Table of Contents
Introduction
The Machine Learning Operations (MLOps) Market is witnessing substantial growth, poised to reach a remarkable valuation of USD 75.42 Billion by 2033, reflecting a notable Compound Annual Growth Rate (CAGR) of 43.2% from 2024 to 2033.
MLOps is about making sure that machine learning models are implemented and maintained properly so that they can be used effectively in real-world applications. It involves tasks such as preparing data for training, training the models, deploying them in production systems, monitoring their performance, and updating them when necessary.
The MLOps market has observed significant growth, propelled by the increasing adoption of artificial intelligence and machine learning technologies across diverse sectors, including finance, healthcare, and retail. This growth can be attributed to the demand for more efficient ways to deploy, monitor, and manage machine learning models, thereby reducing time-to-market and improving the reliability and performance of AI applications.
However, the MLOps market faces several challenges. One of the primary hurdles is the complexity involved in integrating machine learning models with existing IT infrastructure and workflows, requiring substantial changes to both technology stacks and operational processes. Additionally, the scarcity of skilled professionals with expertise in both machine learning and operational deployment further complicates the adoption of MLOps practices. Security and compliance issues also pose significant challenges, as machine learning applications often process sensitive data, necessitating rigorous data protection measures and adherence to regulatory standards.
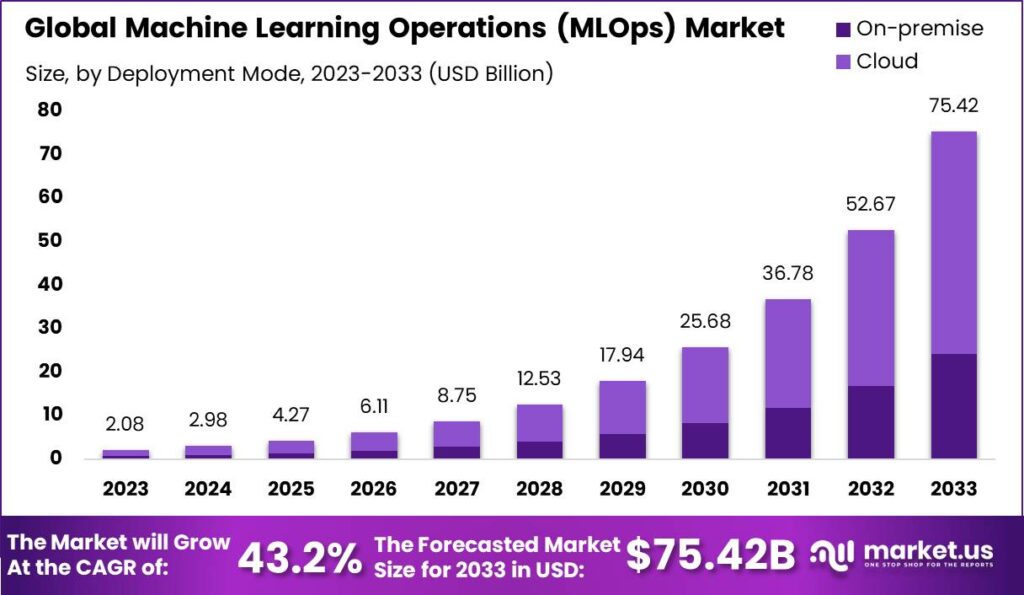
To learn more about this report – request a sample report PDF
Key Takeaways
- The global MLOps market is anticipated to reach a valuation of USD 75.42 Billion by 2033, exhibiting a remarkable Compound Annual Growth Rate (CAGR) of 43.2% from 2024 to 2033.
- In 2023, the Platform segment emerged as the frontrunner, commanding a market share exceeding 70%. This dominance is attributed to the versatility and user-friendly interfaces of automated machine learning (AutoML) platforms.
- As of 2023, the Cloud segment emerged as the leader, capturing over 68% of the market share. Its scalability and flexibility make it the preferred choice for organizations seeking agile MLOps solutions.
- Large Enterprises held a dominant market position in 2023, capturing more than 71% of the market share. Their substantial resources and technical capabilities enable efficient implementation and scaling of MLOps initiatives.
- The Banking, Financial Services, and Insurance (BFSI) sector led the adoption of MLOps, capturing over 20% of the market share in 2023. MLOps is pivotal in enhancing data analytics, risk management, and personalized customer services in this sector.
- North America held a dominant market position, capturing more than a 41% share in 2023. Europe closely follows North America in the MLOps market, with the Asia-Pacific region experiencing rapid growth driven by digital transformation initiatives.
Machine Learning Operations (MLOps) Statistics
- In 2024, there was a notable surge in the adoption of open-source MLOps platforms, with platforms like Kubeflow and MLflow experiencing a 35% increase.
