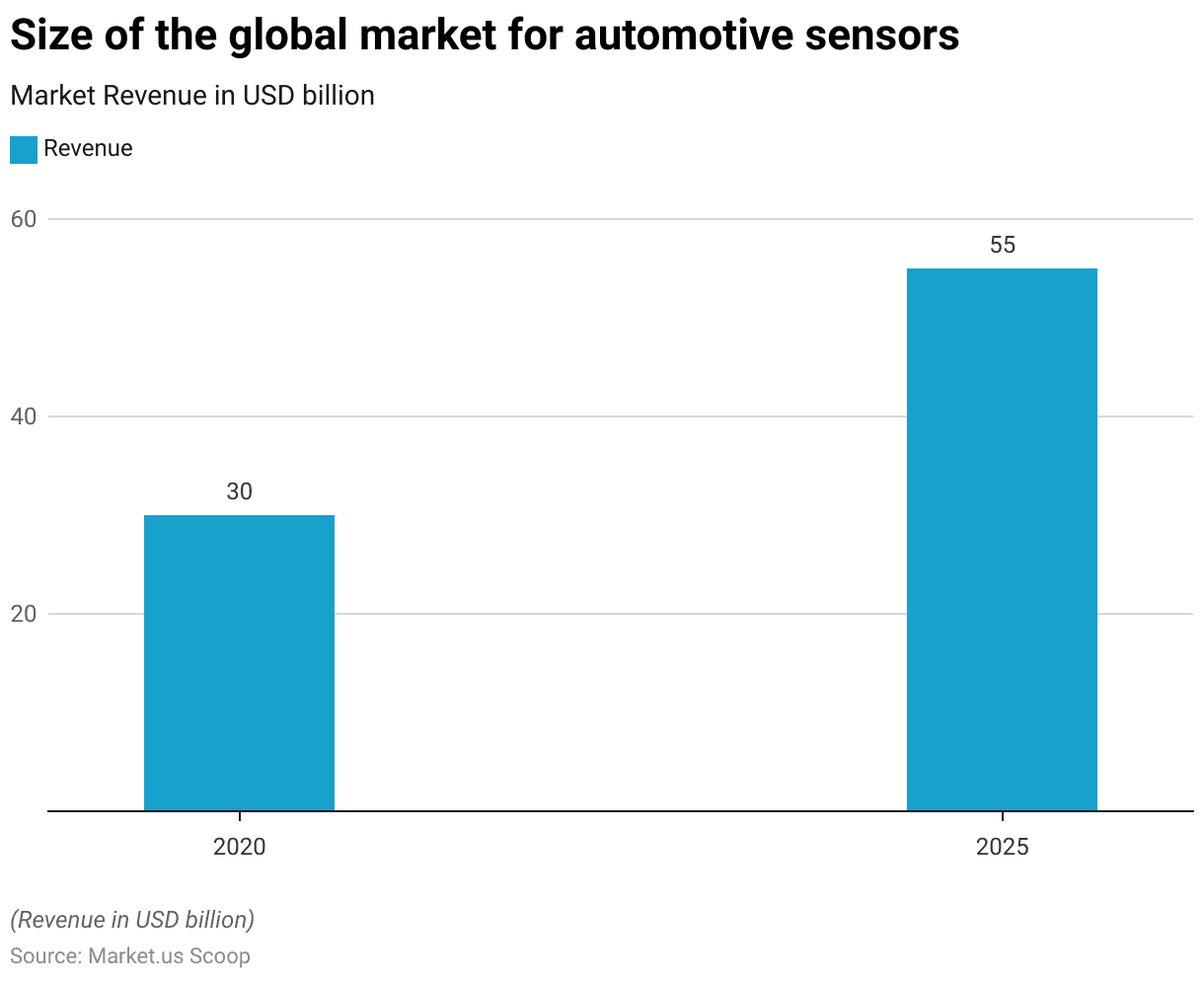
Studies Based on Data Environment Types in Hand Gesture Recognition Systems Statistics
- From 2014 to 2020, studies focusing on hand gesture recognition systems have primarily utilized three types of data environments.
- Single camera systems, being the most common, accounted for 53% of the studies. Reflecting their widespread adoption due to simplicity and cost-effectiveness.
