Table of Contents
- Introduction
- Editor’s Choice
- Predictive Analytics Market Overview
- Top Use Cases of AI & ML
- Data Analysis Processes Improved with Big Data and AI Solutions
- Tasks That Can Be Easily Automated by Machines
- Predictive Analytics in Marketing
- Predictive AI Integration Statistics
- Predictive AI Applications Statistics
- Challenges in the Adoption of Predictive AI Statistics
- Predictive AI Trends Statistics
- Recent Developments
- Conclusion
- FAQs
Introduction
Predictive AI Statistics: Predictive AI is a method rooted in data analysis that utilizes past and current data to predict upcoming developments and patterns with precision.
It depends on sophisticated statistical techniques and mathematical algorithms to recognize trends, make forecasts, and enhance decision-making.
This approach has been broadly employed across different sectors. Allowing companies to improve efficiency, cut expenses, and acquire valuable insights for making well-informed decisions.
Nevertheless, it is important to cautiously handle issues concerning data accuracy. The comprehensibility of models, and data protection to ensure successful utilization.
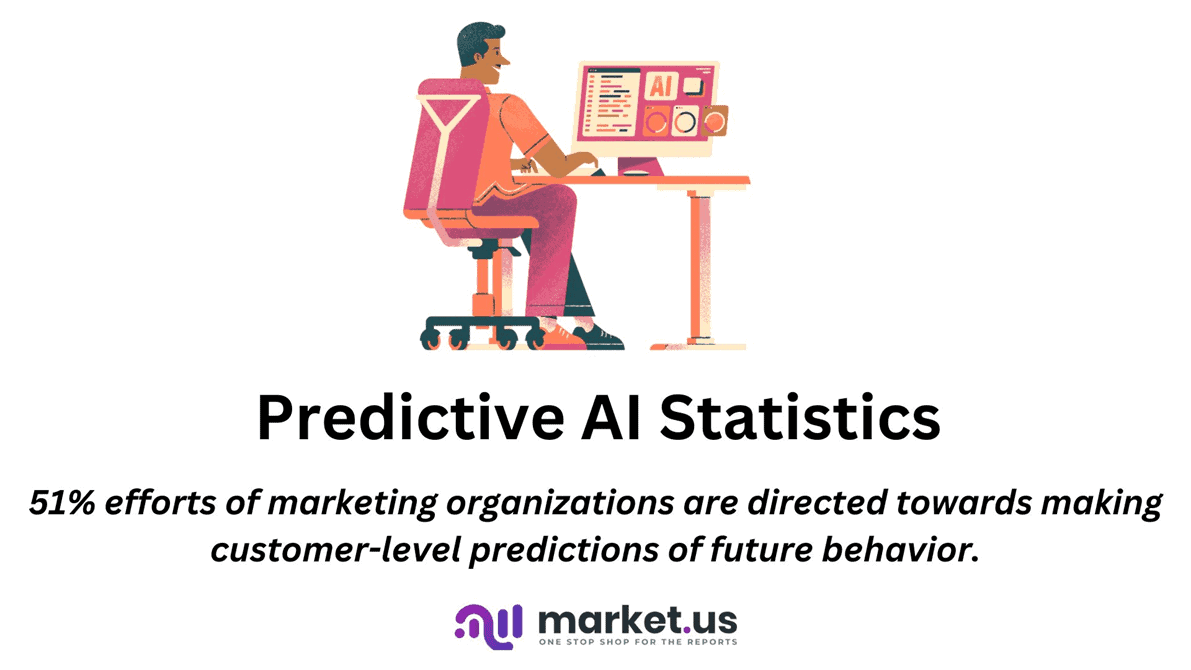
Editor’s Choice
- The Predictive AI Market size is expected to be worth around USD 108 Billion by 2033, from USD 14.9 Billion in 2023
- The global predictive analytics market is poised for substantial expansion. With projections indicating that by 2028, its revenue is expected to soar to USD 41.52 billion.
- Big Data and Artificial Intelligence (AI) solutions technologies have enabled organizations to tackle the analysis of large volumes of data with great efficiency, garnering 57% of attention.
- Among the tasks that can be easily automated, predictable physical work tops the list, with a substantial 78% focus.
- 51% of efforts made by marketing organizations are directed towards making customer-level predictions of future behavior, enabling tailored marketing approaches.
- Despite the widespread acknowledgment of consumer data’s importance in areas like predicting future purchases and customer retention. More than 4 out of 5 marketing executives face challenges when it comes to making data-driven decisions.
- PepsiCo has successfully shifted approximately 4,300 workdays annually from routine tasks to more strategic endeavors through the use of predictive AI for managing inventory.
- Challenges hindering the adoption of predictive analytics within marketing teams include the significant expenses associated with manual data science. Which is a concern for 40% of respondents.