- An IBM study shed light on the operational benefits of embracing MLOps, indicating that organizations integrating MLOps practices into their operations witnessed an average improvement of 15% in the accuracy of their machine learning models.
- The year 2023 marked a substantial growth phase for Microsoft Azure’s suite of MLOps tools, including Azure Machine Learning, with a reported 40% increase in adoption.
- A substantial proportion of companies, quantified at 71%, report an inability to harness machine learning (ML) effectively at scale, culminating in a pronounced deficit in revenue generation and value creation.
- A clear intention to augment investments in MLOps is evident among surveyed organizations.
- Specifically, 42% of respondents disclosed plans to enhance their MLOps expenditure by 11-25%.
- A further 37% are aiming for an investment increase of 26-50%, indicating a robust confidence in the value proposition offered by MLOps.
- An additional segment, comprising 16% of the surveyed entities, plans to elevate their spending by 51-75%.
- Meanwhile, a smaller fraction, at 5%, anticipates a surge in investment by 76-100%.
- Only a minimal 2% of respondents foresee a modest increment of 10% or less in their MLOps budget.
- An overwhelming consensus among respondents, at 98%, signals a plan to boost their investment by a minimum of 11%.
- Notably, 58% of participants project an increase in spending by over 25%, underscoring a significant commitment to enhancing MLOps capabilities.
- MLOps is credited with the potential to dramatically expedite the production timeline of ML applications by up to 90%.
- Concurrently, it is poised to substantially diminish the requisite resources for development, presenting a dual advantage of speed and efficiency.
- A prevailing challenge, identified by 86% of organizations, revolves around the effective utilization of ML to foster tangible business value.
- This aligns with the earlier insight regarding the struggle of 71% of companies to generate revenue due to the hurdles in implementing ML at scale.
Emerging Trends
- Automation in Model Deployment: The trend towards automating the deployment of machine learning models is gaining momentum. This enables businesses to streamline their operations and reduce the time from development to deployment, enhancing agility.
- Increased Focus on Model Monitoring: There’s a growing emphasis on continuously monitoring machine learning models to ensure they perform as expected over time. This includes identifying when models decay and need retraining or adjustments.
- Rise of Explainable AI: As machine learning models become more complex, there’s a push for explainable AI, which aims to make these models more transparent and understandable. This is crucial for gaining trust and meeting regulatory requirements.
- Integration of DevOps Practices: The integration of Development Operations (DevOps) practices with machine learning workflows, known as MLOps, is becoming more prevalent. This promotes collaboration between data scientists and IT professionals, improving the efficiency of model development and deployment.
- Adoption of Cloud-Based Solutions: The shift towards cloud-based platforms for MLOps is accelerating. These platforms offer scalability, flexibility, and access to advanced computational resources, facilitating the development and deployment of machine learning models.
Top Use Cases
- Predictive Maintenance: MLOps is extensively used in predictive maintenance, helping industries predict machinery failures before they happen, thereby saving costs and reducing downtime.
- Customer Churn Prediction: Businesses leverage MLOps to predict customer churn, allowing them to implement strategies to retain customers and enhance satisfaction.
- Fraud Detection: In the financial sector, MLOps plays a crucial role in detecting fraudulent activities by analyzing transaction patterns and identifying anomalies.
- Personalized Recommendations: E-commerce and streaming services use MLOps to provide personalized recommendations to users, enhancing user experience and engagement.
- Supply Chain Optimization: MLOps is used to optimize supply chains, predicting demand, and managing inventory more efficiently, ensuring smoother operations.
Major Challenges
- Data Privacy and Security: Ensuring the privacy and security of data used in machine learning models is a significant challenge, especially with stringent regulations like GDPR.
- Skill Gap: The shortage of skilled professionals proficient in MLOps presents a barrier to the adoption and implementation of these technologies.
- Model Bias and Fairness: Addressing biases in machine learning models to ensure they are fair and do not perpetuate inequalities is a major concern.
- Complexity in Model Management: Managing the lifecycle of machine learning models, including versioning and updating, can be complex and resource-intensive.
- Integration with Existing Systems: Integrating MLOps practices with existing IT systems and workflows can be challenging, requiring significant time and effort.
Market Opportunity
- Healthcare Sector: The application of MLOps in healthcare, for predictive diagnostics and personalized treatment plans, presents a significant market opportunity.
- Financial Services: There’s a growing demand for MLOps in finance for risk assessment, fraud detection, and algorithmic trading, offering substantial market prospects.
- Retail and E-commerce: MLOps can revolutionize retail and e-commerce through demand forecasting, inventory management, and personalized customer experiences.
- Manufacturing: In manufacturing, MLOps offers opportunities for predictive maintenance, quality control, and supply chain optimization.
- Smart Cities and Infrastructure: The use of MLOps in developing smart cities, optimizing traffic management, and enhancing public safety presents emerging market opportunities.
