Table of Contents
The Global Predictive Maintenance Market is undergoing a period of significant growth, fueled by advancements in technologies such as the Internet of Things (IoT), machine learning, and data analytics. As of 2023, the market size was projected at USD 8.7 billion, with forecasts suggesting an increase to USD 11.2 billion by the end of 2024. This trend is expected to continue, showcasing a robust Compound Annual Growth Rate (CAGR) of 28.5%, potentially reaching USD 107.3 billion by 2033.
Several key factors contribute to this expansion. Industries with heavy assets and high downtime costs, such as oil & gas, chemicals, mining & metals, are increasingly adopting predictive maintenance solutions. These industries are driving demand due to the critical need for efficient and effective maintenance strategies that minimize downtime and reduce operational costs. Moreover, partnerships between major corporations like Microsoft Corporation and Procter & Gamble Company (P&G) to expand digital manufacturing platforms underline the sector’s growth. These collaborations are enhancing predictive quality, maintenance, and sustainability optimization.
The predictive maintenance market is characterized by its innovative approaches to maintenance, which include indirect failure prediction, anomaly detection, and estimating the Remaining Useful Life (RUL) of assets. Each of these methodologies offers unique benefits and challenges, with anomaly detection gaining prominence due to its scalability and lower data requirements. Recent developments underscore the increasing integration of predictive maintenance with enterprise systems, the application of predictive analytics for complex systems, and a focus on proactive maintenance strategies. Advancements such as digital twin technology, cross-industry collaboration, and enhanced sensor technologies are poised to further drive market growth.
Key Takeaways
- The Predictive Maintenance Market is projected to be valued at USD 11.2 billion by the end of 2024.
- By 2033, the Predictive Maintenance Market is anticipated to reach a significant valuation of USD 107.3 billion.
- During the forecast period, the Predictive Maintenance Market is expected to exhibit a remarkable Compound Annual Growth Rate (CAGR) of 28.5%.
- In 2023, the Solution segment dominated the market with over 83% of the market share.
- On-premise deployment solutions accounted for more than 71% of the market share in 2023.
- Large Enterprises held a dominant market position with a share exceeding 75% in 2023.
- The Manufacturing segment led the Predictive Maintenance Market in 2023 with a share exceeding 29%.
- North America emerged as a dominant force in the Predictive Maintenance Market in 2023, capturing over 35% of the market share.
- The demand for Predictive Maintenance in North America was valued at USD 3.05 billion in 2023.
- Europe’s market share in 2023 stood at an impressive level.
- Latin America made steady progress in embracing predictive maintenance, with a focus on industries like mining, oil and gas, and utilities.
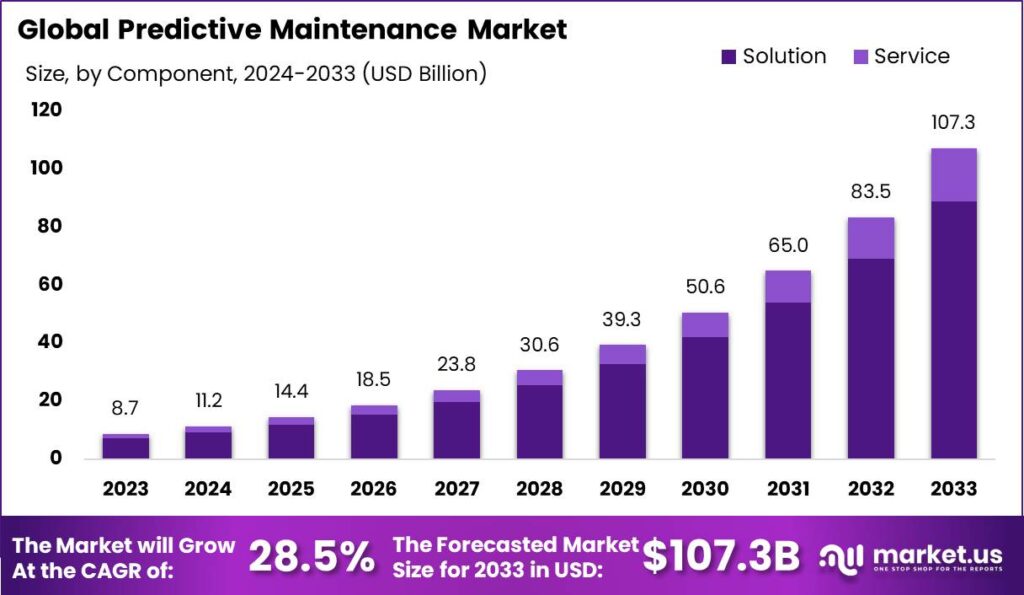
Predictive Maintenance Statistics
- Predictive maintenance usage increased from 47% to 51%.