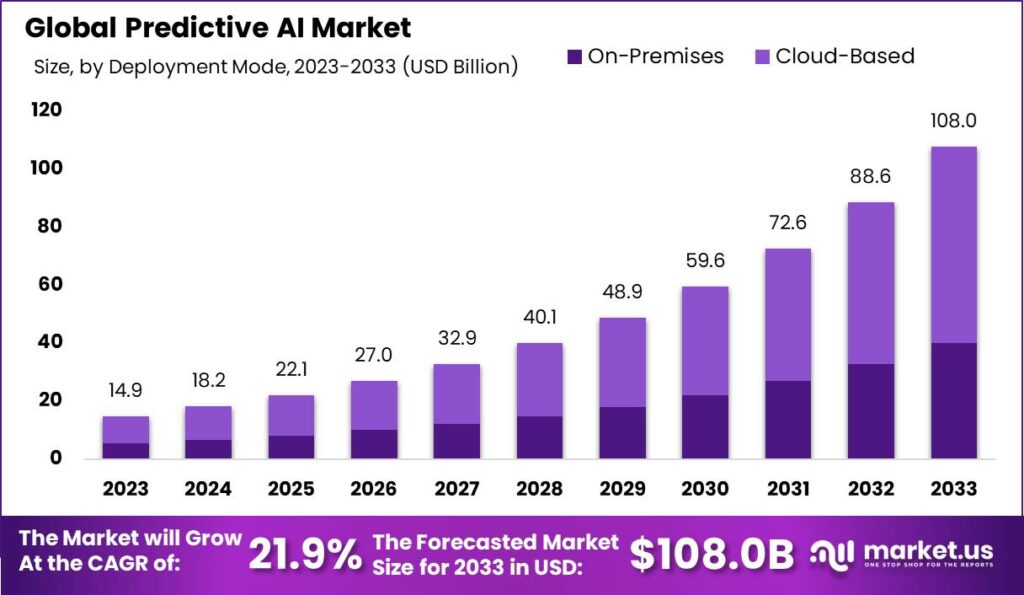
Predictive Analytics Market Overview
Global Predictive Analytics Market Size
- The global predictive analytics market has witnessed remarkable growth in recent years. In 2020, it recorded a revenue of USD 5.29 billion.
- However, the market is poised for substantial expansion, with projections indicating that by 2028, its revenue is expected to soar to USD 41.52 billion.
- This significant growth can be attributed to the increasing adoption of predictive analytics across various industries.
- Organizations are recognizing the value of leveraging historical and real-time data to make informed decisions, enhance operational efficiency, and gain a competitive edge.
- As a result, the predictive analytics market is becoming increasingly integral to modern business strategies, and the forecasted revenue figures reflect the growing importance of data-driven insights in today’s competitive landscape.
(Source: Statista)
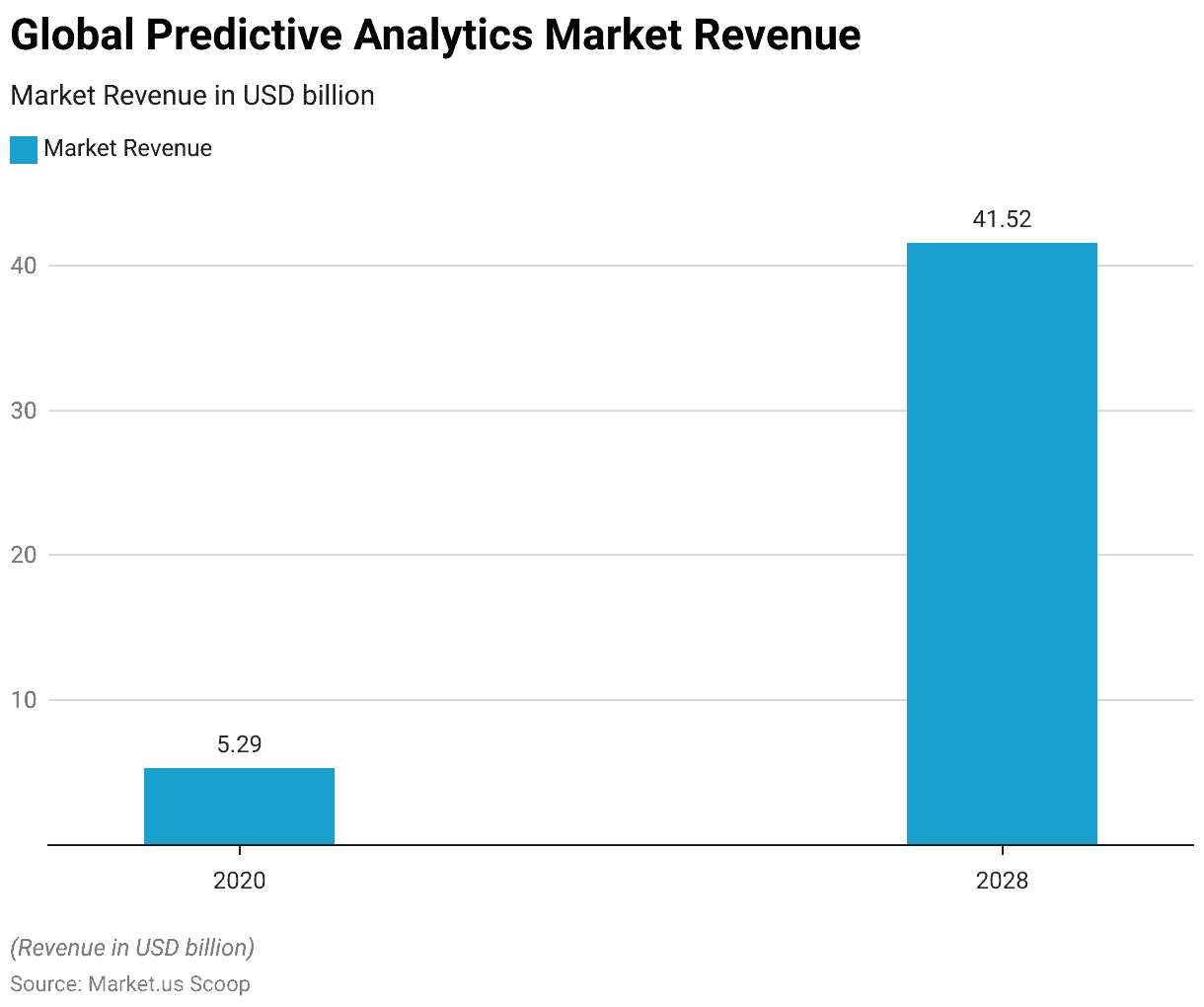
Top Use Cases of AI & ML
- In both 2020 and 2021, the top use cases of Artificial Intelligence (AI) and Machine Learning (ML) technologies have centered around enhancing customer-centric aspects of businesses.