- Active techniques, which might include methods like using depth sensors or infrared cameras, represented 39% of the studies. Indicating a substantial focus on more advanced, dynamic tracking technologies.
- Invasive techniques involving equipment or sensors that are attached directly to the user were the least utilized, comprising only 5% of the studies.
- This small percentage suggests a lesser preference for methods that require physical contact with the user, likely due to issues related to comfort and user acceptance.
(Source: IEEE Access)
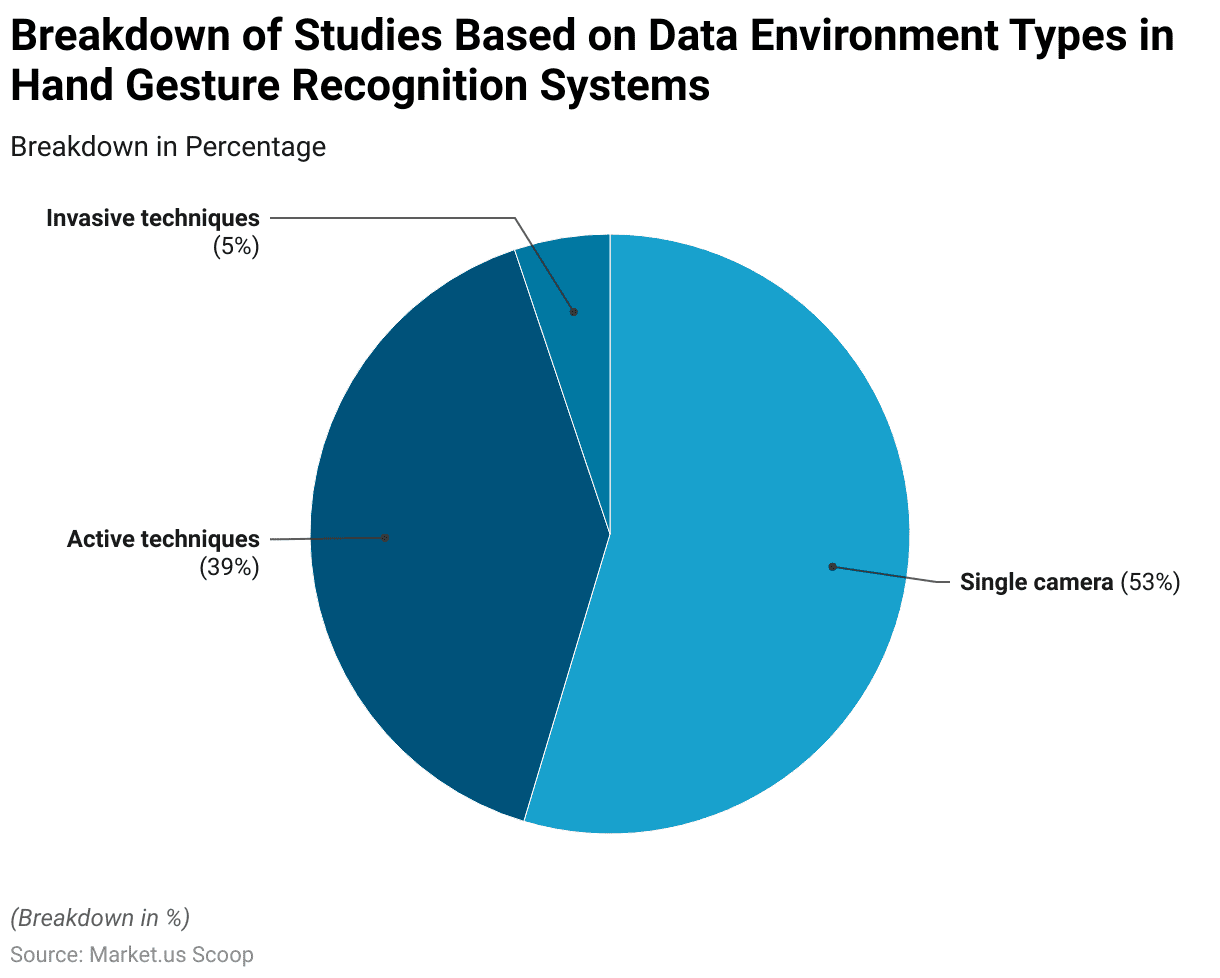
Studies Focusing on Hand Gesture Representations
- Between 2014 and 2020, studies on hand gesture representations in gesture recognition research showcased a clear preference for specific types.