- The trend of running equipment until failure decreased from 61% to 57%.
- The global market for enterprise asset management software is valued at $4 billion, growing at an 11% CAGR yearly.
- About 80% of CMMS (Computerized Maintenance Management System) users don’t fully utilize all available functions.
- For one company in 2017, using data visualization software reduced production hours by 320 and increased production by 15%.
- The global CMMS software market is expected to reach $1.26 billion by 2026, with a 9.8% CAGR from 2020 to 2026.
- Approximately 33% of respondents find new software adoption challenging.
- Only about 10% of industrial equipment actually wears out, indicating many mechanical failures are preventable.
- Total productive maintenance can increase plant capacity by over 10% and productivity by 50%, yet over half of all attempts at implementing it fail.
- Leading causes of unscheduled equipment downtime include aging equipment (34%) and mechanical failure (20%).
- Predictive maintenance is cost-effective, saving about 8% to 12% over preventive maintenance, and up to 40% over reactive maintenance.
- Maintenance costs typically range between 15% and 40% of total production costs.
- As of 2018, about 53% of facilities use a CMMS for maintenance monitoring.
- In 2017, 78% of companies using a CMMS saw improvements in equipment life.
- Up to 80% of CMMS implementations fail.
- Preventive maintenance is preferred by 80% of maintenance personnel, but the use of predictive maintenance rose from 47% in 2017 to 51% in 2018.
- Maintenance teams face challenges in hiring, onboarding, and retention (48%), streamlining processes (27%), and successful technology adoption (25%).
- Predictive maintenance can reduce downtime by 30% to 50% and increase machine life by 20%.
Use Cases
Manufacturing
In the manufacturing sector, predictive maintenance (PdM) is revolutionizing process digitization and industrial automation. By leveraging IoT data and analytics, manufacturers are able to anticipate machinery failures, schedule maintenance efficiently, and reduce unplanned downtimes, thus maximizing production uptime and profitability.
Energy
The energy industry benefits from AI and ML-driven PdM by transitioning from static, time-based maintenance routines to dynamic, intelligence-based approaches. This enables energy companies to utilize both historical and real-time data for precise asset forecasting, enhancing asset time and safety significantly.
Automotive
Automotive manufacturers are applying condition-based maintenance to improve maintenance planning both in production plants and for after-sales vehicles. This approach minimizes planning delays and enhances vehicle service, thereby improving customer experience and safety.
Logistics
For logistics companies, PdM integrated with IoT allows for better management of distributed assets, such as transport vehicles and distribution centers. This facilitates timely maintenance planning, reducing material handling system downtimes and improving overall operational efficiency.
Smart City Infrastructure
City authorities are leveraging PdM to model and predict potential health issues of city infrastructures, like sewer systems, water tanks, and energy grids. This predictive approach aids in planning maintenance activities more effectively, improving the quality of life for residents by ensuring infrastructure reliability and safety.
Real-World Examples
- Frito-Lay: Implemented a robust PdM program using vibration analysis and infrared monitoring to predict equipment failures. This program is crucial for maintaining the production of over 150 million pounds of products annually.
- Noranda Alumina Refinery: Achieved a 60% reduction in bearing changes, saving approximately $900,000 by employing ultrasonic predictive maintenance.
- San Diego Gas & Electric: Used machine learning algorithms to predict failures of underground power distribution assets like T-splices, enhancing operational efficiency and reducing the risk of wildfires.
- Singapore Rail Operator SMRT Trains Ltd.: Achieved one million mean kilometers between failures across all lines by implementing a Predictive Decision Support System, significantly improving safety, reliability, and convenience for commuters.
- An Iron Ore Mine in Australia: Avoided substantial costs by utilizing AI-driven PdM to detect anomalies in a crusher’s drive and hydraulic system, preventing potential catastrophic failures.