- In 2020, 37% of the focus was on generating customer insights and intelligence. While 34% aimed at improving the overall customer experience.
- Retaining customers, interacting with them effectively, and implementing recommender systems also featured prominently.
- Fast forward to 2021, and the trend continued to intensify, with 50% of the attention directed toward generating customer insights and a significant leap to 57% focused on improving the customer experience.
- AI and ML played crucial roles in detecting fraud, with a 27% focus in 2020. This will increase to 46% in 2021, reflecting the growing need for security measures.
- While reducing customer churn and acquiring new customers remained important, the year 2021 saw a notable emphasis on increasing customer loyalty and fostering long-term engagement.
- The evolving landscape of AI and ML applications underscored their pivotal role in strengthening brand awareness and shaping diverse aspects of customer interactions. With only 1% representing other use cases.
(Source: Statista)
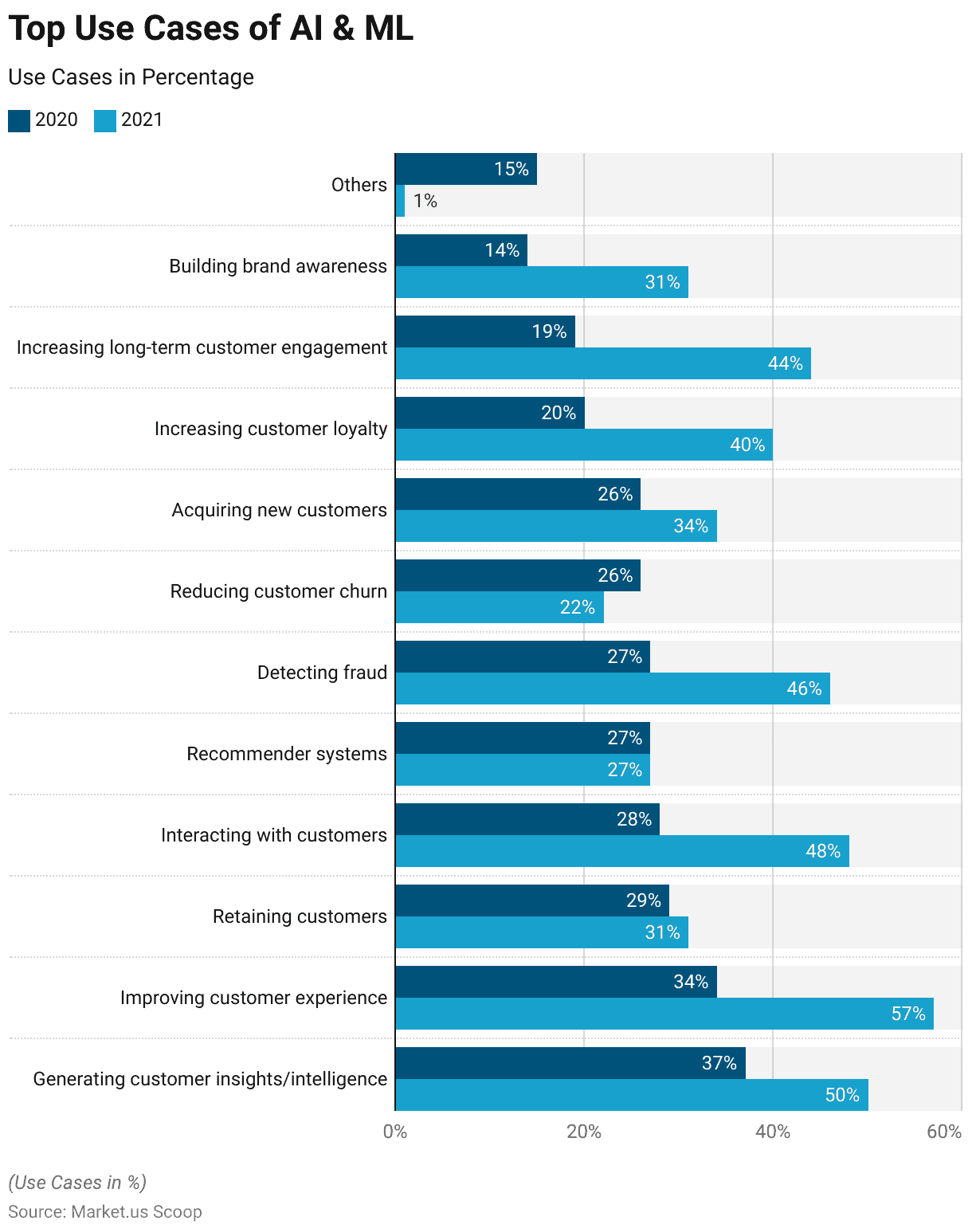
Data Analysis Processes Improved with Big Data and AI Solutions
- The integration of Big Data and Artificial Intelligence (AI) solutions has significantly improved various aspects of data analysis processes.