- Fingerspelling was the most prevalent type, representing 67% of the studies. This dominance underscores the importance of discrete finger positioning and movements in the interpretation of gestures, particularly in contexts like sign language recognition.
- Isolated gestures, which involve distinct, separate movements or signs, accounted for 21% of the studies. Reflecting their significant role in applications where specific, clear-cut gestures are required.
- Continuous gestures involving fluid, ongoing movements were the least studied, making up only 12% of the research.
- This lesser focus may reflect the increased complexity and challenges associated with accurately recognizing and interpreting continuous hand motions compared to more defined and static gestures.
(Source: IEEE Access)

Innovations and Developments in Gesture Recognition Technology Statistics
- Recent advancements in gesture recognition technology have been focused on enhancing the interaction between humans and computers by enabling more natural. Intuitive communication methods without the need for physical contact.
- Innovations in this field include the development of dynamic gesture recognition systems that use sophisticated algorithms to interpret human gestures more accurately and efficiently.
- For instance, a novel approach has been implemented using the MediaPipe framework along with Inception-v3 and LSTM networks to process and analyze temporal data from dynamic gestures. Achieving a significant increase in accuracy up to 89.7%.
- This method notably improves performance by efficiently converting 3D feature maps into 1D row vectors, demonstrating its superiority over traditional models.
- Moreover, gesture recognition technology has expanded into various applications across different sectors. It is now being utilized in smart homes, medical care, and sports training. Where gestures can control devices, assist in rehabilitation, and enhance training programs.
- This technology employs a combination of sensor technologies, including electromagnetic, stress, electromyographic, and visual sensors, to capture and interpret gestures.
- Each technology offers specific advantages depending on the application scenario. Whether it’s for more precise medical assessments or robust controls in smart home environments.
- Further, these developments underline the continuous evolution of gesture recognition technologies. Making them more adaptable, robust, and capable of handling complex interaction scenarios without traditional input devices like keyboards and mice.
(Source: MDPI)
Regulations for Gesture Recognition Technology Statistics
- Moreover, gesture recognition technology, a subset of artificial intelligence, has seen a varied regulatory approach across different jurisdictions, aiming to balance technological advancement with concerns about privacy and civil liberties.
- In the United States, recent legislative proposals, such as the one introduced by Senators Kennedy and Merkley. Target specific uses of facial recognition by federal agencies. Suggesting a cautious approach towards privacy issues, particularly at airports.
- Similarly, the Department of Homeland Security has instituted new policies ensuring responsible AI usage, emphasizing compliance with privacy and civil rights standards.
- Meanwhile, other federal agencies like the FBI and the U.S. Customs and Border Protection have developed policies and training requirements to govern the use of such technologies. Highlighting a growing emphasis on operational protocols that protect civil liberties while employing these advanced tools.
- These developments reflect a broader trend toward establishing a framework that can accommodate the rapid evolution of biometric technologies while addressing the critical ethical implications they present.
(Sources: The Tech Edvocate, U.S. Department OF Homeland Security, GAO)
Recent Developments
Acquisitions and Mergers:
- Apple acquires PrimeSense: In 2023, Apple completed the acquisition of PrimeSense, a company specializing in 3D gesture recognition technology, for $345 million. This acquisition is expected to enhance Apple’s augmented reality (AR) and gesture-based control capabilities across its product line, particularly in AR and wearable devices.
- Microsoft acquires GestureTek: In early 2024, Microsoft acquired GestureTek, a leading developer of gesture recognition solutions, for $250 million. The acquisition strengthens Microsoft’s Kinect and HoloLens platforms by incorporating advanced gesture recognition technologies for gaming, education, and workplace solutions.