Key Companies Analysis
- IBM offers predictive maintenance through its Maximo Application Suite, utilizing operational data and analytics for asset health insights to optimize asset health and predict failures. It features condition-based predictive maintenance, incorporating IoT data for enhanced reliability and maintenance management. Maximo Health and Maximo Predict are integral parts, enabling better decision-making and maintenance planning to improve asset availability and reduce failures.
- General Electric (GE) specializes in predictive maintenance technologies aimed at detecting, diagnosing, and predicting failures in machine components before they become critical. GE’s approach focuses on preventing downtime and enabling efficient, evidence-based maintenance planning. Their technologies are utilized across various sectors including aerospace, power generation, transportation, and healthcare, supporting over a hundred thousand assets. GE Research pursues advancements in early warning systems and prognostics to enhance predictive maintenance capabilities.
- SAP SE is a global leader in enterprise application software, helping businesses of all sizes and industries to operate more efficiently. With over 105,000 employees from more than 157 countries, SAP’s innovative solutions support businesses by offering end-to-end applications and services. This enables customers to run profitably, adapt continuously, and make a significant impact worldwide. In 2022, SAP reported a total revenue of €29.52 billion and has a cloud user base of over 280 million subscribers. SAP is recognized for its contributions to sustainability, holding the position of the #1 software company in the Dow Jones Sustainability Index for 16 years.
- Microsoft Corporation has embarked on a strategic collaboration with Procter & Gamble to transform digital manufacturing. Leveraging Microsoft Cloud, this partnership aims to enhance P&G’s digital manufacturing platform across over 100 global sites, integrating AI, machine learning, and the Industrial Internet of Things (IIoT). This initiative is set to expedite product delivery, elevate customer satisfaction, and amplify productivity to curtail costs, marking a significant advancement in the utilization of digital technologies in manufacturing.
- PTC Inc. specializes in predictive maintenance, utilizing real-time data to track the health, status, and performance of assets. This approach aims to minimize unexpected breakdowns, allowing manufacturers to schedule maintenance around their production schedules. PTC leverages technologies like the Industrial Internet of Things (IIoT) to continuously analyze equipment conditions, enabling early detection of potential failures. Predictive maintenance at PTC includes techniques such as vibration analysis, infrared analysis, and sonic acoustical analysis, contributing to decreased downtime, increased worker productivity, and reduced field service costs.
- Schneider Electric SE is a global leader in digital automation and energy management. It provides innovative solutions for managing energy and automation in homes, buildings, data centers, infrastructure, and industries. The company, a Fortune Global 500 entity, is publicly traded on the Euronext Exchange and is part of the Euro Stoxx 50 stock market index. With a history dating back to 1836, Schneider Electric has evolved through strategic acquisitions to focus on electricity and energy management. It’s committed to sustainability and innovation, with significant investments in research and development to integrate emerging technologies like IoT, AI, and big data into its solutions.
- Siemens AG is actively advancing in the realm of predictive maintenance, which aims to minimize unplanned downtime and optimize maintenance planning through condition-based maintenance solutions. These efforts are especially crucial for industries where downtime can lead to significant financial losses. Siemens offers a comprehensive suite of predictive maintenance services, leveraging analytics and artificial intelligence to foresee potential faults and ensure reliability redefined.
Conclusion
The predictive maintenance market’s future is bright, fueled by a relentless pursuit of efficiency, cost reduction, and operational excellence across diverse industries. As the market evolves, it will likely witness further technological innovations and increased adoption, making predictive maintenance an indispensable tool in the modern industrial landscape.
Discuss your needs with our analyst
Please share your requirements with more details so our analyst can check if they can solve your problem(s)
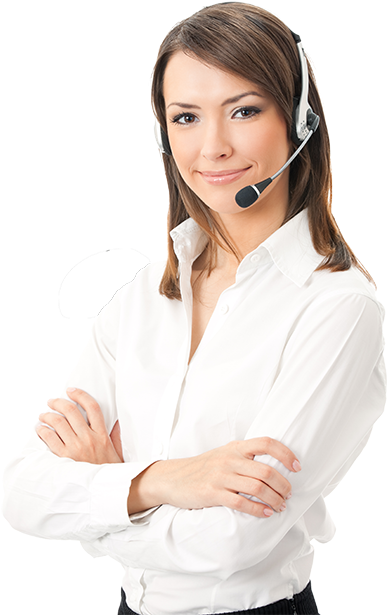