- In particular, these technologies have enabled organizations to tackle the analysis of large volumes of data with great efficiency, garnering 57% of attention.
- Moreover, they have expanded the horizons of data analysis. Providing new possibilities that were previously inaccessible, which garnered 55% of focus.
- Building predictive models emerged as another vital area, with 51% of organizations leveraging AI and Big Data for this purpose.
- The ability to analyze information from diverse data sources. Even those with polystructured data have become more accessible, ranking at 50%.
- Additionally, these solutions have expedited the delivery of data for analysis (46%) and accelerated decision-making processes (31%).
- Monitoring and analyzing streaming data (27%) have also gained prominence.
- Organizations have started to see improved cost-benefit ratios in their analytical environments (23%) and are exploring automation of decision-making (19%).
- While a minority report no pressing challenges (6%), only 1% cited other use cases. Underscoring the transformative impact of AI and Big Data on data analysis processes.
(Source: BI-Survey)
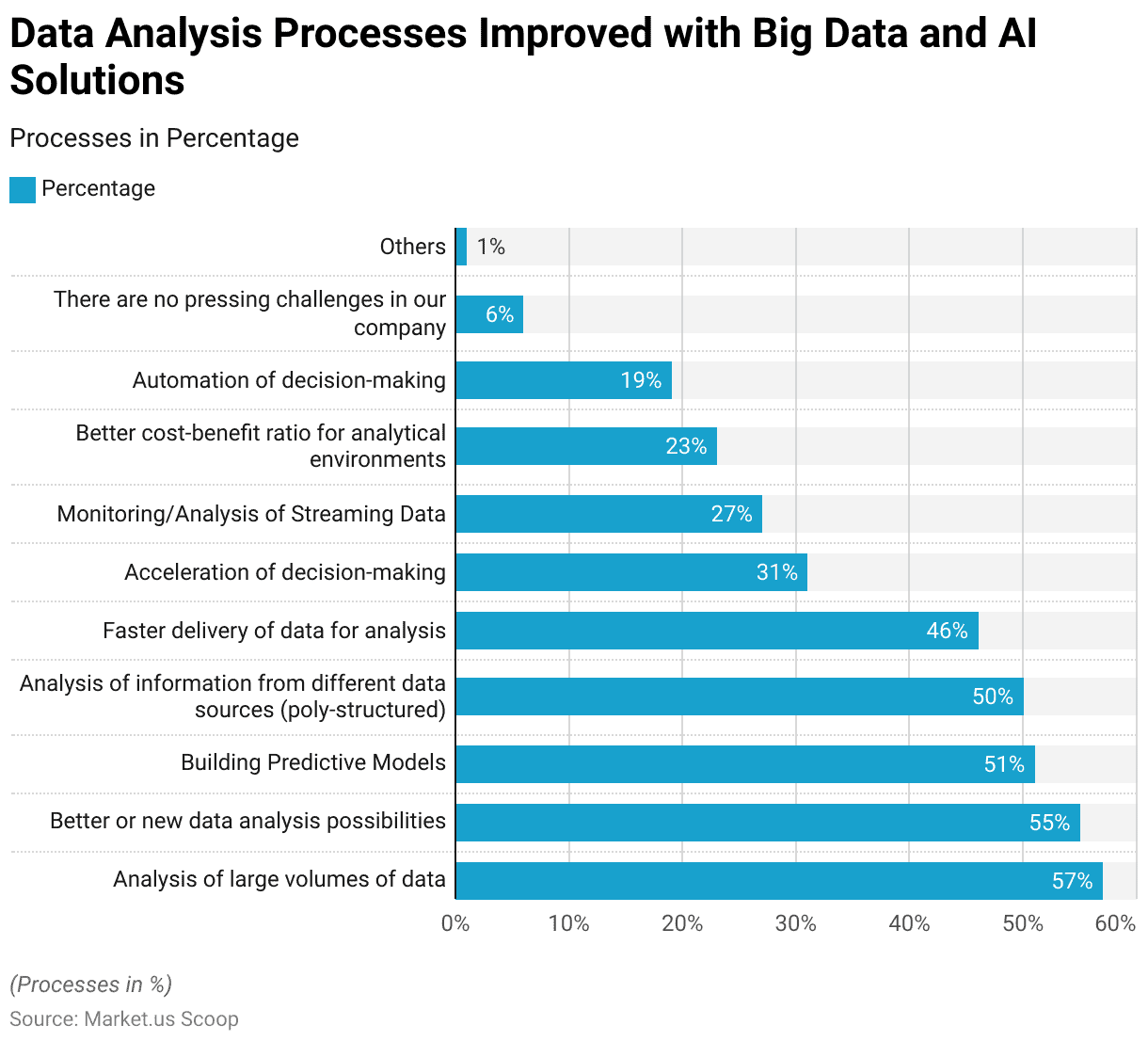
Tasks That Can Be Easily Automated by Machines
- Machines have demonstrated remarkable capabilities in automating various tasks, making significant contributions across diverse fields.