New Product Launches:
- Google introduces Soli Radar Gesture Technology: In late 2023, Google introduced its Soli radar-based gesture recognition technology to the Pixel 8 smartphone. Allowing users to control their devices through hand gestures without touching the screen. This new feature is designed for accessibility and convenience. Enabling tasks like changing music tracks and dismissing calls with simple hand motions.
- Sony launches gesture-controlled gaming: In mid-2023, Sony introduced a gesture-based gaming system for the PlayStation 5. This system enables players to control in-game movements and interactions through hand gestures. Enhancing immersive gaming experiences, and is expected to boost gaming industry demand for gesture recognition by 15% by 2025.
Funding:
- Leap Motion secures $50 million for gesture recognition technology: In 2023, Leap Motion, a pioneer in gesture recognition and hand-tracking technology. Raised $50 million in a funding round to further develop its AR and VR gesture interfaces. This funding will help Leap Motion integrate its technology into more consumer electronics, including wearables and smart home devices.
- Ultraleap raises $75 million for hand-tracking and gesture control: In 2023, Ultraleap, a company specializing in hand-tracking and gesture-control technology. Raised $75 million to expand its product offerings in the automotive, gaming, and retail sectors. Ultraleap aims to lead in touchless interfaces and increase its market share in gesture recognition technologies.
Technological Advancements:
- AI-powered gesture recognition: By 2025, it is projected that 45% of gesture recognition systems will incorporate AI for enhanced accuracy and responsiveness. AI integration will enable better real-time gesture analysis in applications like automotive controls, smart homes, and healthcare.
- Gesture recognition in automotive: Gesture control is gaining traction in the automotive industry, with 30% of new vehicles by 2026 expected to offer gesture-based controls for tasks such as adjusting temperature, media controls, and navigation.
Conclusion
Gesture Recognition Statistics – Gesture recognition technology has advanced significantly. Fueled by developments in machine learning, computer vision, and sensors, facilitates intuitive human-machine interactions.
Research predominantly utilizes single-camera systems due to cost-effectiveness, while active and invasive techniques are explored less frequently.
Studies largely focus on finger spelling, which is crucial for precise gesture interpretation. Especially in sign language, with isolated and continuous gestures also examined for their roles in simple and dynamic interactions, respectively.
The expanding market for gesture recognition is driven by its adoption across automotive, healthcare, and consumer electronics. Where demand for innovative interfaces and safety enhancements grows.
Future advancements are anticipated with the integration of AI, aiming to refine accuracy and expand applicability. Although challenges like diverse environmental adaptability and extensive training data requirements remain.
FAQs
Gesture recognition technology is a field of computer science that enables machines to interpret human gestures as commands. These gestures can be captured via sensors or cameras and then processed using algorithms to perform specific actions.
The process involves capturing motion or gesture data through sensors or cameras. Which is then analyzed by software to identify specific gesture patterns. These patterns are compared against a pre-defined set of gestures to determine the intended command.
The two primary types are touch-based and touchless. Touch-based gestures require physical contact with a device, while touchless gestures involve Motion captured through cameras or infrared sensors.
Gesture recognition is widely used in various sectors, including gaming, automotive, consumer electronics, and healthcare. It enhances user interfaces by allowing for hands-free control, thereby improving accessibility and user experience.
Benefits include enhanced user interaction, increased accessibility, improved safety in environments where touch is impractical or hazardous, and the ability to control devices more naturally and intuitively.
Discuss Your Needs With Our Analyst
Please share your requirements with more details so our analyst can check if they can solve your problem(s)
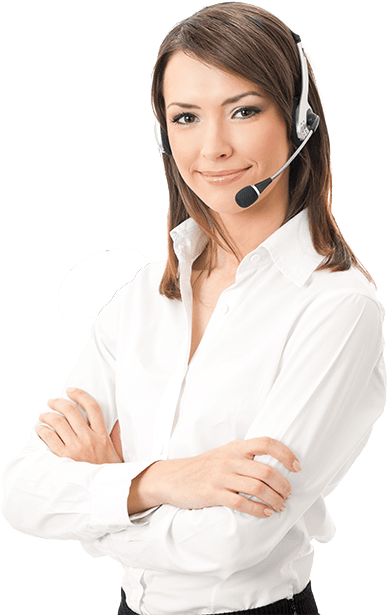