Predictions for 2024
- Evolution of MLOps: The MLOps domain is anticipated to continue its evolutionary path, effectively bridging the existing gap between ML development and deployment. This evolution is underpinned by the increasing demand for seamless integration of machine learning models into operational business processes, thereby enhancing efficiency, scalability, and the agility of AI-driven solutions.
- Governance and Compliance: There will be an intensified focus on governance and compliance frameworks within the MLOps sphere to ensure the development of responsible AI. This trend reflects the growing awareness of ethical considerations, data privacy laws, and the need for transparent, accountable AI systems. The implementation of robust governance and compliance mechanisms is expected to mitigate risks and enhance trust in AI applications.
- Automated Model Monitoring and Management: The automation of model monitoring and management is projected to gain prominence. This involves leveraging advanced tools and technologies to continuously monitor the performance of machine learning models, identify degradation, and manage model updates without significant manual intervention. Automation in this area promises to enhance the operational efficiency and reliability of machine learning systems.
- Hybrid and Multi-Cloud Solutions: Hybrid and multi-cloud MLOps solutions are set to become indispensable in the contemporary AI landscape. These solutions offer flexibility, enabling organizations to deploy and manage machine learning models across various cloud environments and on-premises infrastructure. This trend is driven by the need for scalable, resilient, and customizable MLOps platforms that can accommodate diverse computational and data storage requirements.
- Collaboration Tools: The integration of collaboration tools within the MLOps workflow is anticipated to facilitate seamless teamwork among data scientists, ML engineers, and operational staff. These tools are designed to streamline communication, project management, and the sharing of resources and insights. Enhanced collaboration is essential for the iterative development and deployment of machine learning models, ensuring alignment with business objectives and user needs.
Recent Developments
- April 2023: ClearML, a leading provider of MLOps solutions, responded to the growing demand in global markets by launching new capabilities for continuous machine learning (ML). They introduced the Sneak Peek application, a functionality that allows ClearML enterprise users to directly deploy applications from their development ecosystem. This enhancement aims to streamline the deployment process and meet the evolving needs of ML practitioners.
- November 2023: DataRobot, a prominent player in the ML industry, formed a strategic alliance with Cisco and partnered with Evolutio to develop an MLOps solution for the Cisco FSO (Full-Stack Observability) platform. This collaborative effort resulted in a comprehensive solution that provides business-grade observability for generative AI and predictive AI. It offers optimization and scalability for ML deployments, ultimately delivering enhanced business value to customers.
- April 2023: MLflow, an open-source ML platform, introduced MLflow 2.3, an upgraded version with new features and LLMOps (Large Language Model Operations) support. This update empowers users to deploy and manage large language models more effectively, incorporating them seamlessly into their ML operations. The addition of LLMOps expands the platform’s capabilities and supports the growing demand for managing and deploying complex language models.
- March 2023: Striveworks, in collaboration with Microsoft, partnered to offer the Chariot MLOps platform for the public segment. This integration enables organizations to leverage Striveworks’ Chariot platform to handle their complete model lifecycle on the scalable infrastructure of Microsoft Azure. The partnership aims to provide users with a robust MLOps solution that leverages the scalability and reliability of Azure.
Conclusion
The MLOps (Machine Learning Operations) market is experiencing significant growth and is becoming increasingly important in the field of artificial intelligence and machine learning. MLOps refers to the practices and tools used to streamline and automate the deployment, monitoring, and management of machine learning models in production environments. It aims to bridge the gap between data scientists and IT operations, ensuring that machine learning models can be deployed and maintained effectively.
In recent years, the adoption of machine learning has surged across various industries, driving the demand for MLOps solutions. Organizations recognize the need to operationalize their machine learning workflows to ensure scalability, reliability, and governance. MLOps helps in optimizing the entire lifecycle of machine learning models, from development to deployment and ongoing maintenance.
Discuss your needs with our analyst
Please share your requirements with more details so our analyst can check if they can solve your problem(s)
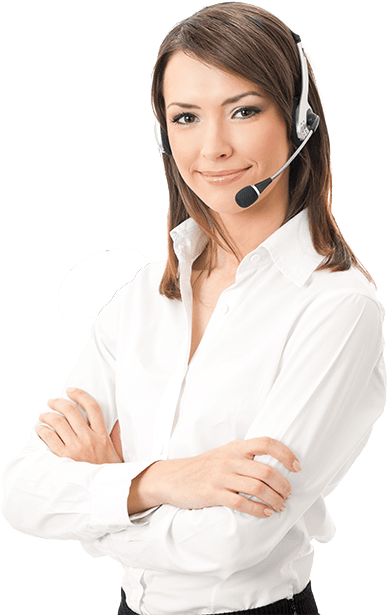