- Among the tasks that can be easily automated, predictable physical work tops the list, with a substantial 78% focus.
- Data processing closely follows, with 69% recognizing the efficiency of machines in handling this aspect of work.
- Data collection, another data-related task, also stands out as highly amenable to automation, at 64%.
- In contrast, unpredictable physical work is seen as less suitable for automation, garnering 25% of attention.
- Stakeholder interactions and applying expertise, tasks often requiring human judgment, scored at 20% and 18%, respectively.
- Lastly, the management of others appears to be less conducive to automation, with only 9% acknowledging its automation potential.
- These insights reflect the evolving landscape of automation, where machines excel in tasks characterized by predictability and data-related activities.
(Source: McKinsey)
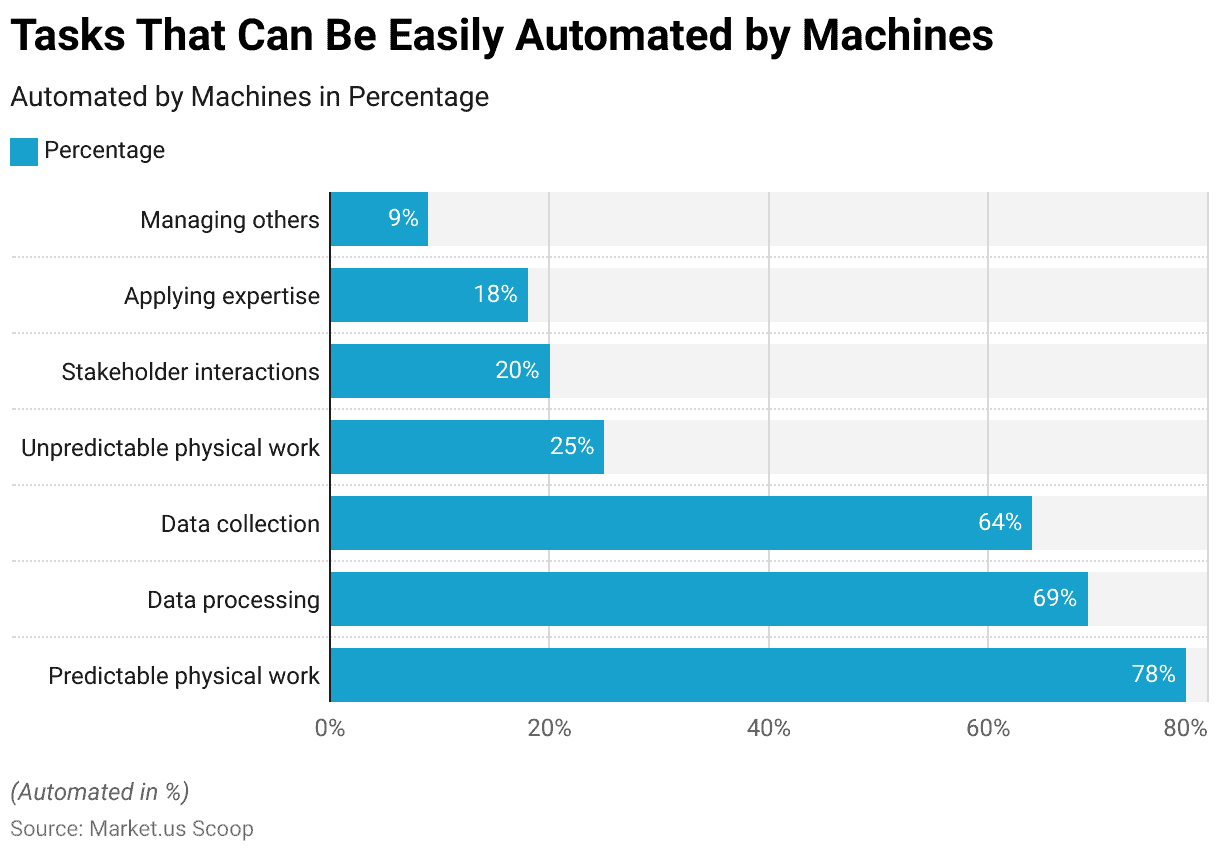
Predictive Analytics in Marketing
- Marketing organizations have harnessed the power of predictive analytics in a variety of ways to enhance their strategies and customer engagement.
- A significant 51% of their efforts are directed towards making customer-level predictions of future behavior, enabling tailored marketing approaches.
- Close behind, at 50%, is the forecasting of customer trends, allowing organizations to stay ahead of market shifts.
- Forecasting purchasing behavior for priority segments, a crucial aspect of marketing, garners 46% of the attention, while a nearly equal 44% focus on forecasting respondent-level purchasing behavior and customer segmentation.
- Moreover, predictive analytics plays a vital role in modeling to uncover valuable insights, with 40% of marketing organizations relying on this approach to inform their decision-making and refine their marketing strategies.
- These practices underline the integral role of predictive analytics in modern marketing efforts, enabling organizations to better understand and engage with their target audience.
(Source: Pecan’s State of Predictive Analytics in Marketing Report)
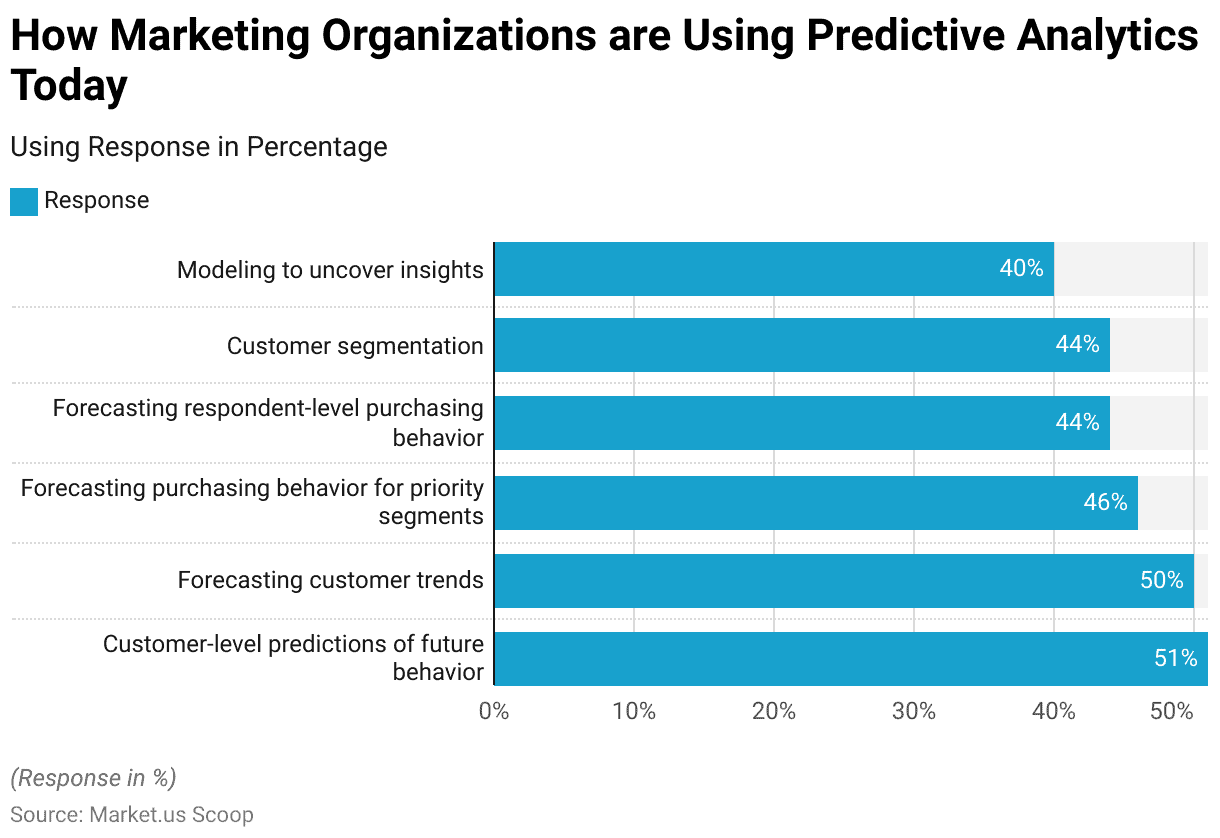
Predictive AI Integration Statistics
- Despite the widespread acknowledgment of consumer data’s importance in areas like predicting future purchases and customer retention, more than 4 out of 5 marketing executives face challenges when it comes to making data-driven decisions.
- These difficulties are reported by 84% of respondents, who feel that their ability to predict consumer behavior often lacks accuracy and resembles guesswork.
- Furthermore, a substantial 95% of companies now incorporate AI-powered predictive analytics into their marketing strategies, with 44% indicating full integration.
- Interestingly, among those companies that have fully integrated AI predictive analytics, 90% encounter hurdles in making daily data-informed decisions.
- These challenges include data not being updated promptly (38%), time-consuming model development processes (35%), overwhelmed data scientists (42%), a disconnect between model builders and marketing objectives (40%), and the utilization of incorrect or incomplete data for model creation (37%).
- These findings highlight the complexities marketing executives face despite the widespread adoption of data-driven approaches.
(Source: Venturebeat)
Predictive AI Applications Statistics
- EY has achieved significant time savings equivalent to 250,000 hours by implementing smart document automation.
- PepsiCo has successfully shifted approximately 4,300 workdays annually from routine tasks to more strategic endeavors through the use of predictive AI for managing inventory.
- Amadeus utilizes predictive AI to process a staggering 100,000 transactions per second, thereby reducing data fragmentation within the travel industry.
- This has led to improved experiences for travelers and industry clients.
- Johnson & Johnson expedited their COVID-19 vaccine development by employing predictive AI to identify optimal clinical trial locations, enabling an earlier start to vaccine trials.
- Coveo leverages AI-driven cognitive search to provide highly relevant results and enhance the searchability of product catalogs, thereby improving the B2B purchasing process and enhancing the overall shopping experience.
(Source: Forbes, Unite.ai, Coveo)
Challenges in the Adoption of Predictive AI Statistics
- Challenges hindering the adoption of predictive analytics within marketing teams include the significant expenses associated with manual data science, which is a concern for 40% of respondents.
- Another obstacle, shared by the same percentage, is the presence of disorganized or unstructured customer data.
- Additionally, 39% cited limited technical expertise within their marketing and analytics teams, while an equal percentage pointed to leadership’s skepticism regarding the value of predictive analytics.
- A lack of internal data science resources was noted by 38% of respondents, and 35% faced issues related to insufficient or outdated data.
- Lastly, 31% struggled with data isolation, where information was compartmentalized and not easily accessible across the organization.
(Source: Pecan)
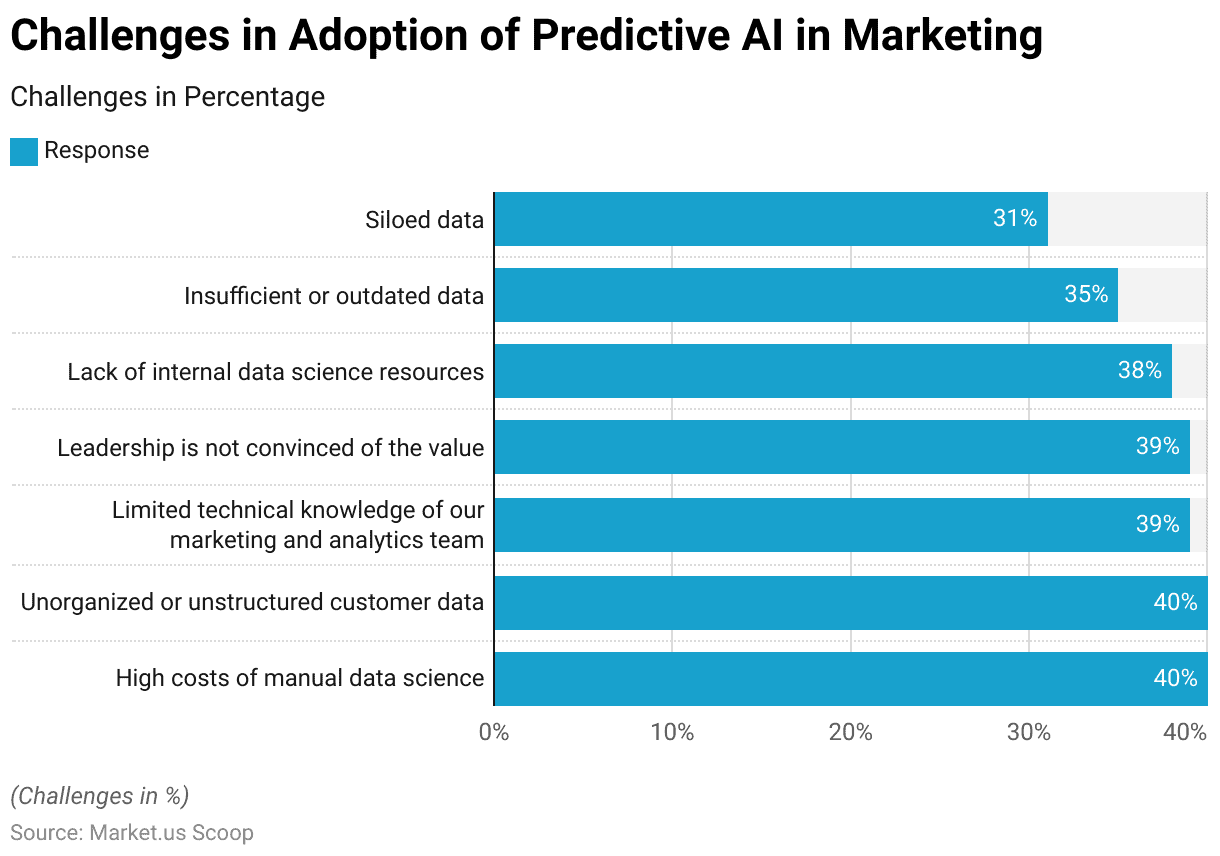
Predictive AI Trends Statistics
- In the realm of hyper-personalization, a recent survey by Ascend2 reveals that open-ended chatbots are the least favored among the provided AI-powered tools, with only 18% of respondents opting for them.
- On the contrary, predictive analytics emerges as the preferred choice, with a significant 56% of respondents selecting it, surpassing conversational tactics by more than threefold.
- Following closely behind are user experience (UX) applications, chosen by 46% of respondents as the second most effective option.
- Predictive analytics has gained significant attention recently, with findings from Harvard Business Review indicating that 61% of enterprise business leaders presently view it as “highly significant.”
- Furthermore, an even more substantial 74% anticipate its growing importance within the next two years.
- Notably, as far back as 2016, predictive analytics stood out as the top priority in the realm of data analytics, particularly for those engaged in B2B activities.
- According to the Ascend2 study, implementing this technology remains a complex task, with nearly half of respondents (48%) considering it one of the most challenging applications to incorporate into a hyper-personalization strategy.
- User experience (UX) applications also present difficulties, as over 4 in 10 respondents (41%) find them challenging to implement.
- This aligns with another research study supported by Adobe, which revealed that only half of companies felt confident in their ability to design effective user experiences.
(Source: Ascend2, Adobe, Harvard Business Review)
Recent Developments
Acquisitions and Mergers:
- IBM acquires Databand.ai: In mid-2023, IBM acquired Databand.ai, a data observability startup, for $75 million. This acquisition aims to enhance IBM’s predictive AI capabilities by integrating Databand.ai’s technology to improve data quality and predictive analytics across its AI platforms.
- Microsoft acquires Nuance Communications: In 2023, Microsoft completed its acquisition of Nuance Communications, a leader in AI-powered speech recognition and predictive analytics, for $19.7 billion. The acquisition strengthens Microsoft’s healthcare AI offerings, enabling more advanced predictive analytics in patient diagnostics.
New Product Launches:
- Google Cloud introduces Vertex AI Forecast: In early 2024, Google Cloud launched Vertex AI Forecast, a tool designed for predictive analytics across multiple industries. Vertex AI Forecast enables businesses to improve demand forecasting by 20% and optimize supply chain operations using AI-powered insights.
- Salesforce launches Einstein AI for predictive marketing: In March 2024, Salesforce introduced a new version of Einstein AI, focusing on predictive analytics for marketing. The tool helps businesses forecast customer behavior and optimize marketing campaigns, resulting in 25% more effective targeted ads.
Funding:
- C3.ai secures $100 million for AI platform expansion: In 2023, C3.ai, a leader in enterprise AI, raised $100 million to expand its predictive AI platform, focusing on industries such as energy, manufacturing, and healthcare. This funding will accelerate the development of AI solutions for predictive maintenance and operational efficiency.
- H2O.ai raises $72.5 million for predictive AI innovation: In late 2023, H2O.ai, an AI cloud company, raised $72.5 million in Series D funding to enhance its H2O Driverless AI platform. This platform uses machine learning algorithms to deliver highly accurate predictive models for various industries.
Technological Advancements:
- AI-driven predictive healthcare solutions: By 2025, 50% of healthcare providers are expected to integrate AI-powered predictive analytics to improve patient care and diagnostics, helping predict disease outbreaks and optimize treatment plans.
- Predictive AI in supply chain management: Predictive AI is transforming supply chains by forecasting demand, managing inventory, and mitigating risks. By 2026, 45% of global supply chains will use AI for predictive analytics to streamline operations and reduce costs.
Conclusion
Predictive AI Statistics – In summary, predictive AI offers significant potential across diverse industries and applications. It empowers organizations to base their decisions on data, predict future trends, and enhance operational efficiency.
However, as evident from the information presented, several obstacles and intricacies still hinder the complete utilization of predictive AI.
Despite its increasing importance, certain challenges like implementation difficulties and the necessity for technical proficiency persist. Nevertheless, the demand for predictive analytics remains strong and is projected to grow further.
As businesses address these obstacles, they should also actively explore the prospects that predictive AI brings to stay competitive and provide better customer service.
FAQs
Predictive AI, or predictive artificial intelligence, is a technology that uses algorithms and machine learning techniques to analyze historical and real-time data to make predictions about future events or outcomes with a high level of accuracy.
Predictive AI is used in various fields, including marketing (customer behavior prediction), finance (risk assessment and fraud detection), healthcare (disease prognosis), and manufacturing (predictive maintenance), among others.
Predictive AI works by training models on historical data, identifying patterns and correlations in the data, and then applying these patterns to new data to make predictions. These models continuously learn and adapt to new information.
Predictive AI helps organizations make informed decisions, optimize processes, reduce costs, and improve customer experiences. It can also identify hidden insights in data that may not be apparent through traditional analysis.
Challenges include the high cost of implementation, the need for high-quality and up-to-date data, a shortage of technical expertise, and the complexity of integrating predictive AI into existing systems.
Discuss your needs with our analyst
Please share your requirements with more details so our analyst can check if they can solve your problem(s)
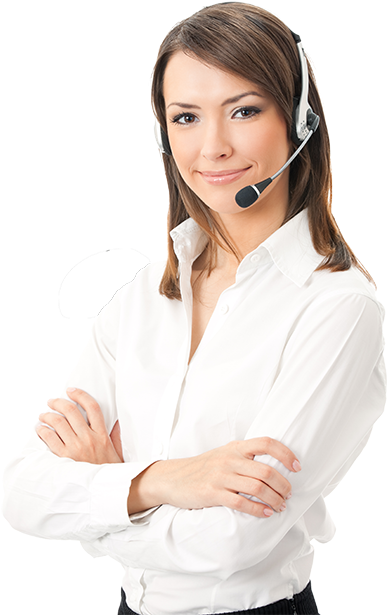